目的
質的変数(カテゴリー変数)をOne-hotベクトルに変換する
使用データ・環境
データ:kaggleのTitanicデータ
環境:kaggle notebook
方法
onehot_encoding.py
# モジュールのインポート,osの準備
import numpy as np
import pandas as pd
import matplotlib as plt
import os
for dirname, _, filenames in os.walk('/kaggle/input'):
for filename in filenames:
print(os.path.join(dirname, filename))
データを読み込む
onehot_encoding.py
train_data=pd.read_csv('../input/titanic/train.csv')
test_data=pd.read_csv('../input/titanic/test.csv')
データを見てみる
onehot_encoding.py
train.data.head()

カテゴリー変数のデータフレームがいくつかあることがわかる.これらををOne-hotベクトルに変換することを狙う.
とりあえず,文字列のままだと扱いにくいので,各カテゴリーに異なる数値を割り当てる.
Pandasのfactorize()
を使う.
factorize()
は,数値のデータ(emb_cat_encoded)とカテゴリーのリスト(emb_categories)の両方を返す.
onehot_encoding.py
train_cat=train_data['Embarked']
train_cat_encoded,train_categories=train_cat.factorize()
# 見てみる
print(train_cat.head())
print(train_cat_encoded[:10])
print(train_categories)

次にone-hotベクトルへの変換
scikit-learnが提供しているOneHotEncoderを用いる.
onehot_encoding.py
# scikit-learnからOneHotEncoderをインポート
from sklearn.preprocessing import OneHotEncoder
# one-hotベクトルに変換
oe=OneHotEncoder(categories='auto')
train_cat_1hot=oe.fit_transform(train_cat_encoded.reshape(-1,1))
# 中を見てみる
train_cat_1hot
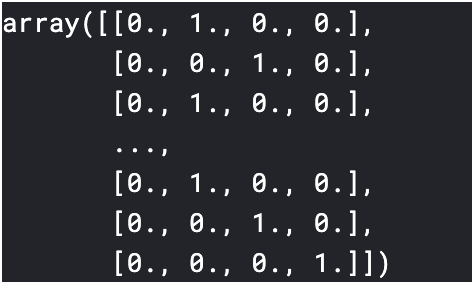
変換完了.