はじめに
実験で得られるデータには測定データ本体と測定時の条件などを記録したヘッダーなどと呼ばれるメタ情報があります。日々得られる測定データを適切に管理するためにはメタ情報は大切な情報です。多くの測定装置はメーカー独自あるいは汎用のバイナリ形式とテキスト形式の出力に対応しています。テキスト形式は非常に単純なフォーマットであるためOSやマシンパワーに関係なく中身を確認できる汎用性が魅力です。しかし、メタ情報に関しては、書き出しの実装が非常に楽なのに対して、読み込みには多少の文字列処理が必要となり急に厄介になります。汎用バイナリ形式の一つであるHDF1は、一つのファイルの中に階層構造を持たせ、いろいろな種類のデータ(文字、数字、行列、画像など)を保存することができます。メタ情報の書き出しと読み込みに非対称的な難易度があるテキスト形式に比べて、HDFファイルに格納されたメタ情報の読み書きはどちらもファイル内のパスを指定するだけであり、操作が対称的で非常にわかりやすいです。開発を進めるNPO団体"The HDF Group"の公式サイトで紹介されている通り、HDFはI/O速度も速く大容量データも扱えるオープンソースフォーマットであり、学術研究から産業界まで幅広い分野で使われています2。
kerasやchainerなどのニューラルネットワークライブラリの学習結果を保存するフォーマットとして採用されているようで、それらを通して初めてHDFを知った方も多いでしょう3。幅広い分野で使われているということは、おそらくHDFを出入力に使えるGUIソフトやライブラリはたくさんあるはずなのですが、どうも自作のスクリプトでどう扱えばいいのかという情報は(少なくとも日本語では)かなり少ないようです。たとえばCやFortranはPythonより先にHDFを扱えるようになっていたようですが、データの読み方 その3 HDF5 ライブラリ利用編 - JAXA AMSR‐2 データ利用講習会 とHDF5 with Fortran90 入門くらいしか日本語の説明が見つかりません4。
本記事の目的
h5pyというパッケージを使って自作のPythonスクリプトでHDF5ファイルを読み込む実践的な方法を紹介します。具体的には「自分で作ったわけではない(つまり階層構造を把握できていない)HDFファイルから欲しい情報を探して抽出してまとめたい」という状況を想定しています。Pythonを使ってHDFファイルの読み書きをする方法を説明した以下のような日本語記事はすでにいくつかありますが、中身の把握に言及しているものはありませんでした。またPython 3では使えなくなった仕様5を使って説明しているものもあります。
- HDF5形式のファイル (2) pythonを使った書き出し - ねるねるねるねをねらずにくうぜ
- HDF5形式のファイル (3) pythonを使った読み込み - ねるねるねるねをねらずにくうぜ
- Python HDF5 - @//メモ
- h5py | バイナリーデータファイルのフォーマット HDF を扱う Python ライブラリー
- Python + h5py で HDF5 ファイルの読み書き - Qiita
h5py公式マニュアルのチュートリアルには階層構造を把握するためのヒント(visit()
の部分)は書いてあります。ただ、これに触れた日本語記事はまだないようです。また、当然ながらGUIでHDFファイルの中身を確認できるHDF Viewを使って、ぽちぽちとクリックして階層構造を把握することも可能ですが、階層が複数に渡るとかなり大変です。
準備
h5py, pandasを使います。
import h5py
import pandas as pd
h5pyのインストールはconda
とpip
が使えます。conda
の場合はconda-forge
チャンネルの方が最新版の反映が早い印象があります。
conda install -c conda-forge h5py
pip install h5py
HDF5とh5pyの基本概念
HDF5の階層構造はコンピュータの階層構造とほぼ同じです。ただし、以下の表に示すように各要素の名前が違います。
コンピュータ | HDF5 |
---|---|
フォルダ、ディレクトリ | Group |
ファイル | Dataset |
プロパティ | Attribute |
h5pyにおいてはGroup
はディクショナリー、Dataset
はnumpy arrayのように扱われます。Attribute
は使ったことがないのですが、例えばdata
という名前のDataset
に温度を表す数字temperature
を紐づけておきたいときはtemperature
用に別のDataset
を用意することなく、たとえばdata.temperature
のように属性として保持させることができるようです。
cf. Quick Start Guide — h5py 2.8.0.post0 documentation
HDF5の階層構造とメリットについては以下が参考になります。
- HDF5形式のファイル (1) HDF5って? - ねるねるねるねをねらずにくうぜ
- [HDF5フォーマットに関するメモ書き - たまに書きます。] (http://yukisakamoto.hatenablog.com/entry/20130413/1365825028)
- The HDF5® Library & File Format - The HDF Group
階層構造を把握する
三つの方法を紹介します。最後のvisititems
を使う方法が一番良いでしょう。例に使うのはスイスのSINQという中性子実験施設でとった中性子小角散乱(SANS)データの入ったHDFファイルです。このファイルをHDFViewで見ると以下のような階層構造になっています。
