はじめに
「Deep learningをやりたいけど、PythonがMATLAB、どっちがいいの?」
「画像処理をやりたいけど(略)」
「自然言語処理をやりたいけど(略)」
「ゲームを作りたいけど(略)」
・・・
プログラミングでなにかしたい!と思ったときにはこんなことをまず考えますよね?
諸般の事情でMATLAB以外のプログラミング言語を使わざるを得ないケースや他の言語に目移りするケースもしばしば。
諸般の事情によりSimulink内でC言語を使わざるを得ないケース*というのが大人にはあります。
(MATLAB/Simulink Advent Calendar 2021| 1日目
最近はPythonでもMATLABでもGPU処理のアドオンやライブラリが出てきて、以前よりもGPUの活用がしやすくなっています。
(MATLAB/Simulink Advent Calendar 2021| 2日目 by @tommyecguitarさん](https://qiita.com/tommyecguitar)
本記事では上述の疑問を解決してくれるシステムを紹介します。
(もちろん、**MATLABが最強!!**ですけど)
CoQAS (Comparative Question Answering System)
「どのプログラミング言語を使ったらいいかわからない!」
そんな疑問にお答えするのがCoQAS (Comparative Question Answering System)1です.
CoQASは、「XとYのどちらが良いか?(Which is better, X or Y?)」のような比較質問(Comparative question)に回答してくれます。
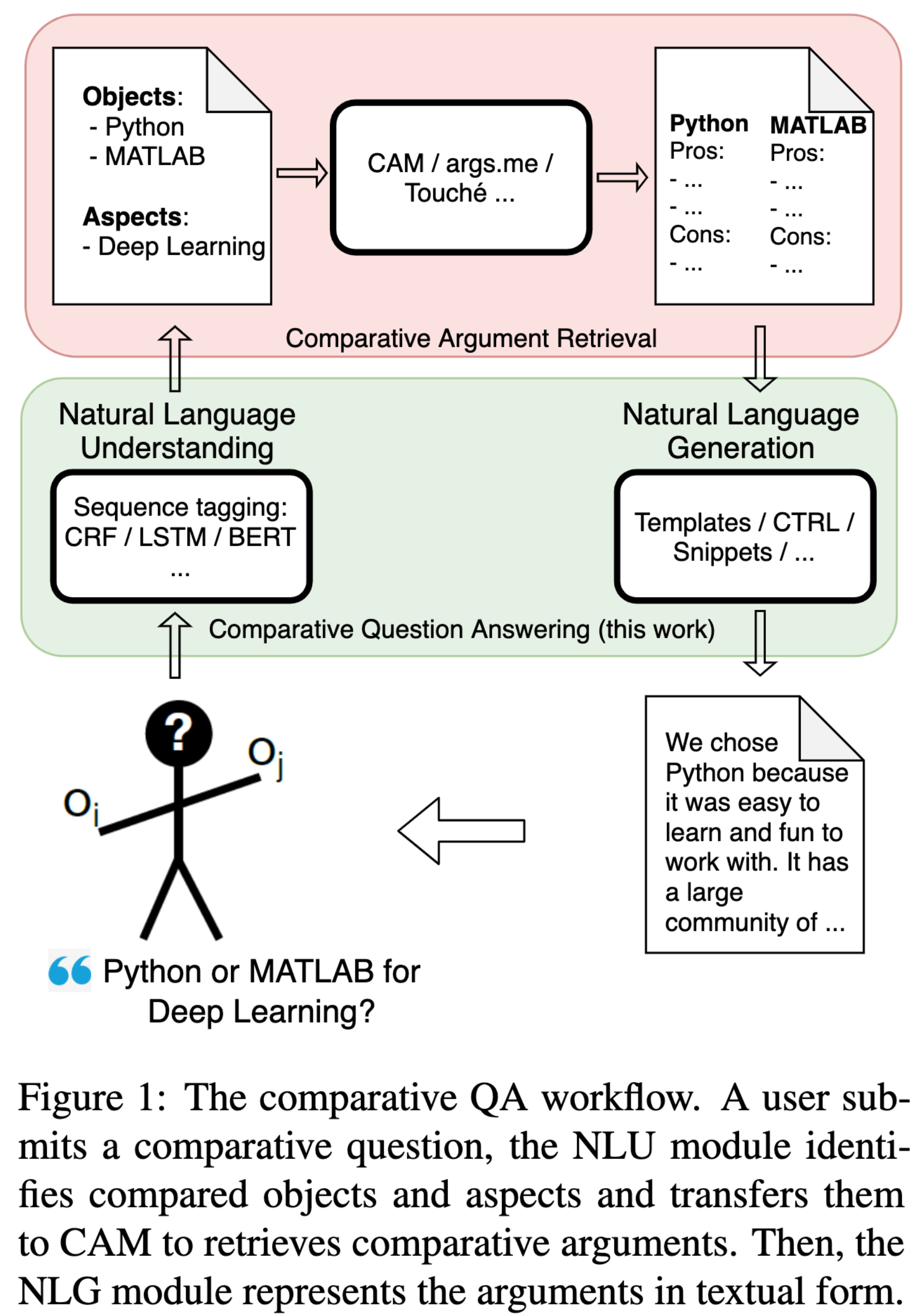
(図は論文1より引用)
CoQASは以下の3つのコンポーネントから構成されます。
