概要
各種Keras学習済みモデルをFruits 360データセットでFine-tuningさせ、クラス分類モデルを構築
モデル精度を比較する
対象モデル
- VGG16
- InceptionV3
- Xception
- MobileNet
Fruits 360
https://www.kaggle.com/moltean/fruits
- KaggleのFruits 360 dataset
- 81クラス、55244枚の画像データセット
実行環境
Google Colaboratory(GPU)
import numpy as np
import pandas as pd
import matplotlib.pyplot as plt
%config InlineBackend.figure_formats = {'png', 'retina'}
import os, cv2, zipfile, io, re, glob
from PIL import Image
from sklearn.model_selection import train_test_split
from keras.applications.vgg16 import VGG16
from keras.applications.inception_v3 import InceptionV3
from keras.applications.inception_resnet_v2 import InceptionResNetV2
from keras.applications.xception import Xception
from keras.models import Model, load_model
from keras.layers.core import Dense
from keras.layers.pooling import GlobalAveragePooling2D
from keras.optimizers import Adam, RMSprop, SGD
from keras.utils.np_utils import to_categorical
from keras.callbacks import ModelCheckpoint, EarlyStopping, TensorBoard, ReduceLROnPlateau
from keras.preprocessing.image import ImageDataGenerator
データ取得
81クラスの内、30クラスの画像を取得。
# ZIP読み込み
z = zipfile.ZipFile('../dataset/fruits-360.zip')
# ラベリングされたディレクトリのみ取得
img_dirs = [ x for x in z.namelist() if re.search("^fruits-360/Training/.*/$", x)]
# 不要な文字列削除
img_dirs = [ x.replace('fruits-360/Training/', '') for x in img_dirs]
img_dirs = [ x.replace('/', '') for x in img_dirs]
img_dirs.sort()
# クラス取得
classes = img_dirs
# クラス数
# num_classes = len(classes)
num_classes = 30
del img_dirs
画像は150にリサイズ後、配列に変換
# 画像サイズ
image_size = 150
# 画像を取得し、配列に変換
def im2array(path):
X = []
y = []
class_num = 0
for class_name in classes:
if class_num == num_classes : break
imgfiles = [ x for x in z.namelist() if re.search("^" + path + class_name + "/.*jpg$", x)]
for imgfile in imgfiles:
# ZIPから画像読み込み
image = Image.open(io.BytesIO(z.read(imgfile)))
# RGB変換
image = image.convert('RGB')
# リサイズ
image = image.resize((image_size, image_size))
# 画像から配列に変換
data = np.asarray(image)
X.append(data)
y.append(classes.index(class_name))
class_num += 1
X = np.array(X)
y = np.array(y)
return X, y
trainデータ取得
%%time
X_train, y_train = im2array("fruits-360/Training/")
print(X_train.shape, y_train.shape)
(15094, 150, 150, 3) (15094,)
CPU times: user 14.1 s, sys: 1.56 s, total: 15.7 s
Wall time: 15.9 s
testデータ取得
%%time
X_test, y_test = im2array("fruits-360/Test/")
print(X_test.shape, y_test.shape)
(5060, 150, 150, 3) (5060,)
CPU times: user 8.02 s, sys: 307 ms, total: 8.32 s
Wall time: 8.39 s
del z
# データ型の変換
X_train = X_train.astype('float32')
X_test = X_test.astype('float32')
# 正規化
X_train /= 255
X_test /= 255
# one-hot 変換
y_train = to_categorical(y_train, num_classes = num_classes)
y_test = to_categorical(y_test, num_classes = num_classes)
print(y_train.shape, y_test.shape)
(15094, 30) (5060, 30)
trainデータからvalidデータを分割
X_train, X_valid, y_train, y_valid = train_test_split(
X_train,
y_train,
random_state = 0,
stratify = y_train,
test_size = 0.2
)
print(X_train.shape, y_train.shape, X_valid.shape, y_valid.shape)
(12075, 150, 150, 3) (12075, 30) (3019, 150, 150, 3) (3019, 30)
学習済みモデルの精度比較
Data Augmentation
datagen = ImageDataGenerator(
featurewise_center = False,
samplewise_center = False,
featurewise_std_normalization = False,
samplewise_std_normalization = False,
zca_whitening = False,
rotation_range = 0,
width_shift_range = 0.1,
height_shift_range = 0.1,
horizontal_flip = True,
vertical_flip = False
)
Callback
# EarlyStopping
early_stopping = EarlyStopping(
monitor = 'val_loss',
patience = 10,
verbose = 1
)
