こんにちは.
本記事では文脈を考慮した単語埋め込み表現(Word Embedding)である ELMo (Embeddings from Language Models)を,TensorFlow Hubを用いて利用する方法について解説します.
実装はGitHubの**こちら**
Word Embeddingとは一体...,という方はこちらがわかりやすそうです.
ELMo
エルモと聞くとまずあの赤いキャラクターを彷彿とさせますが,こっちのエルモはWord2Vecで有名なWord Embeddingの手法の1つです.
さて,ここで以下の3文を見てみましょう.
- You should turn right at the next corner.
(あなたは次の角を右に曲がるべきです) - Your opinion is more or less right.
(君の意見はだいたい正しいよ) - I have a right to ask a question !
(僕には質問をする権利がある!)
もはや見るだけで何を言いたいか伝わりそうですが,自然言語にはいわゆる多義性というものが存在します.つまり,まったく同じ単語でも文脈によっては異なる意味を持つということですね.
ELMoは,双方向LSTM(Bidirectional LSTM)を用いて大量のコーパスを学習することで,同じ単語でも文脈によって異なる埋め込み表現を獲得することができる手法の一つです.今年(2018年)にarXivに投稿され,加えてTensorFlow Hubに学習済みモデルが公開されたことから話題になりました.
ではTensorFlow Hubとはなんなのか,簡単に説明していきます.
TensorFlow Hub
TensorFlow Hubとは学習済みの機械学習モデルを利用できるライブラリで,転移学習をするときなどにとても便利です.今回のELMo等の自然言語モデルは学習において常人のリソースではとても扱えない膨大なコーパスを利用することがあるので,エンドユーザにとっては嬉しい機能ですね.
使い方は非常に簡単で,学習済みモデルが置かれているURLを指定するだけですぐに利用可能です.ただし,初回実行時はモデルをサーバからダウンロードしてくるため数分待つことになるので,気長に待ちましょう.(2回目からはローカルに保存されたモデルが自動的に利用されます!)
パッケージはpip
コマンドでインストール可能です.
pip install tensorflow-hub
例えば,Feed-Forward型NNを用いたWord EmbeddingモデルのNNLMを利用したい場合は,
nnlm = hub.Module("https://tfhub.dev/google/nnlm-en-dim128/1")
