これは深層学習以外の機械学習と応用技術 by QuantumCore Advent Calendar 2019の15日目の記事です。
やりたいこと
時系列情報を用いてNBA選手の活躍予測をする。
今回はユタ・ジャズ所属のDonovan Mitchell選手の「出場分あたり得点数」を予測します。
Donovan Mitchell (Basketball Reference)
ちなみに僕はバスケットボール初心者です。
仮定
「シーズンでの活躍の浮き沈みって毎シーズン同じになるんじゃない?」
例えば、「シーズンはじめは不調だけど途中で調子を取り戻し、シーズン終盤で活躍のピークを迎える」
かなり粗い仮定ですが、これとQoreSDKでどこまでできるか試してみました。
データと予測内容
データ
Basketball Referenceをスクレイピングして、各試合における選手のBox Score(出場記録)を取得。
なおBaketball Referenceには1954年頃からの試合データがあり、一応全データをスクレイピングしました。もし内容が気になる方はご連絡ください。
予測するもの
- 説明変数: 開幕からの日数
- 目的変数: 出場分あたり得点数 (
PTS/MP
)
11月中旬までのDonovan Mitchellの出場分あたり得点数は以下のようになります。
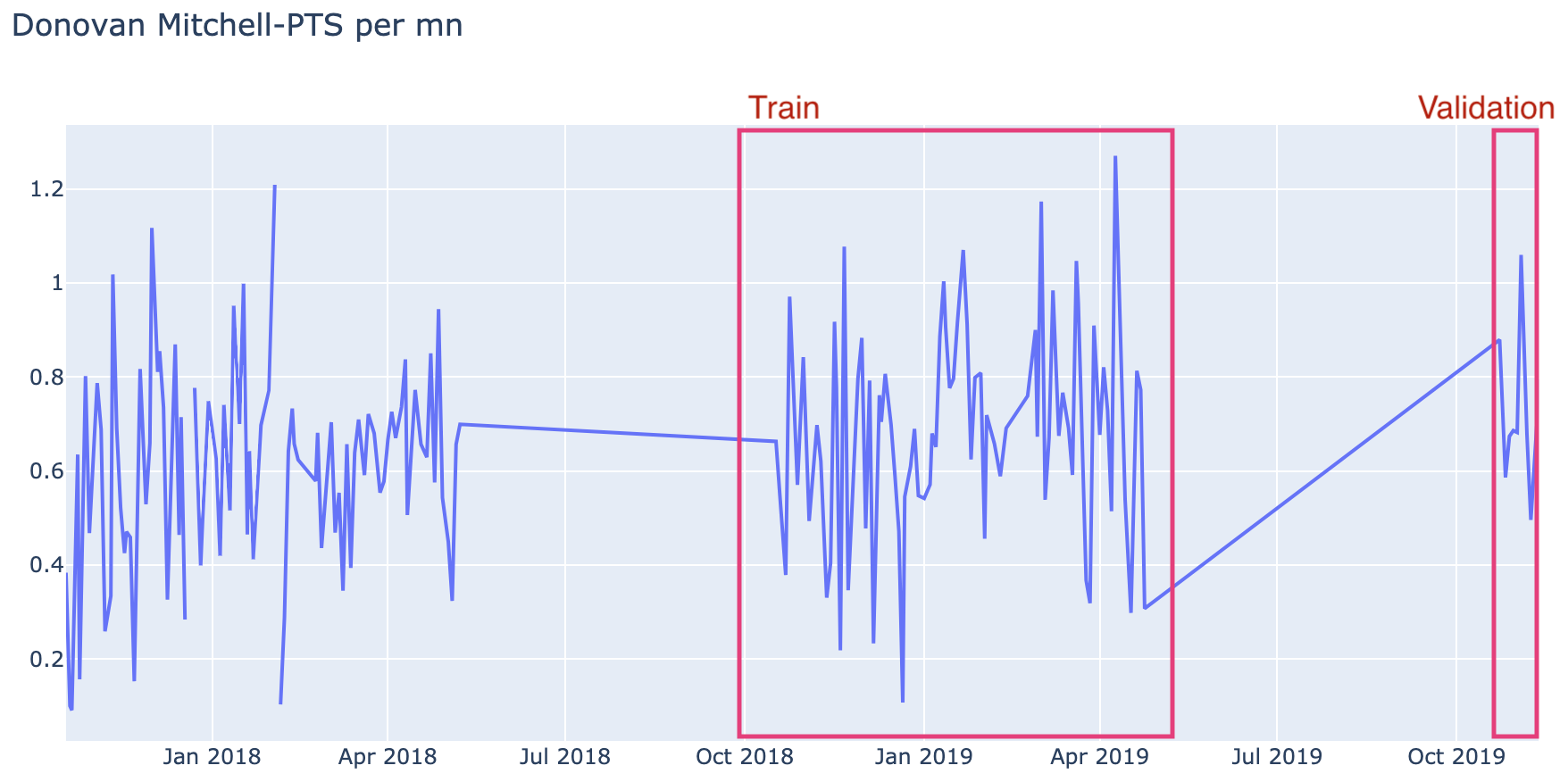
今回は18/19シーズンのデータを使ってTrainを、19/20シーズンの途中まででValidationをします(モデルの変更は行いませんが)。その後、実際の試合に対してDonovanの活躍予測をします。
コード
接続
from qore_sdk.client import WebQoreClient
client = WebQoreClient(username="", password="p@$$w0rd", endpoint="")
データ準備
Donovan.csv
にはスクレイピングした選手の成績があるとします。
import pandas as pd
donovan = pd.read_csv('Donovan.csv', parse_dates=['date'], index_col='date')
(再出)
- 説明変数: 開幕からの日数
- 目的変数: 出場分あたり得点数 (
PTS/MP
)
# シーズン開始前の10月1日を規準とする。
# 開幕の日のほうが良いかもしれない
X = donovan[(datetime.datetime(year=2019, month=10, day=1)>donovan.index) & (donovan.index>datetime.datetime(year=2018, month=10, day=1))].index