# ModelCheckpoint
weights_dir = './weights/'
if os.path.exists(weights_dir) == False:os.mkdir(weights_dir)
model_checkpoint = ModelCheckpoint(
weights_dir + "val_loss{val_loss:.3f}.hdf5",
monitor = 'val_loss',
verbose = 1,
save_best_only = True,
save_weights_only = True,
period = 3
)
# reduce learning rate
reduce_lr = ReduceLROnPlateau(
monitor = 'val_loss',
factor = 0.1,
patience = 3,
verbose = 1
)
# log for TensorBoard
logging = TensorBoard(log_dir = "log/")
各種関数定義
# モデル学習
def model_fit():
hist = model.fit_generator(
datagen.flow(X_train, y_train, batch_size = 32),
steps_per_epoch = X_train.shape[0] // 32,
epochs = 50,
validation_data = (X_valid, y_valid),
callbacks = [early_stopping, reduce_lr],
shuffle = True,
verbose = 1
)
return hist
# モデル保存
model_dir = './model/'
if os.path.exists(model_dir) == False : os.mkdir(model_dir)
def model_save(model_name):
model.save(model_dir + 'model_' + model_name + '.hdf5')
# optimizerのない軽量モデルを保存(学習や評価不可だが、予測は可能)
model.save(model_dir + 'model_' + model_name + '-opt.hdf5', include_optimizer = False)
# 学習曲線をプロット
def learning_plot(title):
plt.figure(figsize = (18,6))
# accuracy
plt.subplot(1, 2, 1)
plt.plot(hist.history["acc"], label = "acc", marker = "o")
plt.plot(hist.history["val_acc"], label = "val_acc", marker = "o")
#plt.yticks(np.arange())
#plt.xticks(np.arange())
plt.ylabel("accuracy")
plt.xlabel("epoch")
plt.title(title)
plt.legend(loc = "best")
plt.grid(color = 'gray', alpha = 0.2)
# loss
plt.subplot(1, 2, 2)
plt.plot(hist.history["loss"], label = "loss", marker = "o")
plt.plot(hist.history["val_loss"], label = "val_loss", marker = "o")
#plt.yticks(np.arange())
#plt.xticks(np.arange())
plt.ylabel("loss")
plt.xlabel("epoch")
plt.title(title)
plt.legend(loc = "best")
plt.grid(color = 'gray', alpha = 0.2)
plt.show()
# モデル評価
def model_evaluate():
score = model.evaluate(X_test, y_test, verbose = 1)
print("evaluate loss: {[0]:.4f}".format(score))
print("evaluate acc: {[1]:.1%}".format(score))
VGG16
base_model = VGG16(
include_top = False,
weights = "imagenet",
input_shape = None
)
# 全結合層の新規構築
x = base_model.output
x = GlobalAveragePooling2D()(x)
x = Dense(1024, activation = 'relu')(x)
predictions = Dense(num_classes, activation = 'softmax')(x)
# ネットワーク定義
model = Model(inputs = base_model.input, outputs = predictions)
print("{}層".format(len(model.layers)))
22層
全22層の内、17層までfreezeさせ、18層以降を学習させる。
# 17層までfreeze
for layer in model.layers[:17]:
layer.trainable = False
# 18層以降、学習させる
for layer in model.layers[17:]:
layer.trainable = True
# layer.trainableの設定後にcompile
model.compile(
optimizer = Adam(),
loss = 'categorical_crossentropy',
metrics = ["accuracy"]
)
%%time
hist = model_fit()
Epoch 1/50
377/377 [==============================] - 98s 260ms/step - loss: 0.4985 - acc: 0.8490 - val_loss: 0.1256 - val_acc: 0.9530
Epoch 2/50
377/377 [==============================] - 96s 255ms/step - loss: 0.0727 - acc: 0.9732 - val_loss: 0.0732 - val_acc: 0.9665
Epoch 3/50
377/377 [==============================] - 96s 255ms/step - loss: 0.0581 - acc: 0.9770 - val_loss: 0.0297 - val_acc: 0.9851
〜省略〜
Epoch 24/50
377/377 [==============================] - 94s 248ms/step - loss: 0.0203 - acc: 0.9871 - val_loss: 0.0218 - val_acc: 0.9834
Epoch 25/50
377/377 [==============================] - 93s 247ms/step - loss: 0.0206 - acc: 0.9854 - val_loss: 0.0218 - val_acc: 0.9834
Epoch 26/50
377/377 [==============================] - 93s 246ms/step - loss: 0.0204 - acc: 0.9864 - val_loss: 0.0218 - val_acc: 0.9834
Epoch 00026: ReduceLROnPlateau reducing learning rate to 1.000000082740371e-08.