- 自然言語処理理解モジュール
- Comparative Argumentative Machine
- 自然言語生成モジュール
自然言語処理モジュール (Natural language understanding module, NTU module)
ユーザが入力した文(user textual query)から比較対象(object)、観点(aspect)、述語(predicate)を特定します。
例えば、Which is better for deep Learning: Python or MATLAB? という文では、object は Python と MATLAB、aspect は deep learning、predicate は better です。
NTU module では、object と aspect を特定するタスクを、系列ラベリング(sequence labeling)とみなし、入力である質問文に含まれるトークンを分類します。
論文では、BiLSTM2、BiLSTM-CRF、ELMO3、BERT4、RoBERTa5 による性能を比較検討しています。
後述の CoQASのonline demo では、その中で性能が最高だったRoBERTaが採用されています。
Comparative Argumentative Machine (CAM)
2つのモノ(object)を比較するシステムです6 7。
与えられたモノに関する情報をウェブページから抽出、順位付けして出力します。
Live demoをオンラインで利用することができます。
自然言語生成モジュール (Natural language generation module, NTG module)
CAMの出力をもとに、簡潔で意味の通った答えを生成します。
論文では、4つの手法を比較検討しています。
- 事前学習モデルに基づく手法: CTRLモデル8を使って文を生成する
