この記事は BrainPad Advent Calendar 2021 2日目の記事です。
はじめに
データサイエンティストです。普段は記事を書かないROM専ですが、たまにはアドベントカレンダーなるお祭り騒ぎに参加してみようと思います。
この記事では、データサイエンティストになじみの深い Matplotlib を用いて、ゲームを作ります。
データサイエンティストは、Jupyter Notebook や Matplotlib が大好きです。
また、データサイエンティストは、白黒はっきりさせることが大好きです。
つまり、データサイエンティストであれば、Jupyter Notebook 上で Matplotlib を用いてオセロができると聞いたら、胸のドキドキが止まらないことでしょう!
※ 個人の感想です。好みには個人差があります。
※ 発言は個人の偏見であって、所属組織を代表するものではありません。
完成系
最初に完成系を見せておきましょう。
石を置くために、マスをクリックしています。
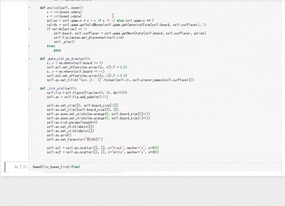
では、作っていきます。
Matplotlib によるインタラクティブな可視化
Jupyter Notebook 上で Matplotlib を使う場合には、ほとんどの人は以下を実行すると思います。
%matplotlib inline
Jupyter Notebook 上で Matplotlib によるインタラクティブな可視化を行うには、以下を実行しましょう。
%matplotlib notebook
さて、Matplotlib でクリックやキーボードを用いたインタラクティブな操作を受け付けるには、connectメソッドを用いて、グラフにイベント発生時の処理を結びつけます。公式ドキュメントを見て頂くのが一番だとは思いますが、下記に簡単な例を載せておきます。
import matplotlib.pyplot as plt
import numpy as np
xy1 = []
fig = plt.figure()
ax1 = fig.add_subplot(111)
sc1 = ax1.scatter([], [], color='blue')
def on_click(event):
xy1.append((event.xdata, event.ydata)) # クリック位置の座標(グラフのx軸とy軸のこと)をxy1に追加
sc1.set_offsets(xy1) # 散布図にxy1を設定
plt.draw() # グラフの再描画
plt.connect('button_press_event', on_click) # eventを引数に取る関数on_clickをbutton_press_eventと紐づける
plt.show()
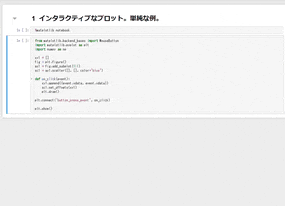
button_press_eventはマウスのクリックに対応するイベントの指定で、どのボタン(LEFT, MIDDLE, RIGHT)が押されたかや、座標の情報などの情報を得ることができます。
インタラクティブモードでは、plt.drawでグラフを再描画することが可能です。
import matplotlib.pyplot as plt
import numpy as np
fig = plt.figure()
ax1 = fig.add_subplot(111)
ax1.set_xlim([-1, 1])
ax1.set_ylim([-1, 1])
sc1 = ax1.scatter([], [], color='blue')
x = np.linspace(start=-1, stop=1, num=100)
def on_key(event):
ax1.set_title('"{}" was pressed'.format(event.key)) # 押されたキーを表示
try:
k = int(event.key)
y = np.sin(k * x)
sc1.set_offsets(np.array([x, y]).T)
plt.draw()
except ValueError:
pass
plt.connect('key_press_event', on_key) # eventを引数に取る関数on_keyをkey_press_eventと紐づける
plt.show()
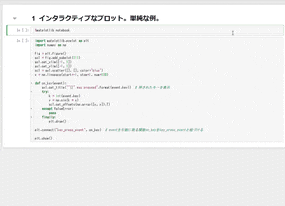
このように、キー入力に対応して処理を行うことも可能です。
インタラクティブなプロットが作れることを知っておくと、簡易的なアノテーションツールの作成やシミュレーションの可視化など、データサイエンス業務やAI開発業務でも役立つシチュエーションがありますので、できるということだけでも知っておくと良いでしょう。
ちなみに、%matplotlib notebook として普通にプロットを作成するだけでも、ズームインができるなど多少インタラクティブな要素が得られますが、単純なインタラクティブなデータ可視化であれば、bokehなどの別ライブラリを使った方が良いでしょう。
また、ゲームとして第三者に提供するのであれば Dash と Plotly を使った方が良いのではないかと言う意見はごもっともですが、今回は「Jupyter Notebook 上で Matplotlib を用いてオセロができる」ということ自体がデータサイエンティストのハートをがっちり掴んで離さないと考え、今回はmatplotlibを、オセロをプレイするためのUIとして利用します。
AlphaZeroオセロモデルの準備
オセロをプレイできるようにするために、オセロAIを用意しましょう。
ルールベースで自作しても良いですし、機械学習で自作しても良いです。
今回は手っ取り早く、MIT-Licenceで公開されているAlpha Zero Generalから、学習済みモデルとコードを使わせて頂きます。
!git clone https://github.com/suragnair/alpha-zero-general
%cd alpha-zero-general
AlphaZero についてここでは説明しませんが、概要に興味がある方は、BrainPad の Platinum Data Blog の下記記事をお読みいただければ、雰囲気は掴めるのではないかと思います。
強化学習入門 Part3 - AlphaGoZeroでも重要な技術要素! モンテカルロ木探索の入門 -
オセロをプレイできるようにする
先ほど、matplotlibで人がクリックした座標を取得する方法や、データを更新する方法を説明しました。
あとは、alpha-zero-general のコードと組み合わせれば完成です。
組み合わせる際に、ちょっと変な書き方してしまっている部分がありますが、愛嬌ということで。
# 元コード(https://github.com/suragnair/alpha-zero-general)のpit.py, Arena.py, OthelloPlayers.pyを参考に作成
from matplotlib import pylab as plt
from MCTS import MCTS
from othello.OthelloGame import OthelloGame
from othello.pytorch.NNet import NNetWrapper as NNet
from utils import dotdict
import numpy as np
class GameUI(object):
def __init__(self, is_human_first=True):
"""
Args:
is_human_first (bool) Trueなら人間が先手、Falseなら人間が後手
"""
self.game = OthelloGame(8)
self.curPlayer = 1
# human
self.player1 = lambda x: 'Human'