Epoch 27/50
377/377 [==============================] - 92s 245ms/step - loss: 0.0203 - acc: 0.9872 - val_loss: 0.0218 - val_acc: 0.9834
Epoch 00027: early stopping
CPU times: user 50min 41s, sys: 4min 41s, total: 55min 22s
Wall time: 42min 35s
learning_plot("VGG16")

model_evaluate()
5060/5060 [==============================] - 24s 5ms/step
evaluate loss: 0.1116
evaluate acc: 96.1%
model_save("VGG16")
InceptionV3
base_model = InceptionV3(
include_top = False,
weights = "imagenet",
input_shape = None
)
# 全結合層の新規構築
x = base_model.output
x = GlobalAveragePooling2D()(x)
x = Dense(1024, activation = 'relu')(x)
predictions = Dense(num_classes, activation = 'softmax')(x)
# ネットワーク定義
model = Model(inputs = base_model.input, outputs = predictions)
print("{}層".format(len(model.layers)))
314層
全314層の内、249層までfreezeさせ、250層以降を学習させる。
# 249層までfreeze
for layer in model.layers[:249]:
layer.trainable = False
# Batch Normalization の freeze解除
if layer.name.startswith('batch_normalization'):
layer.trainable = True
# 250層以降、学習させる
for layer in model.layers[249:]:
layer.trainable = True
# layer.trainableの設定後にcompile
model.compile(
optimizer = Adam(),
loss = 'categorical_crossentropy',
metrics = ["accuracy"]
)
%%time
hist=model_fit()
Epoch 1/50
377/377 [==============================] - 148s 394ms/step - loss: 0.3223 - acc: 0.9127 - val_loss: 0.0609 - val_acc: 0.9748
Epoch 2/50
377/377 [==============================] - 129s 343ms/step - loss: 0.0962 - acc: 0.9684 - val_loss: 0.0469 - val_acc: 0.9781
Epoch 3/50
377/377 [==============================] - 129s 343ms/step - loss: 0.0676 - acc: 0.9756 - val_loss: 0.0365 - val_acc: 0.9821
〜省略〜
Epoch 19/50
377/377 [==============================] - 129s 341ms/step - loss: 0.0225 - acc: 0.9859 - val_loss: 0.0210 - val_acc: 0.9844
Epoch 20/50
377/377 [==============================] - 128s 340ms/step - loss: 0.0235 - acc: 0.9857 - val_loss: 0.0210 - val_acc: 0.9844
Epoch 21/50
377/377 [==============================] - 129s 342ms/step - loss: 0.0210 - acc: 0.9865 - val_loss: 0.0210 - val_acc: 0.9848
Epoch 00021: ReduceLROnPlateau reducing learning rate to 1.000000082740371e-08.
