KerasのImageDataGeneratorを使用すると簡単にデータ拡張が実現できます。
ImageDataGeneratorの使用方法については以下の記事が参考になりますが、
複数の画像を入力したときにどのような順番で画像が出力されるか気になったので調べてみました。
Kerasによるデータ拡張
Kerasでデータ拡張(Data Augmentation)後の画像を表示する
使用したコードと概要
from tensorflow.keras.preprocessing.image import ImageDataGenerator
from tensorflow.keras.preprocessing import image
import numpy as np
import matplotlib.pyplot as plt
def load_img(name):
img = image.load_img(name, target_size=(256, 256))
return image.img_to_array(img) / 255
def show_imgs(imgs, row, col):
for i, img in enumerate(imgs):
plot_num = i+1
plt.subplot(row, col, plot_num)
plt.tick_params(labelbottom=False)
plt.tick_params(labelleft=False)
plt.imshow(img)
plt.show()
imgs = np.array([load_img('b.png'), load_img('c.png'), load_img('t.png')])
# 各画像にラベルを付けるための配列
labels = np.array([0,1,2])
# 画像の表示
show_imgs(imgs, 1, 3)
使用した3枚の画像はペイントで適当に作った以下のものです。

ImageDataGeneratorは以下のコードで作成します。
datagen = ImageDataGenerator(
rotation_range=40.,
width_shift_range=0.3,
height_shift_range=0.3,
zoom_range=0.3)
# 全体を見る必要があるパラメータを使用する場合はfitが必要
# 今回は特に必要ないけど一応やっておく
datagen.fit(imgs)
# 指定したバッチサイズで3回生成する
def create(batch_size):
gen = datagen.flow(imgs, labels, batch_size=batch_size)
for i in range(0, 3):
data = next(gen)
print('len:{}, labels:{}'.format(len(data[0]), data[1]))
show_imgs(data[0], 1, len(data[0]))
画像を生成する
バッチサイズと画像の総数が同じ場合
create(3)
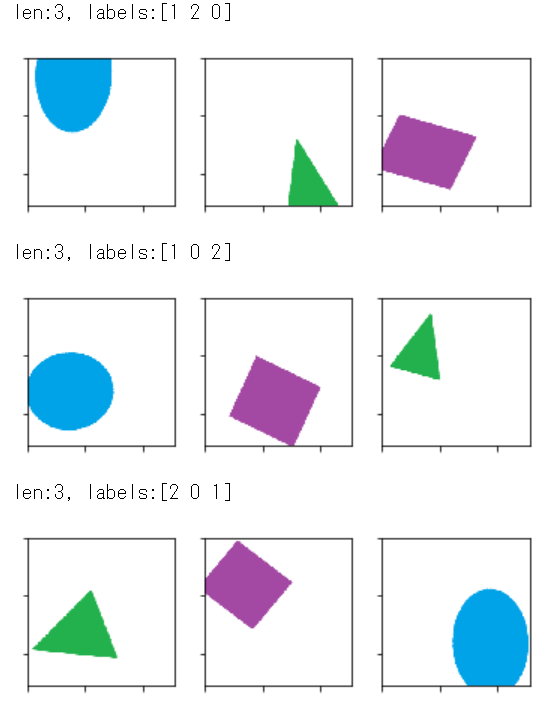
ランダムな順番で画像が生成されているみたいです。
元画像と全く同じものは出力されず、変形が加えられたもののみが出力されています。
バッチサイズのほうが画像の総数より少ない場合
create(2)

2,1,2...
というような感じで出力されるようです。
バッチサイズのほうが総数より多い場合
create(10)
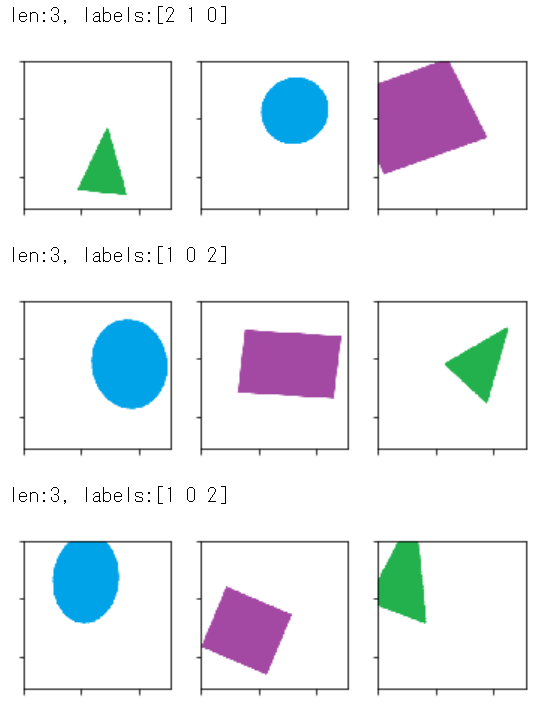
画像の総数が1バッチの最大個数のようです。
まとめ
画像はランダムな順番で出力される。
元々の画像は出力されず常に変形されたものが出力される。
画像の総数より小さいバッチサイズを指定するとすべての画像を使い切るまで同じ画像をもととしたものは現れない。