ファイル直下のentry1というGroup
の下にSANS
やdata1
などのGroup
がある階層構造です。ポチポチ確認はできますが少し面倒そうなのがわかりますね。
Group
をディクショナリーとして扱う
h5pyではGroup
はPythonディクショナリーとして扱えるので、おなじみのkeys()
などが使えます。また、keys
なしでもforループで直下のオブジェクト名を渡してくれます。
hdfpath = 'sans2018n022960.hdf'
# f = h5py.File(hdfpath, 'r')だとf.close()が必須(忘れやすい)
# with構文はインデントブロックが終わると自動でファイルクローズしてくれるので便利
with h5py.File(hdfpath,'r') as f:
print('(1)----- 1st level -----')
# ファイルオブジェクトをイテレートするとファイル直下のオブジェクト名を返す
for k in f:
print(k)
print('(2)----- 2nd level, Group "entry1" -----')
# Groupもそのままforループに渡すと直下にいるオブジェクト名を返す
for k in f['entry1']:
print(k)
print('(3)----- 2nd level, Group "entry1", keys() -----')
# ディクショナリーのkeyを表示するようにしても(2)と同じ結果になる
for k in f['entry1'].keys():
print(k)
print('(4)----- 2nd level, Group "entry1", values() -----')
# entry1に入っている実体を表示
for k in f['entry1'].values():
print(k)
print('(5)----- 2nd level, Group "entry1", items() -----')
# entry1に入っているオブジェクト名と実体のtupleを表示
for k in f['entry1'].items():
print(k)
print('(6)----- 3rd level, Group "data1", items() -----')
# entry1/data1というGroupのオブジェクト名と実体のtuple
for k in f['entry1/data1'].items():
print(k)
print('(7)----- 3rd level, Dataset "comment" -----')
# commentはDatasetなので直下にオブジェクトを持たずkeysは使えない
for k in f['entry1/comment'].keys():
print(k)
(1)----- 1st level -----
entry1
(2)----- 2nd level, Group "entry1" -----
SANS
comment
data1
end_time
experiment_identifier
proposal_id
proposal_title
proposal_user
sample
start_time
title
user
(3)----- 2nd level, Group "entry1", keys() -----
SANS
comment
data1
end_time
experiment_identifier
proposal_id
proposal_title
proposal_user
sample
start_time
title
user
(4)----- 2nd level, Group "entry1", values() -----
<HDF5 group "/entry1/SANS" (16 members)>
<HDF5 dataset "comment": shape (1,), type "|S131">
<HDF5 group "/entry1/data1" (4 members)>
<HDF5 dataset "end_time": shape (1,), type "|S20">
<HDF5 dataset "experiment_identifier": shape (1,), type "|S8">
<HDF5 dataset "proposal_id": shape (1,), type "|S10">
<HDF5 dataset "proposal_title": shape (1,), type "|S8">
<HDF5 group "/entry1/proposal_user" (2 members)>
<HDF5 group "/entry1/sample" (21 members)>
<HDF5 dataset "start_time": shape (1,), type "|S20">
<HDF5 dataset "title": shape (1,), type "|S35">
<HDF5 group "/entry1/user" (1 members)>
(5)----- 2nd level, Group "entry1", items() -----
('SANS', <HDF5 group "/entry1/SANS" (16 members)>)
('comment', <HDF5 dataset "comment": shape (1,), type "|S131">)