Epoch 00021: early stopping
CPU times: user 1h 8min 40s, sys: 9min 16s, total: 1h 17min 56s
Wall time: 45min 28s
learning_plot("InceptionV3")
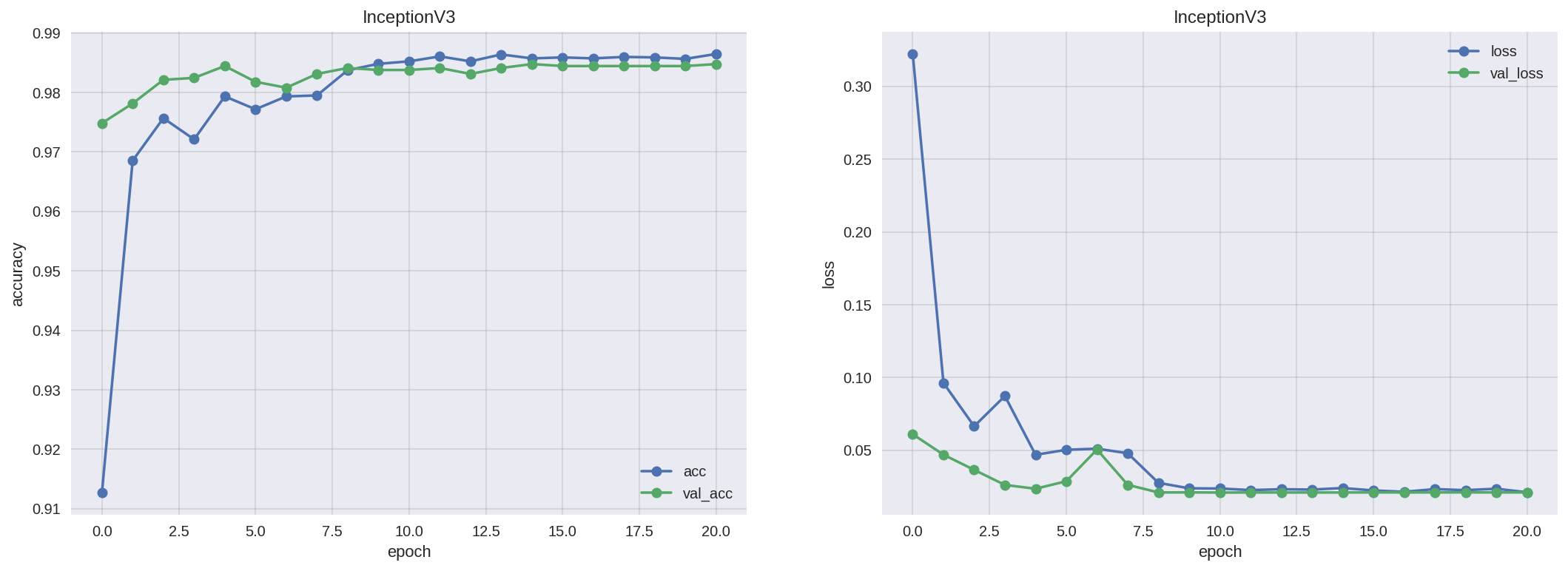
model_evaluate()
5060/5060 [==============================] - 15s 3ms/step
evaluate loss: 0.2082
evaluate acc: 96.5%
model_save("InceptionV3")
VGG16に比べ、accuracyは良くなったが、代わりにlossが増え、必ずしも精度が高いとは言い切れない。
Xception
base_model = Xception(
include_top = False,
weights = "imagenet",
input_shape = None
)
# 全結合層の新規構築
x = base_model.output
x = GlobalAveragePooling2D()(x)
x = Dense(1024, activation = 'relu')(x)
predictions = Dense(num_classes, activation = 'softmax')(x)
# ネットワーク定義
model = Model(inputs = base_model.input, outputs = predictions)
print("{}層".format(len(model.layers)))
135層
全135層の内、108層までfreezeさせ、109層以降を学習させる。
# 108層までfreeze
for layer in model.layers[:108]:
layer.trainable = False
# Batch Normalization の freeze解除
if layer.name.startswith('batch_normalization'):
layer.trainable = True
if layer.name.endswith('bn'):
layer.trainable = True
# 109層以降、学習させる
for layer in model.layers[108:]:
layer.trainable = True
# layer.trainableの設定後にcompile
model.compile(
optimizer = Adam(),
loss = 'categorical_crossentropy',
metrics = ["accuracy"]
)
%%time
hist = model_fit()
Epoch 1/50
377/377 [==============================] - 172s 455ms/step - loss: 0.2736 - acc: 0.9318 - val_loss: 0.0859 - val_acc: 0.9669
Epoch 2/50
