1. ONNXとは
Tensorflow, PyTorch, MXNet, scikit-learnなど、いろんなライブラリで作った機械学習モデルをPython以外の言語で動作させようというライブラリです。C++, C#, Java, Node.js, Ruby, Pythonなどの言語向けのビルドが作られています。ハードウェアもCPU, Nvidia GPUのほかAMD GPUやNPU、FPGAなどにも対応を広げているので、デプロイ任せとけ的な位置付けになるようです。
いろんな言語やハードウェアで動かせるというのも大きなメリットですが、従来pickle書き出し以外にモデルの保存方法がなかったscikit-learnもonnx形式に変換しておけばONNX Runtimeで推論できるようになっていますので、ある日scikit-learnモデルのメモリ構造が変わって読めなくなるんじゃないかと怯えながら使うというのを回避できるのも大きなメリットだと思います。
2. インストール
公式ページでOSや言語、プロセッサを選択すればインストール方法を教えてくれます
https://onnxruntime.ai/

Pythonの場合は以下に誘導されると思います
https://onnxruntime.ai/docs/install/
で、結論としてはpipで入るのだと判明します
pip install onnxruntime
pip install onnxruntime-gpu
GPUを使った方が普通に速いのでCUDA載ってるPCならGPU版を入れましょう。ONNX runtimeバージョンごとに対応しているCUDAが変わるので、現環境に入っているバージョンでインストールします。
https://onnxruntime.ai/docs/execution-providers/CUDA-ExecutionProvider.html#requirements
3. ONNXモデルを使う
使い方のデモファイル通りにやってみます
https://github.com/onnx/onnx-docker/blob/master/onnx-ecosystem/inference_demos/simple_onnxruntime_inference.ipynb
3-1. モデルの準備
onnxruntime.InferenceSession(モデルのPATH)とすると指定したONNXモデルを使って推論するためのsessionを準備してくれます。ここではパッケージに付属しているサンプルモデルを使って推論をやってみます。
import onnx
import onnxruntime
import numpy as np
from onnxruntime.datasets import get_example
example_model = get_example("sigmoid.onnx")
sess = onnxruntime.InferenceSession(example_model,
providers=['CUDAExecutionProvider', 'CPUExecutionProvider'])
onnxruntime-gpuをインストールした場合はどのプロセッサのproviderを使うか明確に指定しないといけないので、ここではCUDAまたはCPUを使うものとして指定しています。CPU版をインストールしている場合は省略可能です。
3-2. モデルを確認
以下のようにするとモデルの内容をざっと確認することが出来ます
model = onnx.load(example_model)
onnx.checker.check_model(model)
print(onnx.helper.printable_graph(model.graph))
入力段が(3, 4, 5)次元でシグモイドをかけて出力するというシンプルなモデルのようです
3-3. モデルの入出力情報を取得
sess.get_input()、sess.get_outputs()から入出力段のname, shape, typeが取得できます
input_name = sess.get_inputs()[0].name
print("Input name :", input_name)
input_shape = sess.get_inputs()[0].shape
print("Input shape :", input_shape)
input_type = sess.get_inputs()[0].type
print("Input type :", input_type)
output_name = sess.get_outputs()[0].name
print("Output name :", output_name)
output_shape = sess.get_outputs()[0].shape
print("Output shape :", output_shape)
output_type = sess.get_outputs()[0].type
print("Output type :", output_type)
3-4. 推論する
適当なxを使って推論させてみます
x = np.random.random(input_shape) * 6 - 3
x = x.astype(np.float32)
result = sess.run([output_name], {input_name: x})
plt.scatter(x.reshape(-1), result[0].reshape(-1))