('data1', <HDF5 group "/entry1/data1" (4 members)>)
('end_time', <HDF5 dataset "end_time": shape (1,), type "|S20">)
('experiment_identifier', <HDF5 dataset "experiment_identifier": shape (1,), type "|S8">)
('proposal_id', <HDF5 dataset "proposal_id": shape (1,), type "|S10">)
('proposal_title', <HDF5 dataset "proposal_title": shape (1,), type "|S8">)
('proposal_user', <HDF5 group "/entry1/proposal_user" (2 members)>)
('sample', <HDF5 group "/entry1/sample" (21 members)>)
('start_time', <HDF5 dataset "start_time": shape (1,), type "|S20">)
('title', <HDF5 dataset "title": shape (1,), type "|S35">)
('user', <HDF5 group "/entry1/user" (1 members)>)
(6)----- 3rd level, Group "data1", items() -----
('counts', <HDF5 dataset "counts": shape (128, 128), type "<i4">)
('detector_x', <HDF5 dataset "detector_x": shape (128,), type "<f4">)
('detector_y', <HDF5 dataset "detector_y": shape (128,), type "<f4">)
('lambda', <HDF5 dataset "lambda": shape (1,), type "<f4">)
(7)----- 3rd level, Dataset "comment" -----
---------------------------------------------------------------------------
AttributeError Traceback (most recent call last)
<ipython-input-43-8ab0e139ffa5> in <module>()
25
26 print('(7)----- 3rd level, Dataset "comment" -----')
---> 27 for k in f['entry1/comment'].keys():
28 print(k)
AttributeError: 'Dataset' object has no attribute 'keys'
しかし、この方法では直下のオブジェクトしか取得できないので再帰的に全Group
の中身を表示するにはかなり工夫が必要そうです。
visit()
Group
には再帰的にオブジェクトにアクセスするvisit()
というメソッドがあります。
http://docs.h5py.org/en/latest/high/group.html#Group.visit
このメソッドに引数一つの関数を渡すと、各オブジェクトのパスを引数としてその関数を渡してくれ、その関数が戻り値を返すまで全オブジェクトを再帰的に訪れます。つまり戻り値を返さないで単にオブジェクトへのパスをprint
するだけの関数を渡せば全オブジェクトのパスがずらっと表示されます。
def PrintAllObjects(name):
print(name)
# パスの一覧からも判断できるが
# 実体をprintするとDatasetかGroupかはっきり表示される
# print('\t', f[name])
with h5py.File(hdfpath,'r') as f:
f.visit(PrintAllObjects)
entry1
entry1/SANS
entry1/SANS/Dornier-VS
entry1/SANS/Dornier-VS/lambda
entry1/SANS/Dornier-VS/rotation_speed
entry1/SANS/Dornier-VS/tilt_angle
entry1/SANS/Dornier-VS/type
entry1/SANS/SINQ
entry1/SANS/SINQ/name
entry1/SANS/SINQ/type
entry1/SANS/attenuator
entry1/SANS/attenuator/selection
entry1/SANS/beam_stop
entry1/SANS/beam_stop/out_flag
entry1/SANS/beam_stop/x_null
entry1/SANS/beam_stop/x_position
entry1/SANS/beam_stop/y_null
entry1/SANS/beam_stop/y_position
entry1/SANS/collimator
entry1/SANS/collimator/length
entry1/SANS/detector
entry1/SANS/detector/beam_center_x
entry1/SANS/detector/beam_center_y
...