377/377 [==============================] - 162s 429ms/step - loss: 0.1284 - acc: 0.9626 - val_loss: 0.0389 - val_acc: 0.9824
Epoch 3/50
377/377 [==============================] - 161s 426ms/step - loss: 0.0882 - acc: 0.9724 - val_loss: 0.0275 - val_acc: 0.9824
〜省略〜
Epoch 16/50
377/377 [==============================] - 161s 427ms/step - loss: 0.0220 - acc: 0.9862 - val_loss: 0.0232 - val_acc: 0.9838
Epoch 17/50
377/377 [==============================] - 161s 427ms/step - loss: 0.0219 - acc: 0.9864 - val_loss: 0.0229 - val_acc: 0.9841
Epoch 18/50
377/377 [==============================] - 161s 427ms/step - loss: 0.0228 - acc: 0.9859 - val_loss: 0.0226 - val_acc: 0.9844
Epoch 00018: ReduceLROnPlateau reducing learning rate to 1.0000000656873453e-06.
Epoch 19/50
377/377 [==============================] - 161s 427ms/step - loss: 0.0229 - acc: 0.9865 - val_loss: 0.0226 - val_acc: 0.9844
Epoch 00019: early stopping
CPU times: user 1h 41s, sys: 12min 51s, total: 1h 13min 33s
Wall time: 51min 20s
learning_plot("Xception")
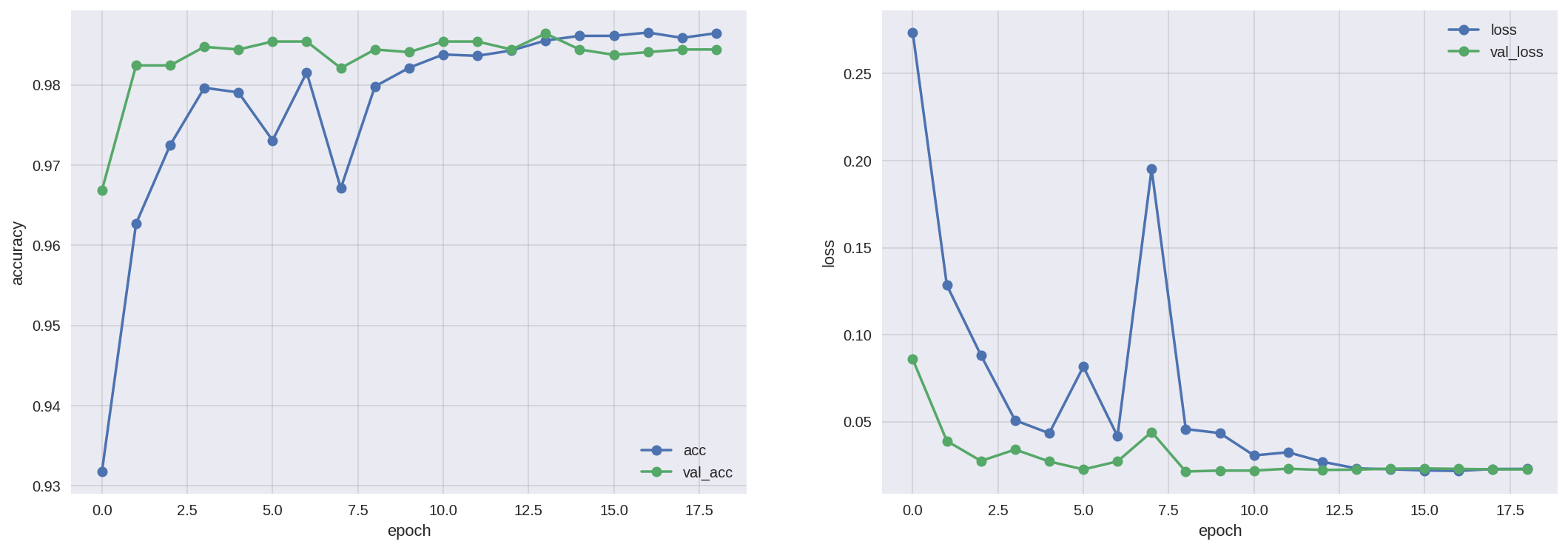
model_evaluate()
5060/5060 [==============================] - 25s 5ms/step
evaluate loss: 0.0977
evaluate acc: 97.7%
model_save("Xception")
VGG16やInceptionV3に比べ、accuracy、lossともに精度が高い。
MobileNet
base_model = MobileNet(
include_top = False,
weights = "imagenet",
input_shape = None
)
# 全結合層の新規構築
x = base_model.output
x = GlobalAveragePooling2D()(x)
x = Dense(1024, activation = 'relu')(x)
predictions = Dense(num_classes, activation = 'softmax')(x)