たったこれだけでモデルが使用可能になります.それぞれのモデルに関する情報は,TensorFlow公式に載っていて,NNLMならば以下のようにModule URLやOverviewが公開されています.ありがたい.
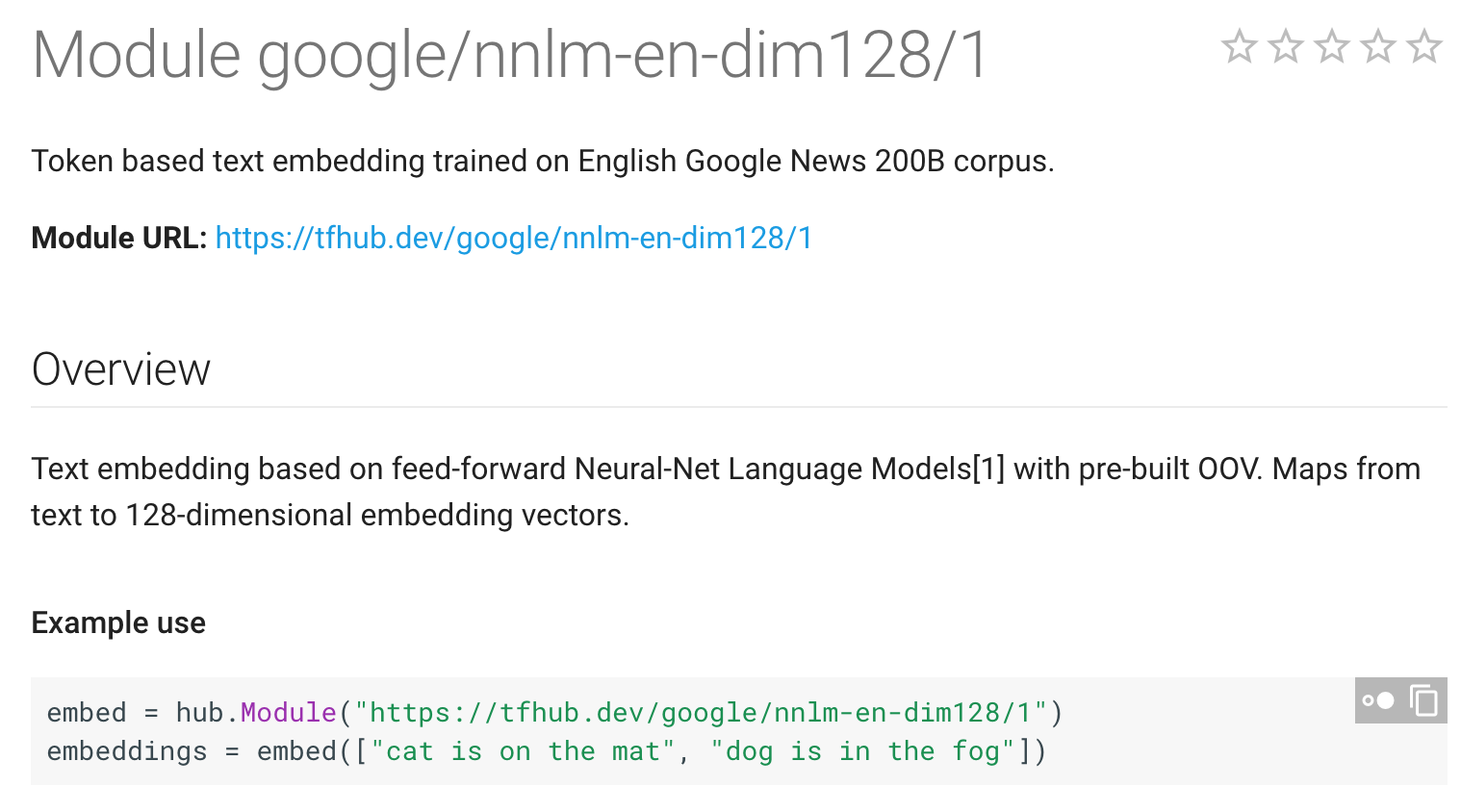
TensorFlow Hubの使い方がわかったところで,本題のELMoを使った実装をしていきましょう.
実装
文を入力した際に,任意のアルゴリズムで対応する文ベクトルを得る機能を実装します.今回は実験でNNLMも利用するので,ELMoとNNLMによるEmbedding機能を作っていきましょう.またこれらはモジュールとして再利用可能なように,embeddings.py上に実装していきます.
はじめに,ELMoとNNLMのModule URLを定義しておきます.
ELMO = "https://tfhub.dev/google/elmo/2"
NNLM = "https://tfhub.dev/google/nnlm-en-dim128/1"
次に,モデル名をキーとして引数に与えられた文に対応するベクトルを返却する関数embed
を作成します.
import tensorflow_hub as hub
import tensorflow as tf
def embed(model_name, sentences):
if model_name == "elmo":
elmo = hub.Module(ELMO, trainable=True)
executable = elmo(
sentences,
signature="default",
as_dict=True)["elmo"]
elif model_name == "nnlm":
nnlm = hub.Module(NNLM)
executable = nnlm(sentences)
else:
raise NotImplementedError
return execute(executable)
def execute(tensor):
with tf.Session() as sess:
sess.run(tf.global_variables_initializer())
sess.run(tf.tables_initializer())
return sess.run(tensor)
この時,hub.Module
に文を投げただけでは結果が帰ってこないことに注意しましょう.帰ってくるのはあくまでTensorオブジェクトですので,Sessionに投げて実行する必要があります.上記コードでは,execute
関数として実装しています.