- 文検索に基づく手法: CAMによる検索結果の上位3つの文を出力する
- 文書検索に基づく手法: CAMの出力で最上位の文を含む文書における周りの3つの文を出力する
- テンプレートに基づく手法: CAMの結果から objects、 aspects、 predicate を抽出して事前に設定したテンプレート(I would prefer to use Object1 because it is Predicate1 and Predicate 2...)に当てはめて出力する。
後述の CoQASのonline demo では、事前学習モデルに基づく手法(CTRL)、文検索に基づく手法(CAM)、文書検索に基づく手法(Snippet)が採用されています。
Online demo
プロジェクトページから、 online demo が利用できます。
使い方はかんたん。
- ブラウザに表示されるテキストボックスに質問を書く
- 言語生成モジュール(NTG module)を決定する
- Answerボタンを押す
では、最初に挙げたいくつかの疑問を解決しましょう。
今回は Python と MATLAB を比較対象にします。
- 生成結果は毎回変化するので、複数の結果を載せています
- 今回は、論文で文の流暢さ(Answer fluency)が高いとされていたCTRLを使用しました。
「Deep learningをやりたいけど、PythonがMATLAB、どっちがいいの?」
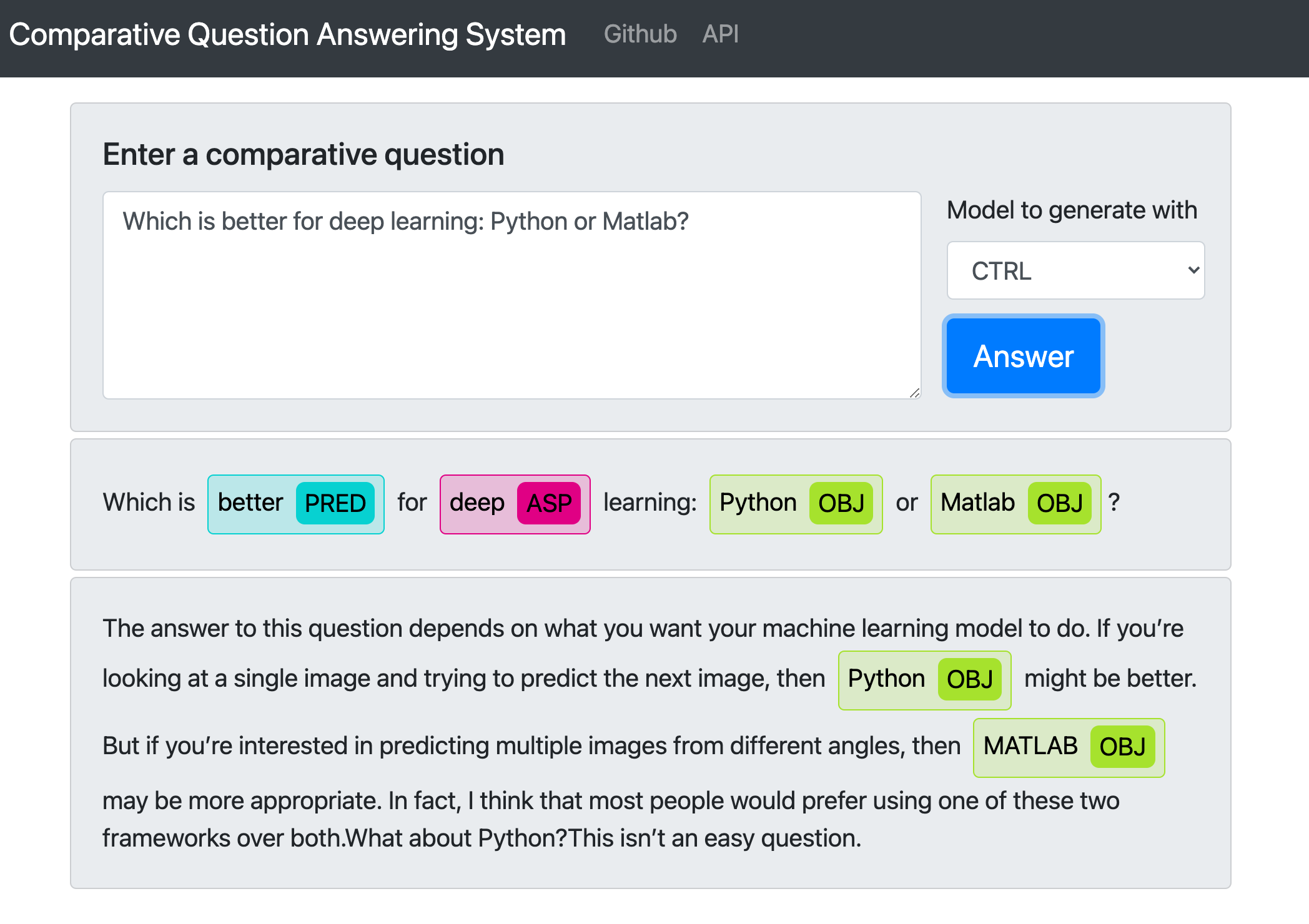
「画像処理をやりたいけど、PythonがMATLAB、どっちがいいの?」
「自然言語処理をやりたいけど、PythonがMATLAB、どっちがいいの?」
「ゲームを作りたいけど、PythonがMATLAB、どっちがいいの?」
どっちがいいか、答えてないやん!!