# ネットワーク定義
model = Model(inputs = base_model.input, outputs = predictions)
print("{}層".format(len(model.layers)))
90層
全90層の内、72層までfreezeさせ、73層以降を学習させる。
# 72層までfreeze
for layer in model.layers[:72]:
layer.trainable = False
# Batch Normalization の freeze解除
if "bn" in layer.name:
layer.trainable = True
# 73層以降、学習させる
for layer in model.layers[72:]:
layer.trainable = True
# layer.trainableの設定後にcompile
model.compile(
optimizer = Adam(),
loss = 'categorical_crossentropy',
metrics = ["accuracy"]
)
%%time
hist = model_fit()
Epoch 1/50
377/377 [==============================] - 92s 244ms/step - loss: 0.0857 - acc: 0.9759 - val_loss: 0.2791 - val_acc: 0.9543
Epoch 2/50
377/377 [==============================] - 79s 210ms/step - loss: 0.0938 - acc: 0.9746 - val_loss: 0.5770 - val_acc: 0.9232
Epoch 3/50
377/377 [==============================] - 80s 212ms/step - loss: 0.0881 - acc: 0.9758 - val_loss: 0.1943 - val_acc: 0.9629
〜省略〜
Epoch 19/50
377/377 [==============================] - 82s 217ms/step - loss: 0.0209 - acc: 0.9859 - val_loss: 0.0212 - val_acc: 0.9854
Epoch 20/50
377/377 [==============================] - 81s 216ms/step - loss: 0.0204 - acc: 0.9867 - val_loss: 0.0212 - val_acc: 0.9858
Epoch 21/50
377/377 [==============================] - 81s 216ms/step - loss: 0.0197 - acc: 0.9867 - val_loss: 0.0212 - val_acc: 0.9854
Epoch 00021: ReduceLROnPlateau reducing learning rate to 1.0000001111620805e-07.