X = X - datetime.datetime(year=2018, month=10, day=1)
y = donovan[donovan.index.year==2018]['PTS per mn']
データ分割
import sklearn.model_selection as model_selection
X_train, X_valid, y_train, y_valid = model_selection.train_test_split(
X, y, shuffle=False, random_state=44
)
学習と予測
# Train
res = client.regression_train(X=X_train.values, Y=y_train.values)
# 予測
pred = client.regression_predict(X=X_valid.values)
バリデーション(という名の確認)
import sklearn.metrics as metrics
rmse = metrics.mean_squared_error(y_valid, pred["Y"])**.5
mae = metrics.mean_absolute_error(y_valid, pred["Y"])
print("RMSE=", rmse)
print("MAE=", mae)
print("RMSE/MAE=", rmse/mae)
結果
学習の時点でHTTPError: HTTP Error 500: INTERNAL SERVER ERROR
が発生。解決次第、記事をアップデートします。
予想される課題
- 仮定が単純すぎる
- 時間以外の要素を考慮するべきかもしれない
- そもそもパフォーマンス評価に軸が「出場分あたりの得点数」だけはおかしい
- まずは、結果を出そう。
QoreSDKの使い心地
別データセットで学習・予測をすると、確かにお手軽さ・楽チンさがあります。Reservoir Computingのような、利点をもつが複雑な計算を簡単に再現できるツールというのはもっと知られるべきだと思いました。
一方、その分ブラックボックス的印象を受けることがあり、運営の初日記事やドキュメンテーションを始めとした説明をしっかりと理解する必要があります。特に、ドキュメンテーションには初日記事にあるような、背景・理論の説明ページがあってもいいのかなと感じました。
いずれにしても、このようなツール開発に時間を注くQuantumCoreの皆様に敬意を示します。