(以下省略)
visititems()
visit()
で当初の目標である階層構造の把握は可能です。しかし、Dataset
のパスだけで階層構造は把握できるので、Group
のパスは必要ありません。出力結果をもっとすっきりとさせるためにDataset
だけprint
できるとありがたいですね。visititems()
メソッドはオブジェクトのパスに加えてそのオブジェクトの実体も関数に引数として渡してくれます。つまりそのオブジェクトが``Datasetの場合だけ
print`するという関数を作ればもっとすっきりした出力になりそうです。
http://docs.h5py.org/en/latest/high/group.html#Group.visititems
def PrintOnlyDataset(name, obj):
if isinstance(obj, h5py.Dataset):
print(name)
# print('\t',obj)
with h5py.File(hdfpath,'r') as f:
f.visititems(PrintOnlyDataset)
entry1/SANS/Dornier-VS/lambda
entry1/SANS/Dornier-VS/rotation_speed
entry1/SANS/Dornier-VS/tilt_angle
entry1/SANS/Dornier-VS/type
entry1/SANS/SINQ/name
entry1/SANS/SINQ/type
entry1/SANS/attenuator/selection
entry1/SANS/beam_stop/out_flag
entry1/SANS/beam_stop/x_null
entry1/SANS/beam_stop/x_position
entry1/SANS/beam_stop/y_null
entry1/SANS/beam_stop/y_position
entry1/SANS/collimator/length
entry1/SANS/detector/beam_center_x
entry1/SANS/detector/beam_center_y
...
(以下省略)
できました。これで自分の作ったHDFファイルでなくても必要な情報がどこにあるか見当がつきます。コメントアウトを外してobj
もprint
すれば
<HDF5 dataset "z_position": shape (1,), type "<f4">
のようにnumpy arrayのshape
やint
かfloat
かbyte文字列かを判断できる追加情報も得られます。
データの読み出し
データの読み出しは基本事項なのですでに前述したような日本語記事がありますが、データの種類別の言及はないので少し詳しく見ていきます。今回使っているHDFファイルには装置の設定値などのスカラー値、測定した試料の名前やコメントなどの文字列、二次元検出器のカウント数の2次元データの三種類が含まれます。HDFファイル内のこの三種類のオブジェクトをどう読み出したらいいか以下に示します。
with h5py.File(hdfpath,'r') as f:
print('----- entry1/sample/z_position (scalar value) -----')
# HDF内のDatasetを指定するだけではDatasetインスタンスが返ってくるだけなのでダメ
print('raw dataset:\t', f['entry1/sample/z_position'])
# valueをつけるとnumpy arrayとして中身を受け取れる
print('with value:\t', f['entry1/sample/z_position'].value)
print('object type:\t', type(f['entry1/sample/z_position'].value))
# valueの代わりにnumpy arrayのテクニックっぽい[()]でも受け取れる
print('with [()]:\t', f['entry1/sample/z_position'][()])
print('object type:\t', type(f['entry1/sample/z_position'][()]))
# スカラー値として受け取るには要素1つの1次元numpy arrayのインデックスを指定
print('with value[0]:\t', f['entry1/sample/z_position'].value[0])
# インデックスを指定する際はvalueを省略できる
print('with [0]:\t', f['entry1/sample/z_position'][0])
print('----- entry1/comment (string) -----')
print('raw dataset:\t', f['entry1/comment'])
# 文字列も要素1つの1次元numpy arrayとして受け取る
print('with value:\t', f['entry1/comment'].value)
print('object type:\t', type(f['entry1/comment'].value))
# 文字列はbyte文字列になっている
print('with value[0]:\t', f['entry1/comment'].value[0])