3-5. 学習済みONNXモデルを使う
ONNX Model Zooにモデルがいろいろ置かれていますので、使いたいモデルがあるならライセンスを確認の上で利用すると良いでしょう
https://github.com/onnx/models
4. Tensorflow Kerasモデルを変換して使う
tf2onnxを使うとTensorflow, Keras, TFLITEモデルをONNXモデルに変換して利用することが出来ます
4-1. インストール
Pyhon環境の場合はpipで入ります
pip install tf2onnx
4-2. モデルの準備
今回はとりあえずモデルだけ作って特に学習はしないという手抜きモデルでやってみます
import numpy as np
import tensorflow as tf
import tf2onnx
import onnx
import onnxruntime
cnt, dim = 100, 4
inputs = tf.keras.layers.Input(4)
outputs = tf.keras.layers.Dense(1, activation='linear', name='output')(inputs)
model = tf.keras.models.Model(inputs, outputs)
model.summary()
x = np.arange(cnt * dim).reshape(cnt, dim).astype(np.float32)
print(x.shape)
y = model.predict(x)
print(y.shape)
4-3. モデルの変換
モデルを変換して書き出しますが、Kerasモデルは入力の次元が必ず決まっているとは限らないためか入力の構造をtf.TensorSpecの形で渡してやる必要があります。ここで1つめの軸がバッチサイズになりますが、バッチサイズを定数で指定するとそのサイズしか推論できなくなるようなので、入ってくるバッチサイズが不定の場合はNoneにしておきます。
model_path = "models/model.onnx"
input_signature = [tf.TensorSpec([None] + list(x.shape[1:]),
tf.float32, name='x')]
onnx_model, _ = tf2onnx.convert.from_keras(model, input_signature, opset=13)
onnx.save(onnx_model, model_path)
4-4. 変換したモデルで推論
onnxruntime.InferenceSession()でsessionを作ってrun()すると結果が取得できます
sess = onnxruntime.InferenceSession(model_path, providers=['CUDAExecutionProvider', 'CPUExecutionProvider'])
input_name = sess.get_inputs()[0].name
print("Input name :", input_name)
input_shape = sess.get_inputs()[0].shape
print("Input shape :", input_shape)
input_type = sess.get_inputs()[0].type
print("Input type :", input_type)
output_name = sess.get_outputs()[0].name
print("Output name :", output_name)
output_shape = sess.get_outputs()[0].shape
print("Output shape :", output_shape)
output_type = sess.get_outputs()[0].type
print("Output type :", output_type)
y2 = sess.run([output_name], {input_name: x})
sess.run()する際にinput_name, output_nameがそれぞれ必要ですが、sess.get_inputs()とsess.get_outputs()により取得できるのでこれをそのまま用いれば良いと思います。
4-5. 学習済みモデルを使う
Kerasモデルを変換できるのでTensorFlow Kerasのmodel zooも利用することもできるわけですね
5. PyTorchモデルを変換して使う
PyTorchの場合、torch.onnxにより変換ができるので追加のインストールは不要です
5-1. モデルの準備
PyTorchのmodel zooからResNet50を使ってみます
https://pytorch.org/serve/model_zoo.html
import torch
import torchvision
x = torch.randn(10, 3, 224, 224, device="cuda")
model = torchvision.models.alexnet(pretrained=True).cuda()
5-2. モデルの変換
torch.onnx.export()によりモデルを変換して書き出します
input_names = [ "actual_input_1" ] + [ "learned_%d" % i for i in range(16) ]