また,sess.run
する前に,
tf.global_variables_initializer()
tf.tables_initializer()
の2つで初期化することを忘れないようにしましょう.Hub上のサンプルコードに書いていないため,ハマりがちです.忘れると
Not found: Container localhost does not exist. (Could not find resource: localhost/module/bilm/char_embed)
みたいなのを含んだ大量のエラーが出てつらいです.
最後に,ELMoとNNLMの文ベクトルを同時に取得できるように,先ほど作成した関数を利用したget_embeddings_elmo_nnlm
関数を作っておきます.これでこの関数に文のリストを与えてあげるだけで,対応する文ベクトルが取得できますね.
def word_to_sentence(embeddings):
return embeddings.sum(axis=1)
def get_embeddings_elmo_nnlm(sentences):
return word_to_sentence(embed("elmo", sentences)), embed("nnlm", sentences)
NNLMはデフォルトで文ベクトルを出力しますが,ELMoはあくまで単語ベクトルですので,関数word_to_sentence
で単語ベクトルを足し合わせています.
そんな単純に足し合わせて良いのかという話もありますが,最近こそSentence Embeddingの研究が盛んになっているものの,単純に単語ベクトルの線形結合をとるだけである程度タスクに対して有効だったりします.ですから,今回はNNLMとの比較ということでsum
(荷重1の線形結合と同じ)をとります.計算するのはcos類似度ですから,単語長で全体を正規化することは今回は考えません.
ここまででできたembeddings.py
をインポートした,comparison_test.py
を作成し,以下のコードを記述します.
import embeddings
import numpy as np
import argparse
def cos_sim(a, b):
return np.inner(a, b) / (np.linalg.norm(a) * (np.linalg.norm(b)))
def get_parser():
parser = argparse.ArgumentParser(
prog='ELMO vs NNLM',
usage='python comparison_test.py [sentence1] [sentence2]',
description='This module demonstrates comparison of ELMo and NNLM',
add_help=True
)
parser.add_argument("sentences", nargs=2, help="The sentences to compare cosine similarity")
return parser
if __name__ == "__main__":
parser = get_parser().parse_args()
sentence1, sentence2 = parser.sentences
# Get embeddings corresponding to each sentences
results_elmo, results_nnlm = embeddings.get_embeddings_elmo_nnlm([sentence1, sentence2])
print("[Cosine Similarity]")
print("\"{}\" vs \"{}\"".format(sentence1, sentence2))
print("ELMo:", cos_sim(results_elmo[0], results_elmo[1]))
print("NNLM:", cos_sim(results_nnlm[0], results_nnlm[1]))
実験
簡単な実験として,同じ単語を使った文同士のコサイン類似度が1.0にならないことを確認してみましょう.
- people read the book
- the book people read
使っている単語は全く同じですが,意味合い的には変わってきます.以下のように実行することで,結果が確認できます.
python comparison_test.py "people read the book" "the book people read"
[Cosine Similarity]
"people read the book" vs "the book people read"
ELMo: 0.83875865
NNLM: 1.0
文脈を考慮しないNNLMではコサイン類似度が1.0,すなわち2文のベクトルが全く同じ方向を向いていることがわかります.対してELMoでは0.84程度と,語順によって異なるベクトルを得られていますね.
ただ,この文は冒頭で例をあげたような明確な多義性を持つわけではないので,正しく文脈を考慮した埋め込み表現になったのかを検証できたわけではありませんが,手法の差異を確認することはできたでしょう.(何か面白い例があれば教えてください)
まとめ
今回は,文脈を考慮した単語埋め込み表現,ELMoをTensorFlow Hubを用いて利用してみました.実験で試験的に用いた文は4語と短いものでしたが,時系列モデルを採用しているためより長い文に対してより有用な効果が得られると思います.ではまた.