Unity ML-Agents(v0.11.0)をDocker上で動作させてみました。
UnityやUnity ML-Agentsの環境構築などは下記をご参考ください。
Macでhomebrewを使ってUnityをインストールする(Unity Hub、日本語化対応)
https://qiita.com/kai_kou/items/445e614fb71f2204e033
MacでUnity ML-Agentsの環境を構築する(v0.11.0対応) - Qiita
https://qiita.com/kai_kou/items/0d40157cbc303fb10c22
手順
v0.10.1まではDockerでの学習方法についてドキュメントがあったのですが、v0.11.0の時点でドキュメントが削除されています。情報としては若干古くなりますが、v0.11.0でもこちらを参考にして動作させることができます。
ml-agents/Using-Docker.md at 0.10.1 · Unity-Technologies/ml-agents
https://github.com/Unity-Technologies/ml-agents/blob/0.10.1/docs/Using-Docker.md
Dockerのインストール
Dockerがインストールされていない場合、インストールします。
> brew cask install docker
(略)
> docker --version
Docker version 19.03.4, build 9013bf5
※Dockerを初回起動すると初期設定のためにパスワード入力が求められます。
UnityにLinuxビルドサポートコンポーネントを追加する
Unity Hubを利用してUnityにLinuxビルドサポートコンポーネントを追加します。
Unityのバージョンは2019.2.10f1
を利用しています。
Unity Hubがインストールされていない場合は下記をご参考ください。
Macでhomebrewを使ってUnityをインストールする(Unity Hub、日本語化対応)
https://qiita.com/kai_kou/items/445e614fb71f2204e033
- Unity Hubアプリを起動する
- [インストール] > [Unityの利用するアプリ]右側にある[︙]をクリックして[モジュールを加える]を選択する

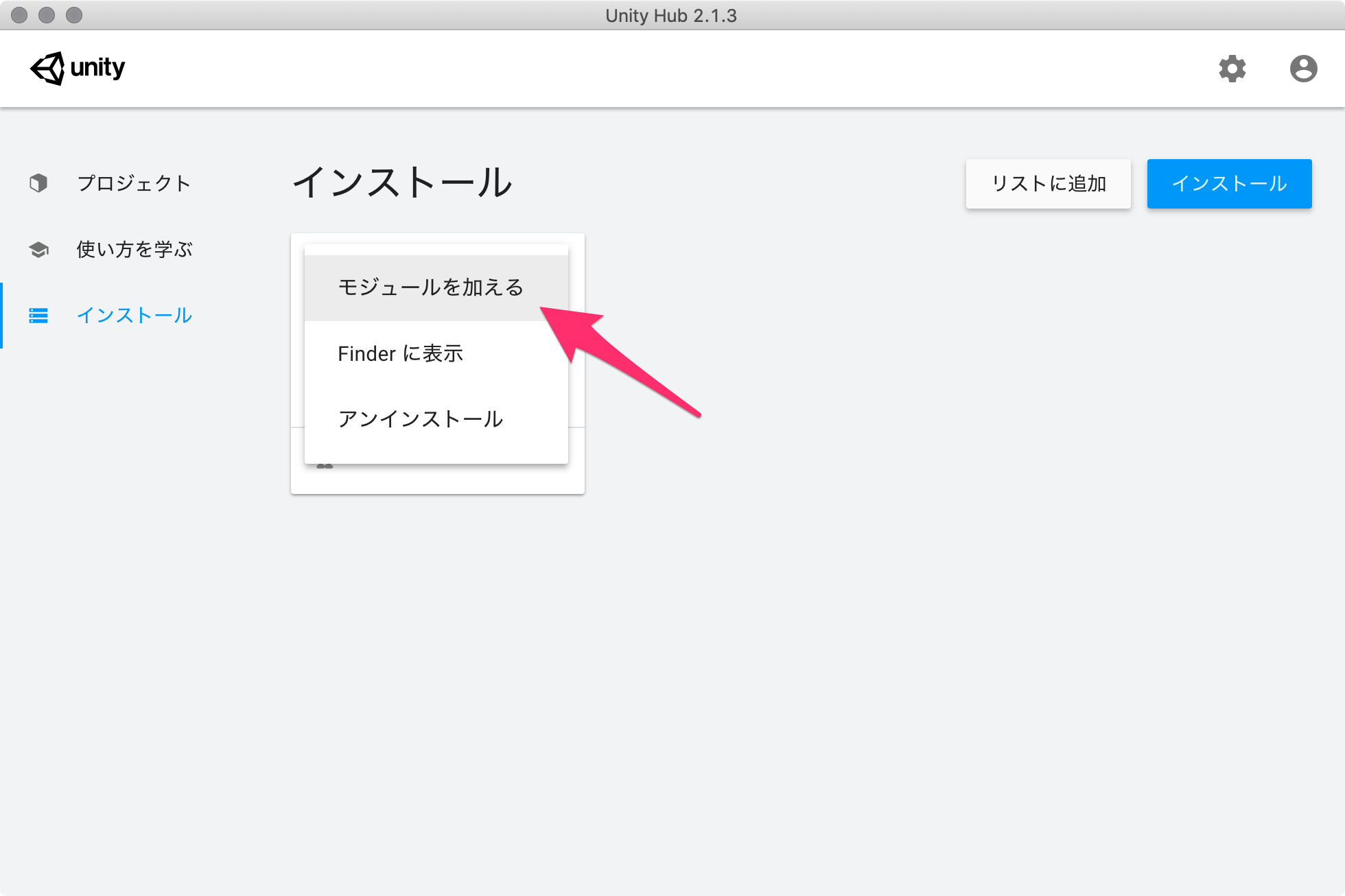
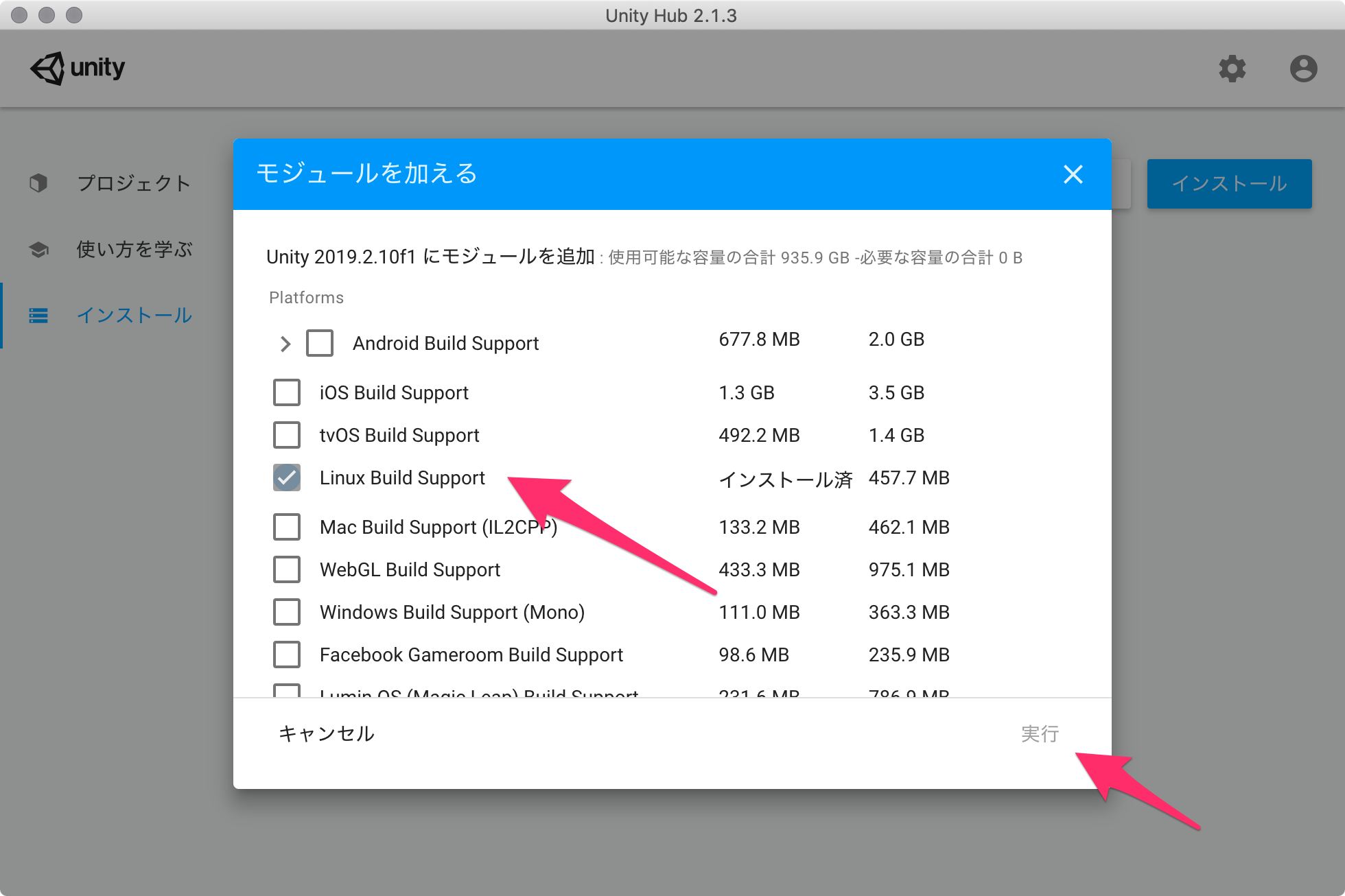
学習用のUnityアプリをダウンロードしてビルドする
ML-Agentsリポジトリに含まれているサンプルを学習できるようにします。
ML-Agentsリポジトリをダウンロード
適当なディレクトリにリポジトリをダウンロードする。
> mkdir 適当なディレクトリ
> cd 適当なディレクトリ
> git clone https://github.com/Unity-Technologies/ml-agents.git
Unityアプリからサンプルプロジェクトを開く
Unity Hubでアプリを立ち上げます。
ML-Agentsを利用するにはUnityのバージョン2017.4
以上が必要となります。今回は2019.2.10f1
を利用しました。
アプリが立ち上がったら「開く」ボタンから任意のディレクトリ/ml-agents/UnitySDK