print('object type:\t', type(f['entry1/comment'].value[0]))
# decodeすると普通の文字列になる
print('decoded:\t', f['entry1/comment'].value[0].decode('utf-8'))
print('----- entry1/SANS/detector/counts (matrix) -----')
print('raw dataset:\t', f['entry1/SANS/detector/counts'])
print('with value:\t', f['entry1/SANS/detector/counts'].value)
print('object type:\t', type(f['entry1/SANS/detector/counts'].value))
# 2次元numpy arrayの場合でもvalueの省略は可能。この例は一行目を指定。一列目なら[:, 0]。
print('with [0]:\t', f['entry1/SANS/detector/counts'][0])
----- entry1/sample/z_position (scalar value) -----
raw dataset: <HDF5 dataset "z_position": shape (1,), type "<f4">
with value: [ 41.19506836]
object type: <class 'numpy.ndarray'>
with [()]: [ 41.19506836]
object type: <class 'numpy.ndarray'>
with value[0]: 41.1951
with [0]: 41.1951
----- entry1/comment (string) -----
raw dataset: <HDF5 dataset "comment": shape (1,), type "|S131">
with value: [ b'sample temp. apprx. 50 C']
object type: <class 'numpy.ndarray'>
with value[0]: b'sample temp. apprx. 50 C'
object type: <class 'numpy.bytes_'>
decoded: sample temp. apprx. 50 C
----- entry1/SANS/detector/counts (matrix) -----
raw dataset: <HDF5 dataset "counts": shape (128, 128), type "<i4">
with value: [[0 0 1 ..., 2 1 0]
[0 0 1 ..., 2 0 0]
[0 1 2 ..., 3 2 0]
...,
[0 7 6 ..., 2 0 0]
[0 0 8 ..., 3 1 0]
[0 0 0 ..., 0 0 0]]
object type: <class 'numpy.ndarray'>
with [0]: [0 0 1 1 2 1 3 3 0 0 1 3 2 8 6 6 0 1 1 2 2 3 4 5 1 1 3 3 5 2 2 6 2 3 0 4 3
4 6 3 6 5 2 4 4 5 2 2 0 5 3 6 5 2 4 2 1 5 2 1 3 3 1 1 6 3 2 4 2 3 4 2 3 1
3 4 1 3 2 6 3 1 2 3 4 3 4 3 1 0 1 5 1 1 3 0 2 6 2 1 0 2 2 0 1 3 4 0 1 0 1
2 1 1 2 0 1 2 2 1 0 3 1 1 3 2 1 0]
要点は以下の通りです。
- 数字、文字列、行列に関わらずデータは
value
をつけるとnumpy arrayとして受け取れる - valueの代わりにnumpyのテクニックである[()]を使ってもよい
- スカラー値、文字列は要素1つの1次元numpy arrayなのでインデックス[0]をつけて受け取る
- 文字列はbyte文字列として格納されているのでdecodeする必要がある
- numpy arrayのインデックスをつける場合はvalueを省略できる
必要な部分を抽出してDataFrameにする
私の手元にある測定条件などのメタ情報と二次元検出器のデータが含まれる大量のHDFファイルから必要な情報だけを取り出して、DataFrameにすることで簡易的な測定条件データベースを作ってみます。
def MakeMeasurementList(hdfpaths, datasets, save=False, output='df.h5'):
'''
測定条件をまとめたDataFrameを作成する。
hdfpaths: HDFファイルへのパスのリスト
datasets: HDFファイルから抽出したい情報パスのディクショナリー。
keyはDataFrameのコラム名。
'''
# このheaderというディクショナリーにdatasetsで指定した情報をHDFから読み取り追加
header = {'path':hdfpaths}
for hdfpath in hdfpaths:
with h5py.File(hdfpath,'r') as f:
for colname, dataset in datasets.items():
# 最初のループ
if not colname in header :