output_names = [ "output1" ]
model_path = './models/alexnet.onnx'
torch.onnx.export(model, dummy_input, model_path, verbose=True, input_names=input_names, output_names=output_names)
5-4. モデルの確認
onnx.checkerによりONNXモデルの構造を確認できます
import onnx
model = onnx.load(model_path)
onnx.checker.check_model(model)
print(onnx.helper.printable_graph(model.graph))
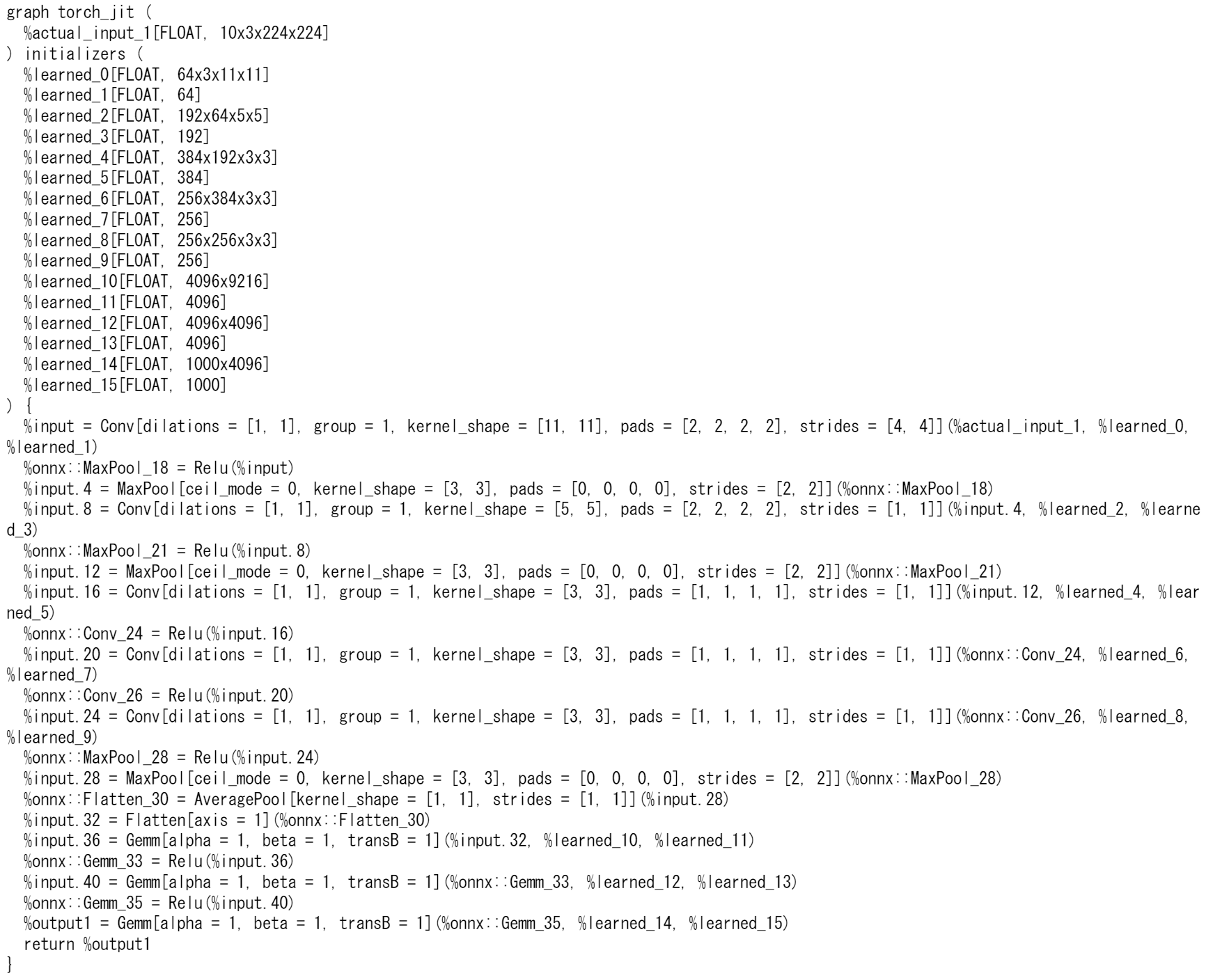
5-3. 変換したモデルで推論
作ってしまえば使い方は同じです
import numpy as np
import onnxruntime
sess = onnxruntime.InferenceSession(model_path,
providers=['CUDAExecutionProvider', 'CPUExecutionProvider'])
outputs = sess.run(
None,
{"actual_input_1": np.random.randn(10, 3, 224, 224).astype(np.float32)},
)
print(outputs[0])
5-4. 学習済みモデルを使う
PyTorchの学習済みモデルは以下に置いてあります
6. scikit-learnモデルを変換して使う
skl2onnxを使えばONNXモデルに変換できます
6-1. インストール
pipで入ります
pip install skl2onnx
6-2. モデルの準備
適当な回帰モデルを作ります
import onnx
import onnxruntime
import sklearn
from sklearn.svm import SVR
import matplotlib.pyplot as plt
x = np.linspace(0, 1, 1000)
y = x * 2
x = x.reshape(-1, 1)
model = sklearn.svm.SVR(C=1, epsilon=0.0001)
model.fit(x, y)
y_pred = model.predict(x)
print(np.round(y[::200], 2))
print(np.round(y_pred[::200], 2))
plt.figure(figsize=(4, 4))
plt.scatter(x, y_pred)
plt.plot(x, y, c='red', linewidth=3)
plt.show()
6-3. モデルの変換と推論
skl2onnx.to_onnx()によってscikit-learnモデルをONNXモデルに変換したものをシリアライズして、onnxruntime.InferenceSession()に渡すと推論sessionを作成できます。それ以降の使い方は同じです。
import skl2onnx
onnx_model = skl2onnx.to_onnx(model, x[:1].astype(np.float32), target_opset=12)
sess = onnxruntime.InferenceSession(onnx_model.SerializeToString(),
providers=['CUDAExecutionProvider', 'CPUExecutionProvider'])
pred_onnx = sess.run(None, {'X': x.astype(np.float32)})[0]
print("Onnx Runtime prediction:\n", np.round(pred_onnx[::200, 0], 2))
print("Sklearn rediction:\n", np.round(y_pred[::200], 2))
6-4. 変換したモデルの保存/読み込み
ONNXモデルをシリアライズしてバイナリで書き出しておけば、onnx.load()で読み込んで復元できるようです
model_path = './models/sklearn2.onnx'
with open(model_path, "wb") as f:
f.write(onnx_model.SerializeToString())
onnx_model2 = onnx.load(model_path, "wb")
7. lightGBMモデルを変換して使う
onnxmltoolsを使うとXGBoost, LightGBM, CatBoost, libsvm, H2Oなどのモデルを変換することができます
lightGBMの場合、ClassifierとRegressorで変換方法が違っていますが両方に対応してくれています
Convert a pipeline with a LightGBM classifier
http://onnx.ai/sklearn-onnx/auto_tutorial/plot_gexternal_lightgbm.html
Convert a pipeline with a LightGBM regressor