Epoch 22/50
377/377 [==============================] - 82s 216ms/step - loss: 0.0216 - acc: 0.9869 - val_loss: 0.0212 - val_acc: 0.9858
Epoch 00022: early stopping
CPU times: user 42min 23s, sys: 3min 30s, total: 45min 53s
Wall time: 29min 59s
learning_plot("MobileNet")
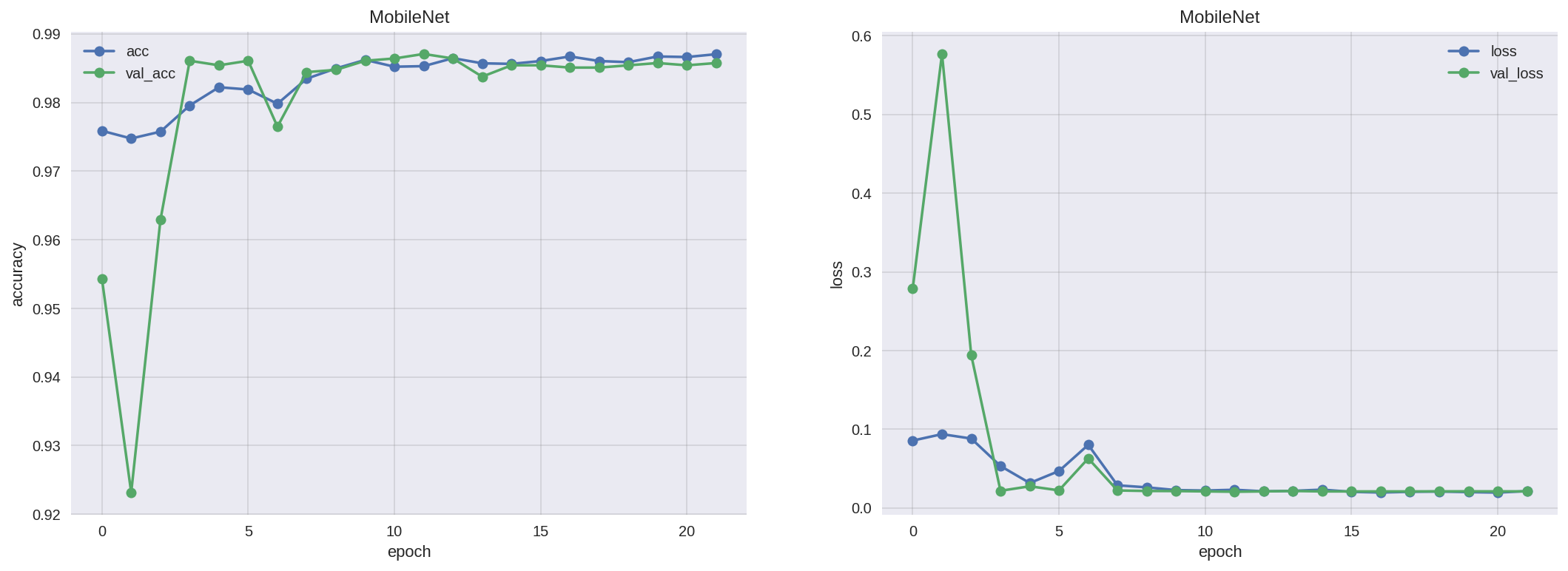
model_evaluate()
5060/5060 [==============================] - 7s 1ms/step
evaluate loss: 0.1705
evaluate acc: 97.6%
model_save("MobileNet")
エポックごとの学習速度が、最も早い。
データセットに依るのだろうが、accuracyがVGG16やInceptionV3よりも高く、Xceptionに近い値を計測したことが驚きである。
モデルの精度比較
accuracy
Xception ≒ MobileNet > Inception > VGG16
loss
Xception < VGG16 < MobileNet < Inception
各エポックごとの学習速度
MobileNet > VGG16 > Inception > Xception
今後はAWSを使って、InceptionResNetV2モデルを検証してみたい。
モデル予測
最後に最も精度が高かったXceptionモデルで、testデータを予測。
モデル読み込み
model = load_model(model_dir + 'model_Xception-opt.hdf5', compile = False)
# 相互のインデックスを対応させながらシャッフル
def shuffle_samples(X, y):
zipped = list(zip(X, y))
np.random.shuffle(zipped)
X_result, y_result = zip(*zipped)