フォルダを選択します。

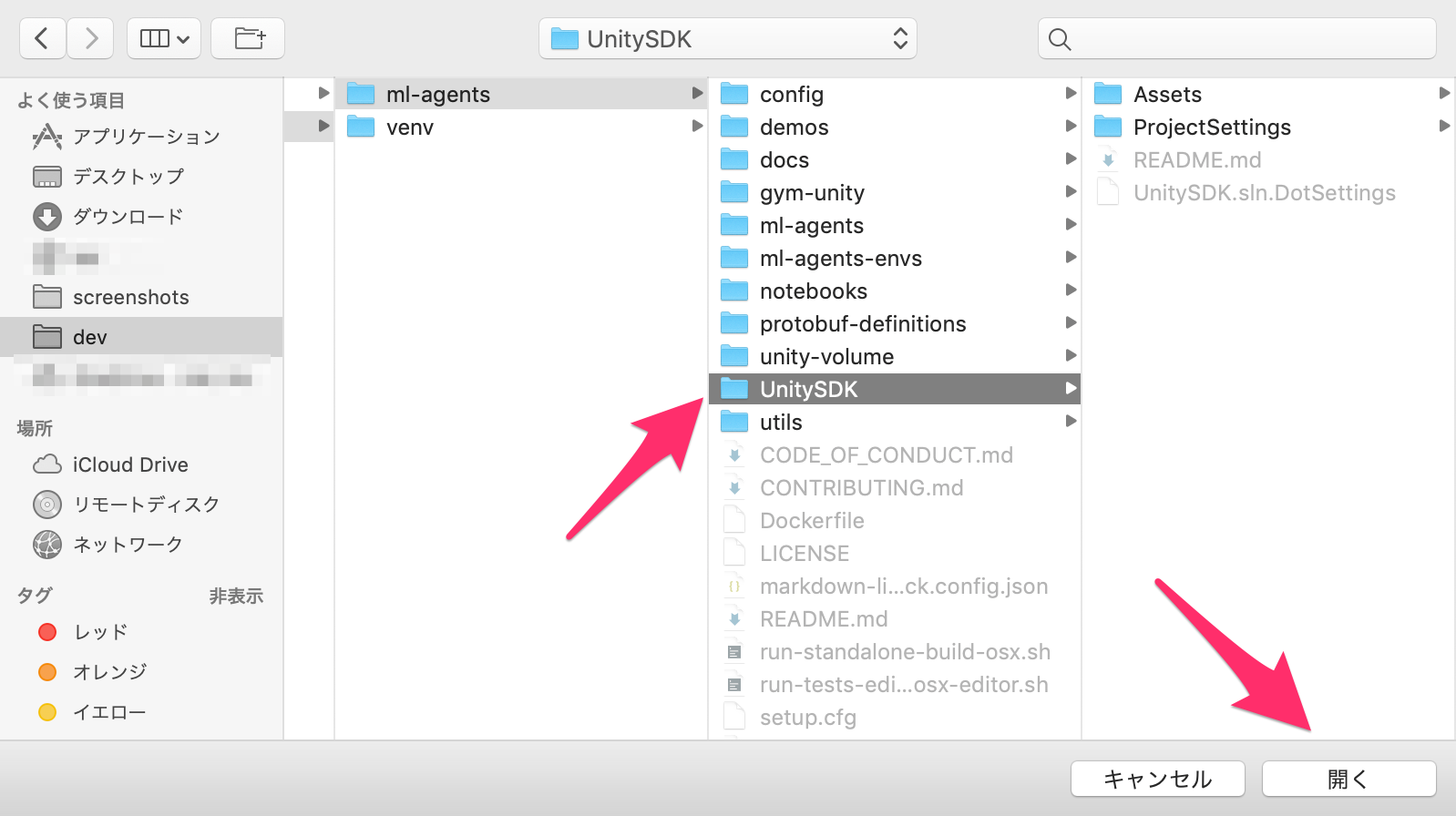
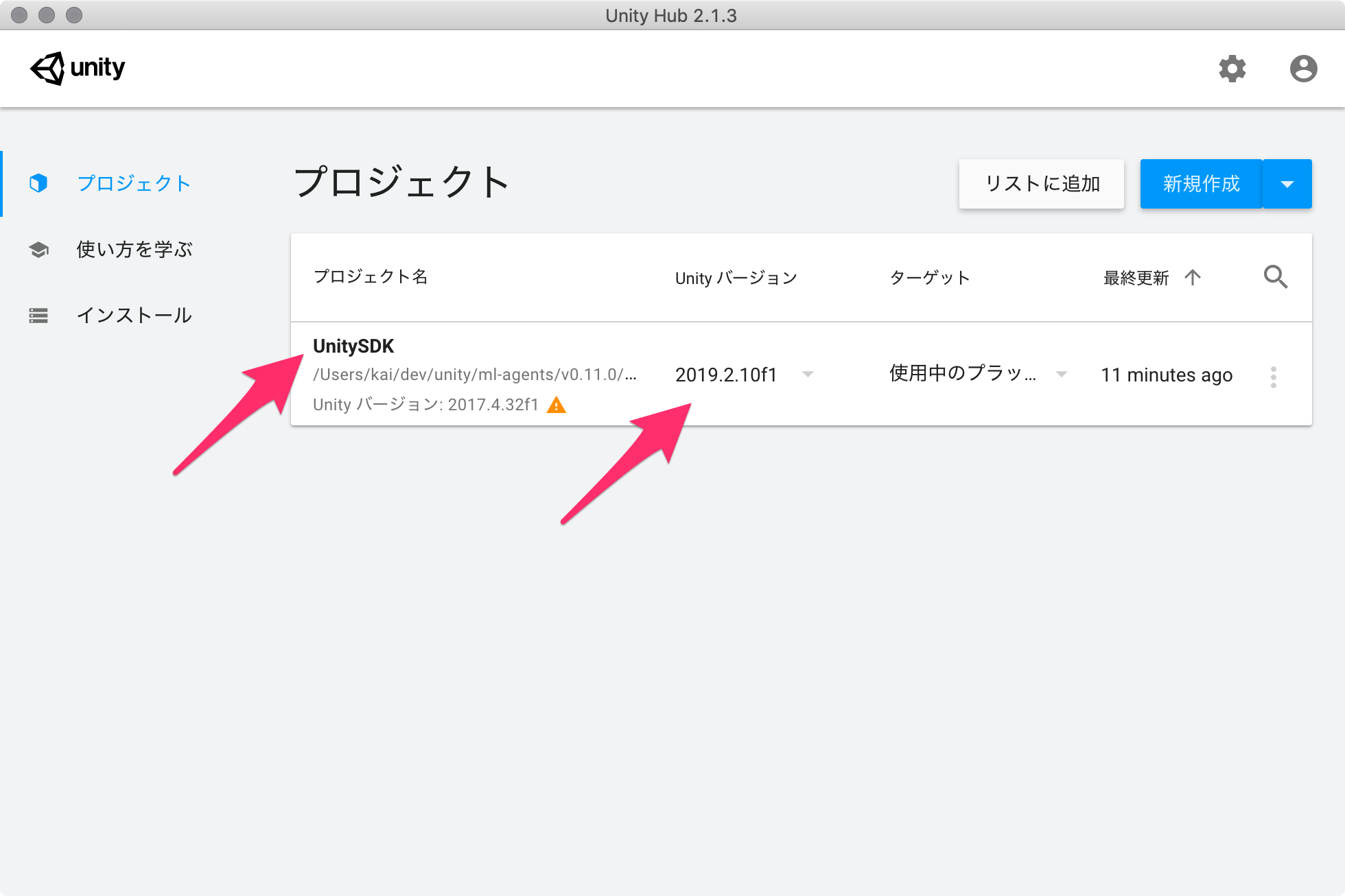
Unityエディタのバージョンによっては、アップグレードするかの確認ダイアログが立ち上がります。

「確認」ボタンをクリックして進めます。アップグレード処理に少し時間がかかります。

起動しました。
今回は、サンプルにある[3DBall]Scenesを利用します。
- Unityアプリの下パネルにある[Project]タブから以下のフォルダまで開く
- [Assets] > [ML-Agents] > [Examples] > [3DBall] > [Scenes]
- 開いたら、[3DBall]ファイルがあるので、ダブルクリックして開く

Scenes(シーン)の設定
ML-Agentsで学習させるための設定です。
- Unityアプリの[Edit]メニューから[Project Settings]を開く

- [Inspector]パネルで以下の設定を確認する
- [Resolution and Presentation]の[Run In Background]がチェックされている
- [Display Resolution Dialog]がDisableになっている

ビルド設定
- Unityアプリの[File]メニューから[Build Settings]を選択して[Build Settings]ダイアログを開く
- [Add Open Scenes]をクリックする
- [Scenes In Build]で[ML-Agents/Examples/3DBall/Scenes/3DBall]にチェックを入れる