http://onnx.ai/sklearn-onnx/auto_tutorial/plot_gexternal_lightgbm_reg.html
7-1. インストール
pipで入ります
pip install onnxmltools
7-2. モデルの準備
適当にRegressorモデルを作ります
import packaging.version as pv
import warnings
import timeit
import numpy as np
from pandas import DataFrame
import matplotlib.pyplot as plt
from tqdm.notebook import tqdm
from lightgbm import LGBMRegressor
from onnxruntime import InferenceSession
import skl2onnx
from skl2onnx.common.shape_calculator import calculate_linear_regressor_output_shapes # noqa
from onnxmltools import __version__ as oml_version
from onnxmltools.convert.lightgbm.operator_converters.LightGbm import convert_lightgbm # noqa
N = 1000
X = np.random.randn(N, 20)
y = (np.random.randn(N) +
np.random.randn(N) * 100 * np.random.randint(0, 1, 1000))
model = LGBMRegressor(n_estimators=1000)
model.fit(X, y)
7-3. モデルの変換
まだ未完成なのかちょっとコード量が多いですし、何がどうなってるのかよく分からない部分が多いですが、とりあえずこれで変換はできました
def skl2onnx_convert_lightgbm(scope, operator, container):
options = scope.get_options(operator.raw_operator)
if 'split' in options:
if pv.Version(oml_version) < pv.Version('1.9.2'):
warnings.warn(
"Option split was released in version 1.9.2 but %s is "
"installed. It will be ignored." % oml_version)
operator.split = options['split']
else:
operator.split = None
convert_lightgbm(scope, operator, container)
skl2onnx.update_registered_converter(
LGBMRegressor, 'LightGbmLGBMRegressor',
calculate_linear_regressor_output_shapes,
skl2onnx_convert_lightgbm,
options={'split': None})
optionsでsplitを指定するとノードあたりのsplitを増やすことができます。splitを増やすと精度が幾分下がる代わりに処理速度が向上します
model_onnx = skl2onnx.to_onnx(model, X[:1].astype(np.float32),
target_opset={'': 14, 'ai.onnx.ml': 2})
model_onnx_split = skl2onnx.to_onnx(model, X[:1].astype(np.float32),
target_opset={'': 14, 'ai.onnx.ml': 2},
options={'split': 100})
7-4. 変換したモデルで推論
split=100のモデルとsplit=NoneのモデルでそれぞれInferenceSessionを作って元々のモデルとの差と処理時間を比較します
sess = InferenceSession(model_onnx.SerializeToString(), providers=['CUDAExecutionProvider', 'CPUExecutionProvider'])
sess_split = InferenceSession(model_onnx_split.SerializeToString(), providers=['CUDAExecutionProvider', 'CPUExecutionProvider'])
X32 = X.astype(np.float32)
expected = model.predict(X32)
got = sess.run(None, {'X': X32})[0].ravel()
got_split = sess_split.run(None, {'X': X32})[0].ravel()
disp = np.abs(got - expected).sum()
disp_split = np.abs(got_split - expected).sum()
print("sum of discrepancies 1 node", disp)
print("sum of discrepancies split node",