return np.asarray(X_result), np.asarray(y_result)
X_test, y_test = shuffle_samples(X_test, y_test)
testデータ30件の画像と正解ラベルを出力
# testデータ30件の正解ラベル
true_classes = np.argmax(y_test[0:30], axis = 1)
# testデータ30件の画像と正解ラベルを出力
plt.figure(figsize = (16, 6))
for i in range(30):
plt.subplot(3, 10, i + 1)
plt.axis("off")
plt.title(classes[true_classes[i]])
plt.imshow(X_test[i])
plt.show()

testデータ30件の画像と予測ラベル・予測確率を出力
# testデータ30件の予測ラベル
pred_classes = np.argmax(model.predict(X_test[0:30]), axis = 1)
# testデータ30件の予測確率
pred_probs = np.max(model.predict(X_test[0:30]), axis = 1)
pred_probs = ['{:.4f}'.format(i) for i in pred_probs]
# testデータ30件の画像と予測ラベル・予測確率を出力
plt.figure(figsize = (16, 6))
for i in range(30):
plt.subplot(3, 10, i + 1)
plt.axis("off")
if pred_classes[i] == true_classes[i]:
plt.title(classes[pred_classes[i]] + '\n' + pred_probs[i])
else:
plt.title(classes[pred_classes[i]] + '\n' + pred_probs[i], color = "red")
plt.imshow(X_test[i])
plt.show()
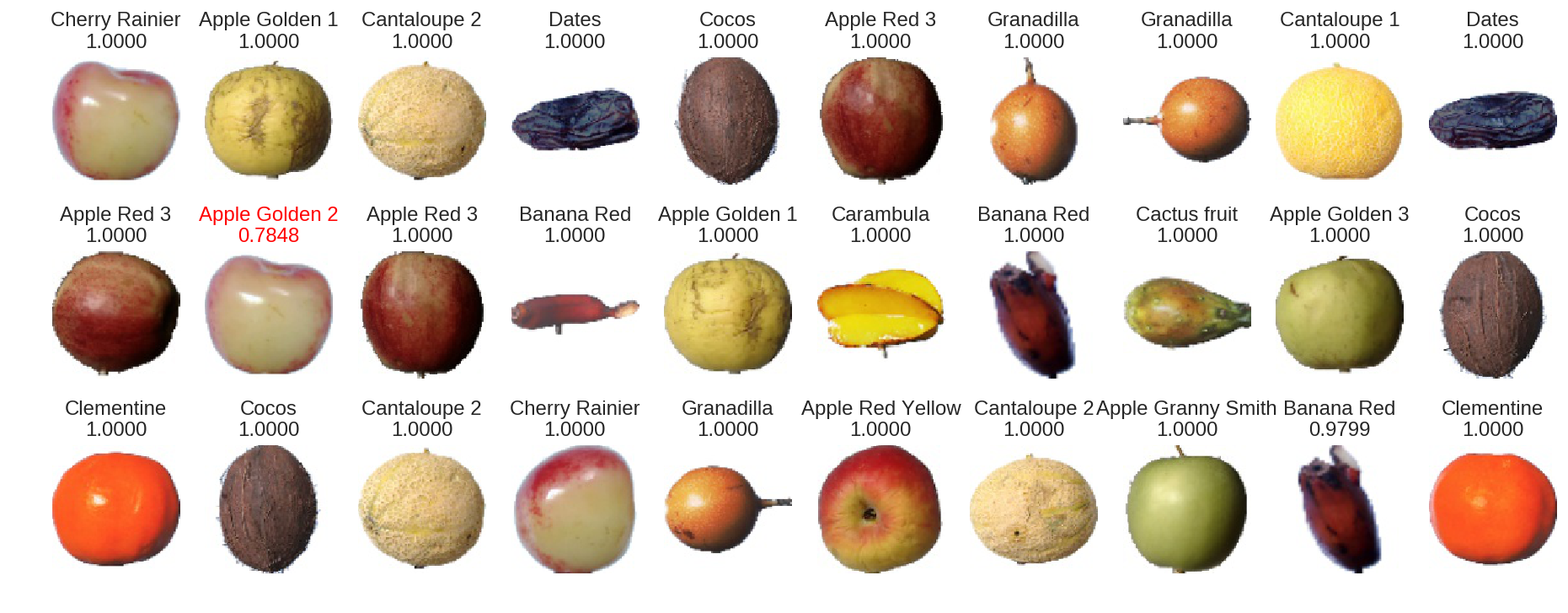
高い確率でクラス分類できているかなと。