- [Platform]で
PC, Mac & Linux Standalone
が選択されていることを確認する - [Target Platform]を
Linux
に変更する - [Architecture]を
x86_64
に変更する - [Server Build]にチェックを入れる
- [Build]ボタンをクリックする
- ファイル保存ダイアログで以下を指定してビルドを開始する
- ファイル名: 3DBall
- フォルダ名: 任意のディレクトリ/ml-agents/unity-volume
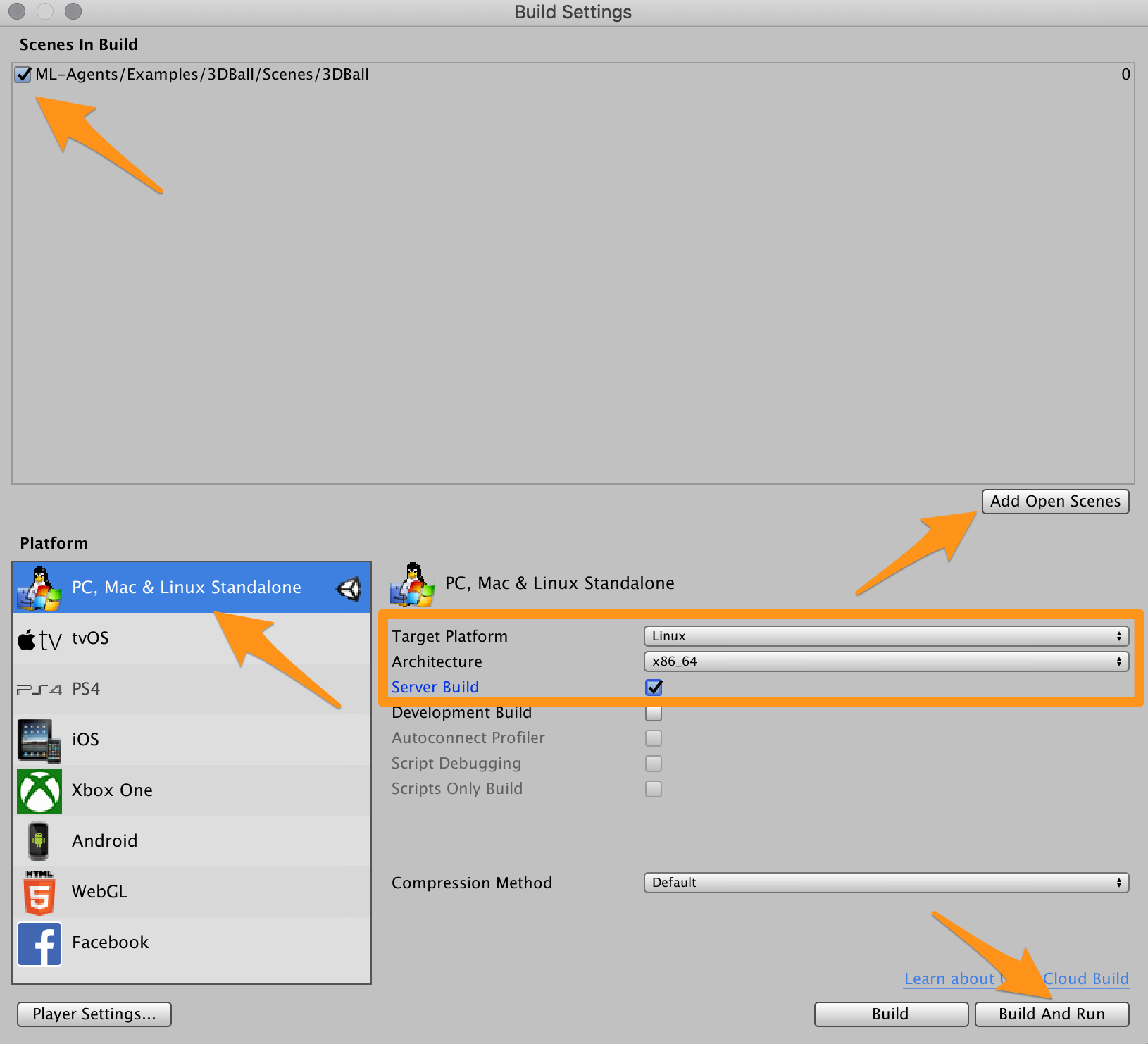
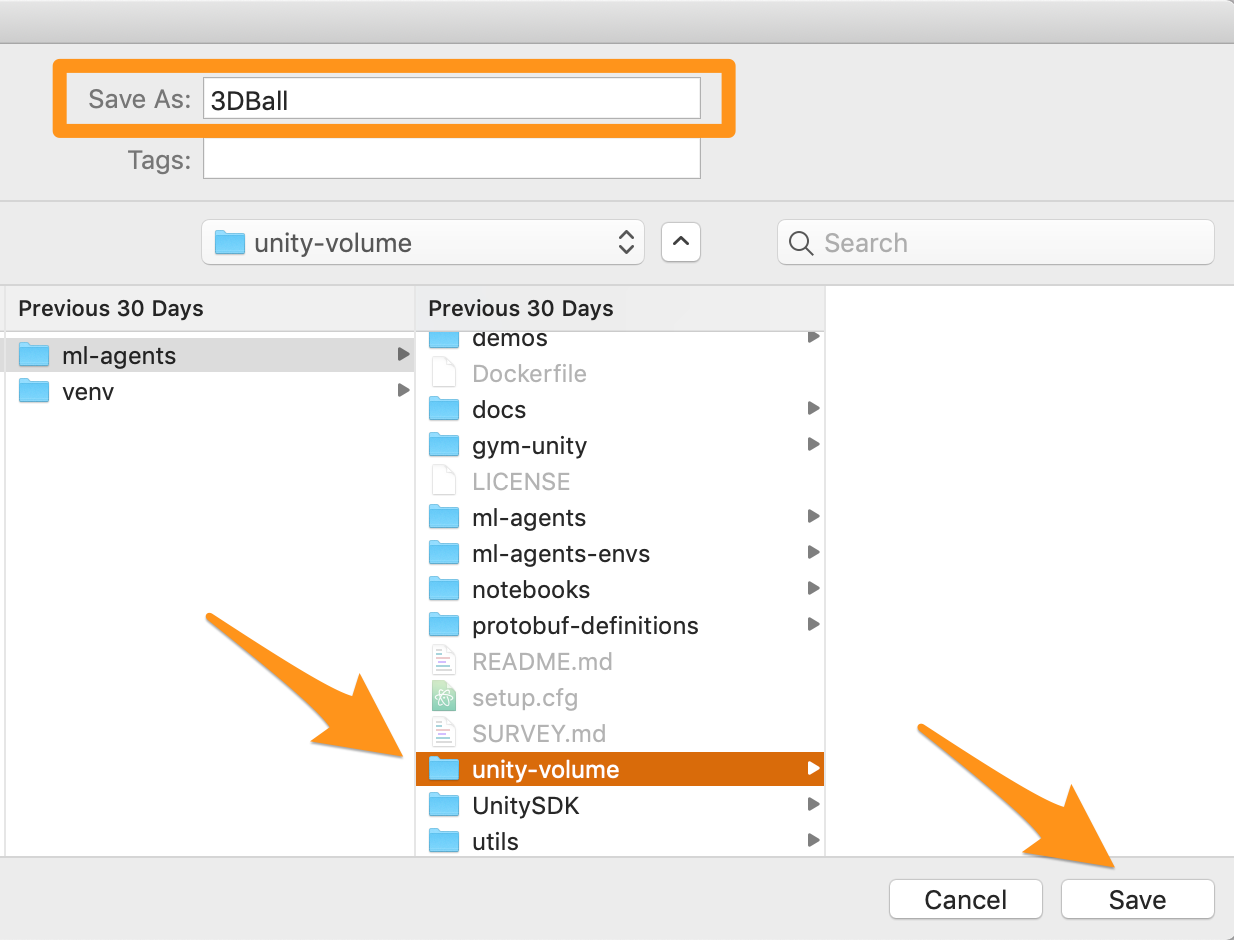
すると、unity-volume
に以下フォルダ・ファイルが出力されます。
> ls 任意のディレクトリ/ml-agents/unity-volume
3DBall.x86_64 3DBall_Data
ハイパーパラメーターファイルの用意
ハイパーパラメーターファイルをunity-volume
フォルダにコピーしておきます。
> cp ml-agents/config/trainer_config.yaml ml-agents/unity-volume/
Dockerイメージを作成する
すでにDockerfile
が用意されているので、docker build
するだけ。楽々ですね。
ml-agents-on-docker
はイメージ名となりますので、任意でOKです。
> docker build -t ml-agents-on-docker ./ml-agents
(略)
Removing intermediate container c1384ee9d5a6
---> 9ff8832e88dc
Step 19/20 : EXPOSE 5005
---> Running in 53253a272fb4
Removing intermediate container 53253a272fb4
---> f0b43146ad36
Step 20/20 : ENTRYPOINT ["mlagents-learn"]
---> Running in f80f7504b790
Removing intermediate container f80f7504b790
---> 9caddd5a62b1
Successfully built 9caddd5a62b1
Successfully tagged ml-agents-on-docker:latest
> docker images
REPOSITORY TAG IMAGE ID CREATED SIZE
ml-agents-on-docker latest 9caddd5a62b1 2 minutes ago 1.23GB
注意点
ml-agents
ディレクトリ直下でdocker build
コマンドを実行するとエラーになるのでご注意ください。
> cd ml-agents
> docker build -t ml-agents-on-docker .
error checking context: 'file ('/Users/xxx/xxxxx/ml-agents/UnitySDK/Temp') not found or excluded by .dockerignore'.
