SECOND: Sparsely Embedded Convolutional Detection
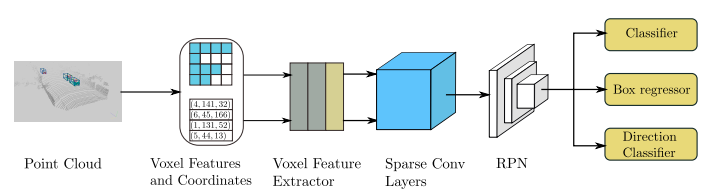VoxelNetとほとんど同じなSECOND。
違う点だけを見ていきたいと思う。
- Feature Learning Network => Voxel Feature and Coordinate + Voxel Feature Extractor
- Convolutional Middle Layers => Sparse Conv Layer
- Region Proposal Network => Region Proposal Network
新規性
Sparse Convolutional Middle Extractor
VoxelNetではConvolutional Middle Layersと呼ばれていた、3Dを2Dに畳み込む処理。 Sparse Convolutionを採用する事で高速化。*Sparse Convolutionの詳細は別記事で追記
Region Proposal Network
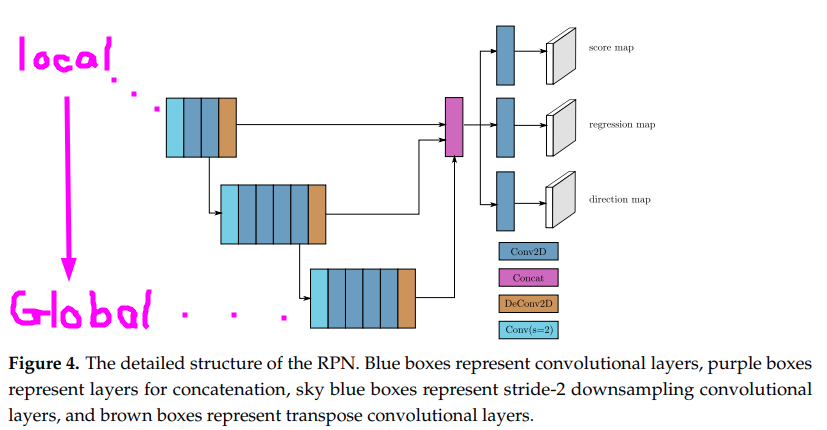2D Object Detectionの話だから軽く流すが、Multi ResolutionでLocalとGlobalの特徴量を得られる構成にした。
Sine-Error Loss for Angle Regression
角度のlossを計算する時0とPIで同じ角度を示すのに、値としてジャンプしてしまうのでsinやcosで解決しましょうっていう、よくあるやつ。