さいごに
2つのモノを比較する疑問に対して回答するCoQASをざっと紹介しました。
CoQASの結果は(おそらく)ウェブページの検索数に基づいています。
(詳細はCAMの論文6にありますが、ちゃんと読めてません・・・)
なので、**MATLAB が Python よりも良い!**という記事を増やせば、同様のウェブページベースのシステムであれば MATLAB を推薦するようになるはずです。
(最初に書いたように、「もちろん、**MATLABが最強!!**です」というスタンスに基づいています)
MATLABの記事増加 → CoQASがMATLABを推薦 → MATLAB利用者増加→MATLABの記事増加 ... というループが回るようになるといいなあ。
コメント
- [実装コード](https://github.com/skoltech-nlp/coqas)が Python (Jupyter Notebook) だったので、ちょっと残念でした。
- Webページベースなので、質問に答えない場合が多い。詳細は不明だが、結果が得られないことがよくある。
- タイトル(Which Is Better for Deep Learning: Python or MATLAB? Answering Comparative Questions in Natural Language)につられて勢いでAdvent calendarに登録してしまいましたが、QA関連の論文は読んだことがなかったので良い勉強になりました。
- 自然言語処理系の学会では、このようなアプリケーションを提案する研究を発表するセッション(System Demonstrations)があります。理論はもちろん大事ですが、実装して実際に触れる形になっていると個人的にポイント高いです。MATLABでもアプリケーション作成が簡単にできるので、活用していきたいですね。
-
Chekalina, Viktoriia, Alexander Bondarenko, Chris Biemann, Meriem Beloucif, Varvara Logacheva, and Alexander Panchenko. 2021. “Which Is Better for Deep Learning: Python or MATLAB? Answering Comparative Questions in Natural Language.” In Proceedings of the 16th Conference of the European Chapter of the Association for Computational Linguistics: System Demonstrations, 302–11. Online: Association for Computational Linguistics. ↩ ↩2
-
Arora, Jatin, Sumit Agrawal, Pawan Goyal, and Sayan Pathak. 2017. “Extracting Entities of Interest from Comparative Product Reviews.” In Proceedings of the 2017 ACM on Conference on Information and Knowledge Management, 1975–78. CIKM ’17. New York, NY, USA: Association for Computing Machinery. ↩
-
Peters, Matthew, Mark Neumann, Mohit Iyyer, Matt Gardner, Christopher Clark, Kenton Lee, and Luke Zettlemoyer. 2018. “Deep Contextualized Word Representations.” In Proceedings of the 2018 Conference of the North American Chapter of the Association for Computational Linguistics: Human Language Technologies, Volume 1 (Long Papers). Stroudsburg, PA, USA: Association for Computational Linguistics. https://doi.org/10.18653/v1/n18-1202. ↩
-
Devlin, Jacob, Ming-Wei Chang, Kenton Lee, and Kristina Toutanova. 2019. “BERT: Pre-Training of Deep Bidirectional Transformers for Language Understanding.” In Proceedings of the 2019 Conference of the North American Chapter of the Association for Computational Linguistics: Human Language Technologies, Volume 1 (Long and Short Papers), 4171–86. Minneapolis, Minnesota: Association for Computational Linguistics. ↩
-
Liu, Yinhan, Myle Ott, Naman Goyal, Jingfei Du, Mandar Joshi, Danqi Chen, Omer Levy, Mike Lewis, Luke Zettlemoyer, and Veselin Stoyanov. 2019. “RoBERTa: A Robustly Optimized BERT Pretraining Approach.” arXiv [cs.CL]. arXiv. http://arxiv.org/abs/1907.11692. ↩
-
Schildwächter, Matthias, Alexander Bondarenko, Julian Zenker, Matthias Hagen, Chris Biemann, and Alexander Panchenko. 2019. “Answering Comparative Questions: Better than Ten-Blue-Links?” In Proceedings of the 2019 Conference on Human Information Interaction and Retrieval, 361–65. CHIIR ’19. New York, NY, USA: Association for Computing Machinery. ↩ ↩2
-
Keskar, Nitish Shirish, Bryan McCann, Lav R. Varshney, Caiming Xiong, and Richard Socher. 2019. “CTRL: A Conditional Transformer Language Model for Controllable Generation.” arXiv [cs.CL]. arXiv. http://arxiv.org/abs/1909.05858. ↩