v0.10.0からPython 3.7.xにも対応しましたが、Dockerfile
を覗いてみるとPyhtonのバージョンは3.6.4
のままでした。
ENV PYTHON_VERSION 3.6.4
Dockerコンテナの実行
ビルドできたら実行してみます。
# ml-agents-3dball: コンテナ名(任意)
# ml-agents-on-docker: Dockerでビルド時に付けたイメージ名
# 3DBall: Unityでビルド時に付けたアプリの名前(拡張子なし)
> cd ml-agents
> docker run -it --rm \
--name ml-agents-3dball \
--mount type=bind,source="$(pwd)"/unity-volume,target=/unity-volume \
-p 5005:5005 \
-p 6006:6006 \
ml-agents-on-docker:latest \
--docker-target-name=unity-volume \
--env=3DBall \
--train \
trainer_config.yaml
mlagents-learn
コマンドの--docker-target-name
オプションはdocker run
コマンドの--workdir(-w)
に置き換えることもできます。
> docker run -it --rm \
--name ml-agents-3dball \
--mount type=bind,source="$(pwd)"/unity-volume,target=/unity-volume \
-w /unity-volume \
-p 5005:5005 \
-p 6006:6006 \
ml-agents-on-docker:latest \
--env=3DBall \
--train \
trainer_config.yaml
fishシェルで実行する場合は、"$(pwd)"
を"$PWD"
に置き換えます。
> cd ml-agents
> docker run -it --rm \
--name ml-agents-3dball \
--mount type=bind,source="$PWD"/unity-volume,target=/unity-volume \
-p 5005:5005 \
-p 6006:6006 \
ml-agents-on-docker:latest \
--docker-target-name=unity-volume \
--env=3DBall \
--train \
trainer_config.yaml
実行すると、学習が始まります。
trainer_config.yaml
のmax_steps
で指定されているステップ数が完了するか、ctrl
+ c
キーで学習が終了します。
> docker run (略)
▄▄▄▓▓▓▓
╓▓▓▓▓▓▓█▓▓▓▓▓
,▄▄▄m▀▀▀' ,▓▓▓▀▓▓▄ ▓▓▓ ▓▓▌
▄▓▓▓▀' ▄▓▓▀ ▓▓▓ ▄▄ ▄▄ ,▄▄ ▄▄▄▄ ,▄▄ ▄▓▓▌▄ ▄▄▄ ,▄▄
▄▓▓▓▀ ▄▓▓▀ ▐▓▓▌ ▓▓▌ ▐▓▓ ▐▓▓▓▀▀▀▓▓▌ ▓▓▓ ▀▓▓▌▀ ^▓▓▌ ╒▓▓▌
▄▓▓▓▓▓▄▄▄▄▄▄▄▄▓▓▓ ▓▀ ▓▓▌ ▐▓▓ ▐▓▓ ▓▓▓ ▓▓▓ ▓▓▌ ▐▓▓▄ ▓▓▌
▀▓▓▓▓▀▀▀▀▀▀▀▀▀▀▓▓▄ ▓▓ ▓▓▌ ▐▓▓ ▐▓▓ ▓▓▓ ▓▓▓ ▓▓▌ ▐▓▓▐▓▓
^█▓▓▓ ▀▓▓▄ ▐▓▓▌ ▓▓▓▓▄▓▓▓▓ ▐▓▓ ▓▓▓ ▓▓▓ ▓▓▓▄ ▓▓▓▓`
'▀▓▓▓▄ ^▓▓▓ ▓▓▓ └▀▀▀▀ ▀▀ ^▀▀ `▀▀ `▀▀ '▀▀ ▐▓▓▌
▀▀▀▀▓▄▄▄ ▓▓▓▓▓▓, ▓▓▓▓▀
`▀█▓▓▓▓▓▓▓▓▓▌
¬`▀▀▀█▓
INFO:mlagents.trainers:CommandLineOptions(debug=False, num_runs=1, seed=-1, env_path='3DBall', run_id='ppo', load_model=False, train_model=True, save_freq=50000, keep_checkpoints=5, base_port=5005, num_envs=1, curriculum_folder=None, lesson=0, slow=False, no_graphics=False, multi_gpu=False, trainer_config_path='trainer_config.yaml', sampler_file_path=None, docker_target_name='unity-volume', env_args=None, cpu=False)
INFO:mlagents.envs:
'Ball3DAcademy' started successfully!
Unity Academy name: Ball3DAcademy
Number of Training Brains : 0
Reset Parameters :
gravity -> 9.8100004196167
scale -> 1.0
mass -> 1.0
(略)
2019-11-07 05:31:38.593118: I tensorflow/core/platform/cpu_feature_guard.cc:142] Your CPU supports instructions that this TensorFlow binary was not compiled to use: AVX2 FMA
2019-11-07 05:31:38.604956: I tensorflow/core/platform/profile_utils/cpu_utils.cc:94] CPU Frequency: 2400000000 Hz
2019-11-07 05:31:38.607307: I tensorflow/compiler/xla/service/service.cc:168] XLA service 0x39ccf70 executing computations on platform Host. Devices:
2019-11-07 05:31:38.607465: I tensorflow/compiler/xla/service/service.cc:175] StreamExecutor device (0): <undefined>, <undefined>
(略)
2019-11-07 05:31:40.131532: W tensorflow/compiler/jit/mark_for_compilation_pass.cc:1412] (One-time warning): Not using XLA:CPU for cluster because envvar TF_XLA_FLAGS=--tf_xla_cpu_global_jit was not set. If you want XLA:CPU, either set that envvar, or use experimental_jit_scope to enable XLA:CPU. To confirm that XLA is active, pass --vmodule=xla_compilation_cache=1 (as a proper command-line flag, not via TF_XLA_FLAGS) or set the envvar XLA_FLAGS=--xla_hlo_profile.
INFO:mlagents.envs:Hyperparameters for the PPOTrainer of brain 3DBall:
trainer: ppo
batch_size: 64
beta: 0.001
buffer_size: 12000
epsilon: 0.2
hidden_units: 128
lambd: 0.99
learning_rate: 0.0003
learning_rate_schedule: linear
max_steps: 5.0e4
memory_size: 256
normalize: True
num_epoch: 3
num_layers: 2
time_horizon: 1000
sequence_length: 64
summary_freq: 1000
use_recurrent: False
vis_encode_type: simple
reward_signals:
extrinsic:
strength: 1.0
gamma: 0.99
summary_path: /unity-volume/summaries/ppo_3DBall
model_path: /unity-volume/models/ppo-0/3DBall
keep_checkpoints: 5
WARNING:tensorflow:From /ml-agents/mlagents/trainers/trainer.py:223: The name tf.summary.text is deprecated. Please use tf.compat.v1.summary.text instead.
WARNING:tensorflow:From /ml-agents/mlagents/trainers/trainer.py:223: The name tf.summary.text is deprecated. Please use tf.compat.v1.summary.text instead.
INFO:mlagents.trainers: ppo: 3DBall: Step: 1000. Time Elapsed: 10.062 s Mean Reward: 1.167. Std of Reward: 0.724. Training.
(略)
INFO:mlagents.trainers: ppo: 3DBall: Step: 10000. Time Elapsed: 109.367 s Mean Reward: 36.292. Std of Reward: 28.127. Training.
(略)
INFO:mlagents.trainers: ppo: 3DBall: Step: 49000. Time Elapsed: 520.514 s Mean Reward: 100.000. Std of Reward: 0.000. Training.