# 最初のループの段階ではheaderには先に定義した'path'しかない
# 新しいkeyとリストにいれた文字列、数字、行列をvalueとして格納
if colname == 'total counts':
header[colname] = [f[dataset].value.sum()]
elif colname == 'counts':
header[colname] = [f[dataset].value]
else:
# 最初に文字列のデコードにトライ、ダメなら普通の数字としてトライ
# 値がなければnanを追加
try:
header[colname] = [f[dataset].value[0].decode('utf-8')]
except AttributeError:
# ここはnumpy arrayをlistに変換
header[colname] = list(f[dataset].value)
except KeyError:
header[colname] = [np.nan]
# 初回以降のループはディクショナリーの該当keyに入ったリストに値を追加していく
else :
if colname == 'total counts':
header[colname].append(f[dataset].value.sum())
elif colname == 'counts':
header[colname].append(f[dataset].value)
else:
try:
header[colname].append(f[dataset].value[0].decode('utf-8'))
except AttributeError:
header[colname].append(f[dataset].value[0])
except KeyError:
header[colname].append(np.nan)
# ディクショナリーをDataFrameに変換
df = pd.DataFrame(header)
# saveオプションがTrueならHDFファイルとして保存
if save:
df.to_hdf(output, 'df')
return df
from pathlib import Path # ファイルパスを便利に扱える標準パッケージ
hdfpaths = sorted(Path('./data').glob('**/*.hdf'))
# print('No. of HDF files:', len(hdfpaths)) #確認用
# HDF5ファイルの抽出したい値の場所を、その値を格納する列の名前をkeyにしたdictionaryに
# ここだけは手作業が必要
instrument = 'SANS'
datasets = {'lambda':f'entry1/{instrument}/Dornier-VS/lambda',
'att':f'entry1/{instrument}/attenuator/selection',
'bsx':f'entry1/{instrument}/beam_stop/x_position',
'bsy':f'entry1/{instrument}/beam_stop/y_position',
'coll':f'entry1/{instrument}/collimator/length',
'bcx':f'entry1/{instrument}/detector/beam_center_x',
'bcy':f'entry1/{instrument}/detector/beam_center_y',
'time':f'entry1/{instrument}/detector/counting_time',
'total counts':f'entry1/{instrument}/detector/counts',
# 'counts':f'entry1/{instrument}/detector/counts',
'SD':f'entry1/{instrument}/detector/x_position',
'mon1':f'entry1/{instrument}/monitor1/counts',
'mon2':f'entry1/{instrument}/monitor2/counts',
'comment':'entry1/comment',
'end_time':'entry1/end_time',
'mf':'entry1/sample/magnetic_field',
'sample_name':'entry1/sample/name',
'sample_z':'entry1/sample/z_position',
'flipper':f'entry1/{instrument}/flipper/state',
'polarizer':f'entry1/{instrument}/polarizer/state',
'start_time':'entry1/start_time'}
df = MakeMeasurementList(hdfpaths, datasets)
# print(f'No. of scans in df: {len(df)}') #確認用
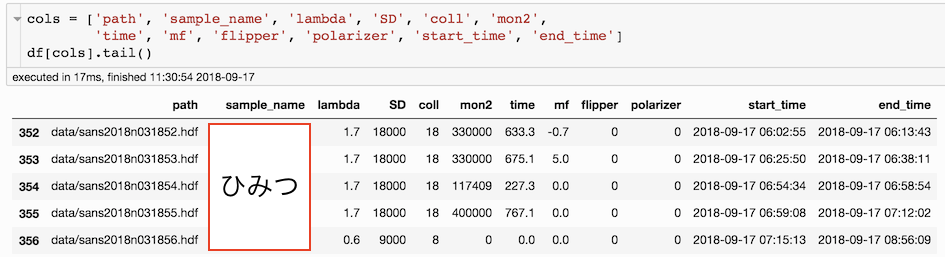
できました。あとは大量の実験データの測定条件管理と解析にpandas.DataFrameを活用する - Qiitaのように好きに活用するだけです。