semantic segmentationで有名なSegNet。ネットワーク見る感じシンプルだけど何が他と違うのかまとめてみた。
もうちょい実験したらgithubにも乗っけようと思う
https://github.com/yokosyun/SegNet
ネットワーク
Encoder-DecoderだけのシンプルなネットワークでDeconvolutionを使わなくてもUnpooling時にPooling Indicesを使えばそこそこ精度も出て、メモリに優しいよっといった設計。
Max-pooling indies vs sum
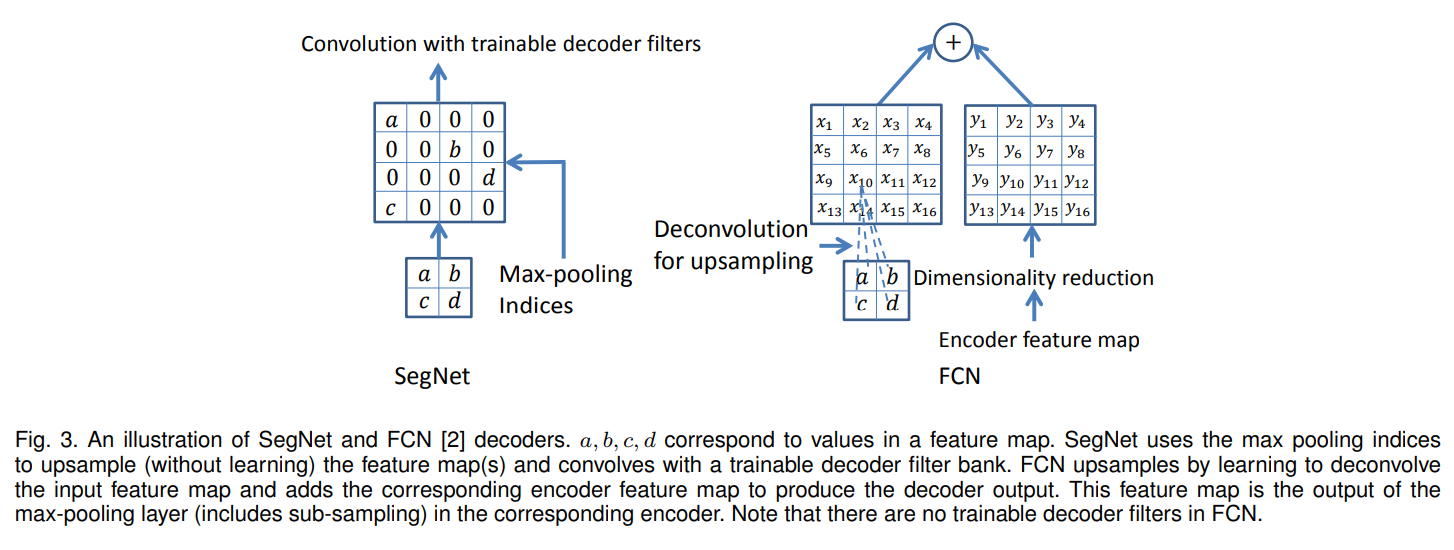左側がSegNetで提案するMax-Pooling Indicesを使ったunpooling。Max Poolingする時に最大値の位置をIndicesとして記憶することでunpooling時に元の位置に特徴量を戻せる。
右側がFCNで2倍にUpsamplingした結果とEncoderの特徴量を足す事で全体感を掴みつつ、細かい情報にアクセス出来るようになっている。
比較
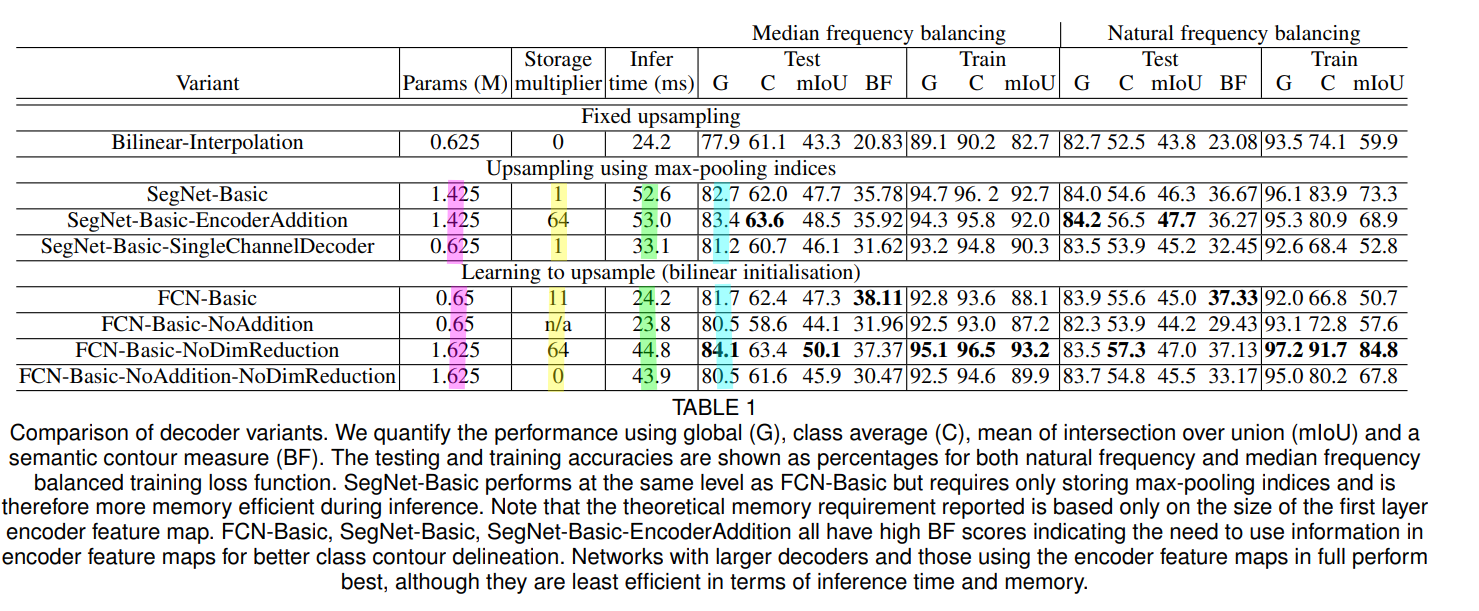 一つずつ比較を解説していこうと思うSegNet-Basic vs SegNet-Basic-EncoderAddition
encoderの結果をdecoderに足すと精度が上がるが、メモリの使用量もあがる。SegNet-Basic vs SegNet-Basic-SingleChannelDecoder
Decorderのfilter数を1にする事でPaeameterの数が減り、計算速度も早くなるが、精度が落ちるFCN-Basic vs FCN-Basic-NoAddition
DecoderにEncoderの結果をたさないと精度が落ちるが、使用するメモリ量が減る。
FCN-Basic vs FCN-Basic-NoDimReduction
channel数を小さくせず、大きなネットワークを使う事で精度はあがるが、parameter数、メモリー使用量、計算時間が上昇するFCN-Basic-NoDimReduction vs FCN-Basic-NoAddition-NoDimReduction
DecoderにEncoderの結果をたさないと精度が落ちるが、使用するメモリ量が減る。結論
SegNet-Basicはメモリの使用量を小さく出来て、さらに結果はFCN-Basicと同じくらいの精度が出るので、Pooling Indiceiesという方法はそこそこ効率的だと言える。もちろん精度を上げたいのであれば、DecoderにEncoderの結果を足し合わせたりするのもありだけどメモリの使用量増えますよっていう論文でした。