(略)
Converting /unity-volume/models/ppo-0/3DBall/frozen_graph_def.pb to /unity-volume/models/ppo-0/3DBall.nn
IGNORED: Cast unknown layer
IGNORED: StopGradient unknown layer
GLOBALS: 'is_continuous_control', 'version_number', 'memory_size', 'action_output_shape'
IN: 'vector_observation': [-1, 1, 1, 8] => 'sub_3'
IN: 'epsilon': [-1, 1, 1, 2] => 'mul_1'
OUT: 'action', 'action_probs'
DONE: wrote /unity-volume/models/ppo-0/3DBall.nn file.
INFO:mlagents.trainers:Exported /unity-volume/models/ppo-0/3DBall.nn file
INFO:mlagents.envs:Environment shut down with return code 0.
WARNING
がかなり出力されますが、学習できました。
TensorBoard
を利用して学習の進捗を視覚的に確認することもできます。
> docker exec -it \
ml-agents-3dball \
tensorboard \
--logdir=/unity-volume/summaries \
--host=0.0.0.0
(略)
_np_qint32 = np.dtype([("qint32", np.int32, 1)])
/usr/local/lib/python3.6/site-packages/tensorflow/python/framework/dtypes.py:525: FutureWarning: Passing (type, 1) or '1type' as a synonym of type is deprecated; in a future version of numpy, it will be understood as (type, (1,)) / '(1,)type'.
np_resource = np.dtype([("resource", np.ubyte, 1)])
TensorBoard 1.14.0 at http://0.0.0.0:6006/ (Press CTRL+C to quit)

学習結果をアプリに組み込む
学習結果は、ml-agents/unity-volume
フォルダ内に保存されます。
それをUnityアプリに組み込むことで学習結果をUnityアプリに反映できます。
> ls unity-volume/
3DBall.x86_64 3DBall_Data csharp_timers.json models summaries trainer_config.yaml
> tree unity-volume/models/
unity-volume/models/
└── ppo-0
├── 3DBall
│ ├── checkpoint
│ ├── frozen_graph_def.pb
│ ├── model-50000.cptk.data-00000-of-00001
│ ├── model-50000.cptk.index