disp_split, "ratio:", disp / disp_split)
disc = np.abs(got - expected).max()
disc_split = np.abs(got_split - expected).max()
print("max discrepancies 1 node", disc)
print("max discrepancies split node", disc_split, "ratio:", disc / disc_split)
print("processing time no split",
timeit.timeit(
lambda: sess.run(None, {'X': X32})[0], number=150))
print("processing time split",
timeit.timeit(
lambda: sess_split.run(None, {'X': X32})[0], number=150))
今回は入力次元が少ないので速度向上幅が小さいですが、入力次元が大きいモデルの場合は精度差は同程度で速度差が大きくなるのでメリットが大きくなるようです
8. Python以外の言語でONNXモデルを使う
ここまでいろんなフレームワークのモデルをPythonで使う方法を書いてきましたが、ここからはPython以外の言語環境でデプロイする際のコードを見ていきたいと思います。
8-1. 対応言語いろいろ
公式が対応している言語は以下に一覧があります
https://onnxruntime.ai/docs/get-started/

上記にないものでもググるとGo言語向けらしきリポジトリやRust向けらしきリポジトリなどが見つかります。
8-2. NodeJSで使う
こちらのサンプルコードが分かりやすいです
https://github.com/microsoft/onnxruntime-inference-examples/tree/main/js/quick-start_onnxruntime-node
ONNXモデルを読みこんだInferenceSessionを作ってsession.run()するだけです
{
"name": "quick-start_onnxruntime-node",
"private": true,
"version": "1.0.0",
"description": "This example is a demonstration of basic usage of ONNX Runtime Node.js binding.",
"main": "index.js",
"dependencies": {
"onnxruntime-node": "^1.8.0"
}
}
// Copyright (c) Microsoft Corporation.
// Licensed under the MIT license.
const ort = require('onnxruntime-node');
// use an async context to call onnxruntime functions.
async function main() {
try {
// create a new session and load the specific model.
//
// the model in this example contains a single MatMul node
// it has 2 inputs: 'a'(float32, 3x4) and 'b'(float32, 4x3)
// it has 1 output: 'c'(float32, 3x3)
const session = await ort.InferenceSession.create('./model.onnx');
// prepare inputs. a tensor need its corresponding TypedArray as data
const dataA = Float32Array.from([1, 2, 3, 4, 5, 6, 7, 8, 9, 10, 11, 12]);
const dataB = Float32Array.from([10, 20, 30, 40, 50, 60, 70, 80, 90, 100, 110, 120]);
const tensorA = new ort.Tensor('float32', dataA, [3, 4]);
const tensorB = new ort.Tensor('float32', dataB, [4, 3]);
// prepare feeds. use model input names as keys.
const feeds = { a: tensorA, b: tensorB };
// feed inputs and run
const results = await session.run(feeds);
// read from results
const dataC = results.c.data;
console.log(`data of result tensor 'c': ${dataC}`);
} catch (e) {
console.error(`failed to inference ONNX model: ${e}.`);
}
}
main();
$ npm install
$ node .