│ ├── model-50000.cptk.meta
│ ├── model-50001.cptk.data-00000-of-00001
│ ├── model-50001.cptk.index
│ ├── model-50001.cptk.meta
│ └── raw_graph_def.pb
└── 3DBall.nn
2 directories, 10 files
Unityアプリの設定
Playerの設定を行います。
- Unityアプリの[Edit]メニューから[Project Settings]を選択する
- [Inspector]ビューの[Other Settings]欄で以下を確認・設定する
- Scripting Backendが
Mono
になっている - Api Conpatibility Levelが
.NET 4.x
になっている
- Scripting Backendが
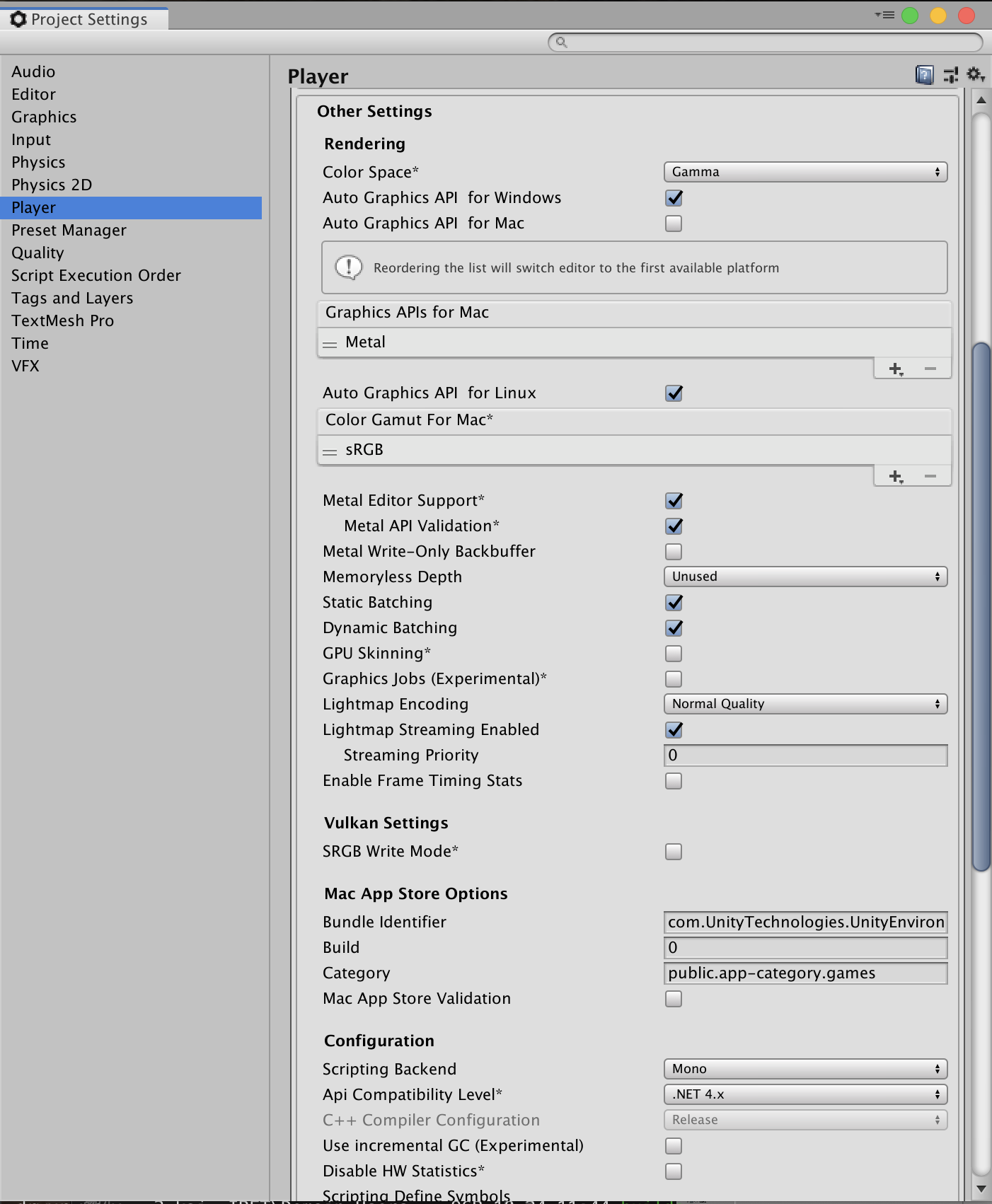
学習結果ファイルの取り込み
ターミナルかFinderで学習結果を以下フォルダにコピーします。
- 学習結果ファイル:
models/ppo-0/3DBall.nn
- 保存先:
UnitySDK/Assets/ML-Agents/Examples/3DBall/TFModels/
※すでに保存先に3DBall.nn
ファイルが存在していますので、リネームします。
> cp models/ppo-0/3DBall.nn ml-agents/UnitySDK/Assets/ML-Agents/Examples/3DBall/TFModels/3DBall_new.nn
- Unityアプリの下パネルにある[Project]タブから以下のフォルダまで開く
- [Assets] > [ML-Agents] > [Examples] > [3DBall] > [Scenes]
- 開いたら、[3DBall]ファイルがあるので、ダブルクリックして開く
- [Hierarchy]パネルから[Agent]を選択する
- Unityアプリの[Project]パネルで以下フォルダを選択する
- [Assets] > [ML-Agents] > [Examples] > [3DBall] > [TFModels]
- Unityアプリの[Inspector]パネルにある[Model]という項目に[TFModels]フォルダ内の
3DBall_new.nn
ファイルをドラッグ&ドロップする
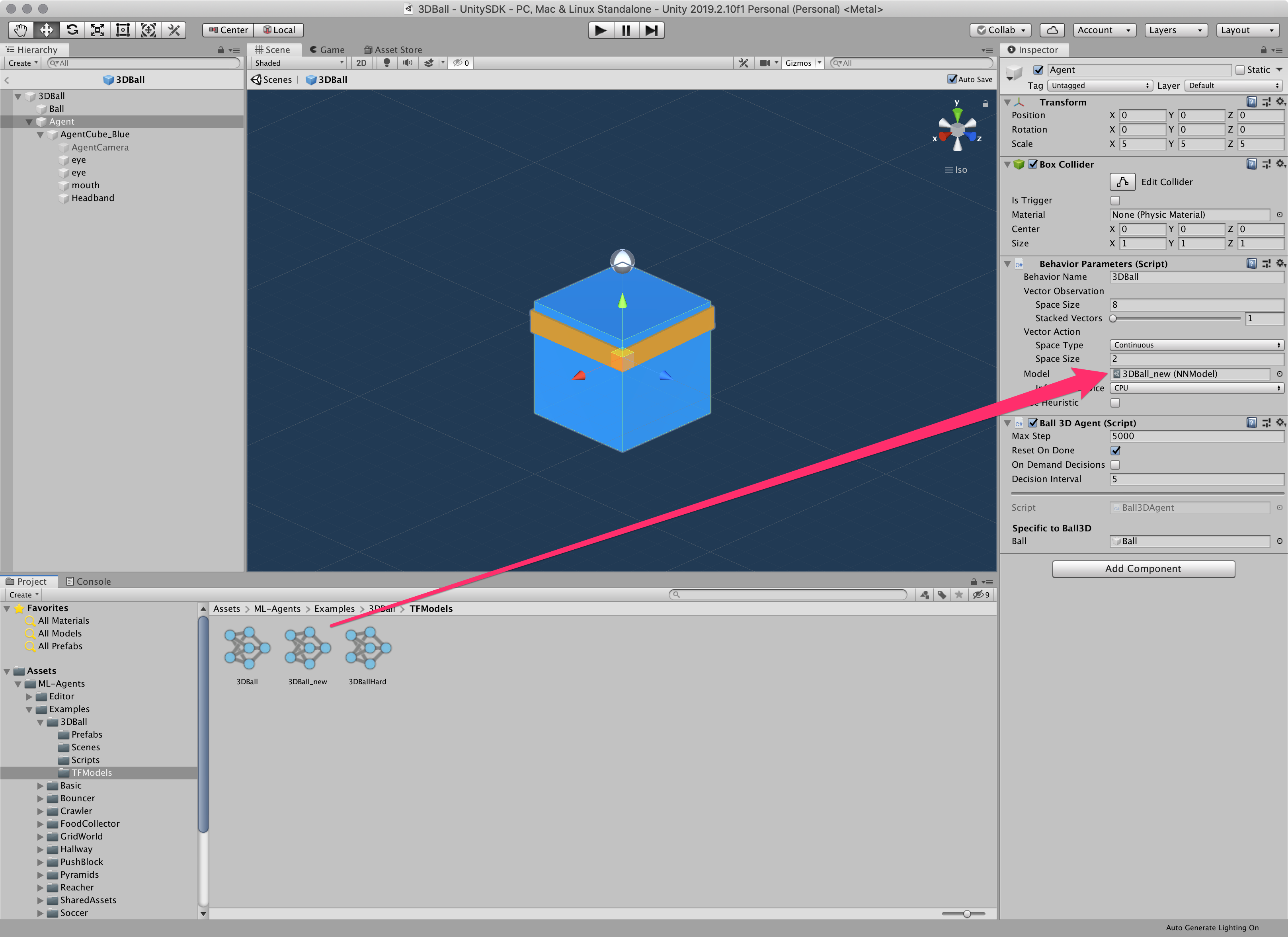
- Unity上部にある[▶]ボタンをクリックする
これで、学習結果が組み込まれた状態でアプリが起動します。
参考
Macでhomebrewを使ってUnityをインストールする(Unity Hub、日本語化対応)
https://qiita.com/kai_kou/items/445e614fb71f2204e033
MacでUnity ML-Agentsの環境を構築する(v0.11.0対応) - Qiita
https://qiita.com/kai_kou/items/0d40157cbc303fb10c22
ml-agents/Using-Docker.md at 0.10.1 · Unity-Technologies/ml-agents
https://github.com/Unity-Technologies/ml-agents/blob/0.10.1/docs/Using-Docker.md