data of result tensor 'c': 700,800,900,1580,1840,2100,2460,2880,3300
簡単ですね
8-3. Webで使う
ブラウザ上のJavascriptからクライアント側のリソースを使って推論することも出来ます。WasmやOpenGLを使って高速に処理される実装になっているようです
https://cloudblogs.microsoft.com/opensource/2021/09/02/onnx-runtime-web-running-your-machine-learning-model-in-browser/
サンプルコードは以下が分かりやすいと思います
https://github.com/microsoft/onnxruntime-inference-examples/tree/main/js/quick-start_onnxruntime-web-bundler
必要なpackageをpackage.jsonに書いておきます
{
"name": "quick-start_onnxruntime-web-bundler",
"private": true,
"version": "1.0.0",
"description": "This example is a demonstration of basic usage of ONNX Runtime Web using a bundler.",
"dependencies": {
"onnxruntime-web": "^1.8.0"
},
"devDependencies": {
"copy-webpack-plugin": "^8.1.1",
"webpack": "^5.36.2",
"webpack-cli": "^4.6.0"
}
}
onnxruntime用のWasmプラグインをバンドルします。この辺よく分かってないので分かったら追記予定です。
// Copyright (c) Microsoft Corporation.
// Licensed under the MIT license.
const path = require('path');
const CopyPlugin = require("copy-webpack-plugin");
module.exports = () => {
return {
target: ['web'],
entry: path.resolve(__dirname, 'main.js'),
output: {
path: path.resolve(__dirname, 'dist'),
filename: 'bundle.min.js',
library: {
type: 'umd'
}
},
plugins: [new CopyPlugin({
// Use copy plugin to copy *.wasm to output folder.
patterns: [{ from: 'node_modules/onnxruntime-web/dist/*.wasm', to: '[name][ext]' }]
})],
mode: 'production'
}
};
後はInferenceSessionを作ってsession.run()するだけです
// Copyright (c) Microsoft Corporation.
// Licensed under the MIT license.
const ort = require('onnxruntime-web');
// use an async context to call onnxruntime functions.
async function main() {
try {
// create a new session and load the specific model.
//
// the model in this example contains a single MatMul node
// it has 2 inputs: 'a'(float32, 3x4) and 'b'(float32, 4x3)
// it has 1 output: 'c'(float32, 3x3)
const session = await ort.InferenceSession.create('./model.onnx');
// prepare inputs. a tensor need its corresponding TypedArray as data
const dataA = Float32Array.from([1, 2, 3, 4, 5, 6, 7, 8, 9, 10, 11, 12]);
const dataB = Float32Array.from([10, 20, 30, 40, 50, 60, 70, 80, 90, 100, 110, 120]);
const tensorA = new ort.Tensor('float32', dataA, [3, 4]);
const tensorB = new ort.Tensor('float32', dataB, [4, 3]);
// prepare feeds. use model input names as keys.
const feeds = { a: tensorA, b: tensorB };
// feed inputs and run
const results = await session.run(feeds);
// read from results
const dataC = results.c.data;
document.write(`data of result tensor 'c': ${dataC}`);
} catch (e) {
document.write(`failed to inference ONNX model: ${e}.`);
}
}
main();
<!DOCTYPE html>
<html>
<header>
<title>ONNX Runtime JavaScript examples: Quick Start - Web (using bundler)</title>
</header>
<body>
<!-- consume a single file bundle -->
<script src="./dist/bundle.min.js"></script>
</body>
</html>
Webの作例
Microsoftが作ったカッコいい作例が以下にあります
https://microsoft.github.io/onnxruntime-web-demo/#/
この作例のコードが以下にあるので、ここを参考にいろいろ作れそうです
https://github.com/microsoft/onnxruntime-web-demo/
9. まとめ
似たようなフレームワークにOpenVINOというのもあるようですがONNXの方が対応範囲が広くて使い勝手が良さそうです。配布されるモデルのフォーマットとしてもONNXが選択されることが多いようなので、今後ドキュメントが増えてくればデファクトスタンダードになっていくんじゃないかと思います。