# AlphaZero
nnet = NNet(self.game)
nnet.load_checkpoint('./pretrained_models/othello/pytorch/', '8x8_100checkpoints_best.pth.tar')
args = dotdict({'numMCTSSims': 50, 'cpuct':1.0})
mcts = MCTS(self.game, nnet, args)
self.player2 = lambda x: np.argmax(mcts.getActionProb(x, temp=1.0))
# game情報の初期化
if is_human_first:
self.players = {-1: self.player2, 1:self.player1}
self.player_names = {-1:'AlphaZero', 1:'You'}
else:
self.players = {-1: self.player1, 1:self.player2}
self.player_names = {-1:'You', 1:'AlphaZero'}
self.board = self.game.getInitBoard()
self.board_size = self.board.shape
self.it = 0
self._init_plot()
self.fig.show()
self._play()
def _play(self):
while self.game.getGameEnded(self.board, self.curPlayer) == 0:
self.it += 1
self._make_plot_wo_draw()
self.fig.canvas.draw()
action = self.players[self.curPlayer](self.game.getCanonicalForm(self.board, self.curPlayer))
if action == 'Human': # human
valids = self.game.getValidMoves(self.game.getCanonicalForm(self.board, self.curPlayer), 1)
if valids[self.game.n ** 2] == 1: # パスしかできないとき
action = self.game.n ** 2
self.board, self.curPlayer = self.game.getNextState(self.board, self.curPlayer, action)
else:
self.cid = self.fig.canvas.mpl_connect('button_press_event', self.onclick) # クリック待ち
break
else: # AlphaZero
self.board, self.curPlayer = self.game.getNextState(self.board, self.curPlayer, action)
if self.game.getGameEnded(self.board, self.curPlayer):
win_player = self.game.getGameEnded(self.board, 1)
self._make_plot_wo_draw()
self.ax.set_title('Game over: Turn {} {} Win!'.format(self.it, self.player_names[win_player]))
self.fig.canvas.draw()
def onclick(self, event):
x = int(event.xdata)
y = int(event.ydata)
action = self.game.n * x + y if x != -1 else self.game.n ** 2
valids = self.game.getValidMoves(self.game.getCanonicalForm(self.board, self.curPlayer), 1)
if valids[action] == 1:
self.board, self.curPlayer = self.game.getNextState(self.board, self.curPlayer, action)
self.fig.canvas.mpl_disconnect(self.cid)
self._play()
else:
pass
def _make_plot_wo_draw(self):
x, y = np.where(self.board == 1)
self.sc1.set_offsets(np.array([x, y]).T + 0.5)
x, y = np.where(self.board == -1)
self.sc2.set_offsets(np.array([x, y]).T + 0.5)
self.ax.set_title('Turn {}: {}'.format(self.it, self.player_names[self.curPlayer]))
def _init_plot(self):
self.fig = plt.figure(figsize=(4, 4), dpi=100)
self.ax = self.fig.add_subplot(111)
self.ax.set_xlim([0, self.board_size[1]])
self.ax.set_ylim([self.board_size[0], 0])
self.ax.axes.set_xticks(np.arange(0, self.board_size[0]+1))
self.ax.axes.set_yticks(np.arange(0, self.board_size[1]+1))
self.ax.tick_params(length=0)
self.ax.set_xticklabels([])
self.ax.set_yticklabels([])
self.ax.grid()
self.ax.set_facecolor('#2e8b57')
self.sc1 = self.ax.scatter([], [], c='black', marker='o', s=400)
self.sc2 = self.ax.scatter([], [], c='white', marker='o', s=400)
簡単にコードの補足をします。
_init_plot でグラフの書式を初期化しています。
背景色を緑色にし、grid を用いて8×8のオセロ盤面を表現しています。
軸の線やラベルを残しておくと Matplotlib らしさが出て、Matplotlib 愛好者はむしろその方が好みかもしれませんが、今回はそれらを消し、見た目をすっきりさせておきました。
player1 は黒色の丸型マーカー、player2 は白色の丸型マーカーの散布図によって表現しています。
_play では、人間の手番かゲーム終了になるまで、ゲームを進めています。
人間の手番になったら、クリックイベントの発生を待ちます。
onclick では、クリックされた位置を取得し、それが着手可能なマスであればゲームを進めます。
以上、オセロゲームの中身は Alpha Zero General のコードを活用させて頂き、可視化と入力は Matplotlib を利用することで、
とっても簡単にオセロゲームが遊べるUIを作ることができました。
GameUI(is_human_first=True)
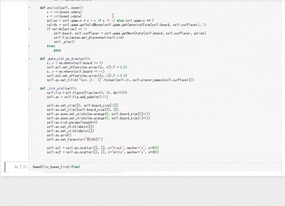
対局の振り返り
さて、オセロが遊べるようになったのは良いのですが、
困ったことに、全然楽しくありません。
Matplotlib で作ったゲーム画面を派手にするとか、
効果音を再生するとか、
キャラクターの顔画像やセリフを表示するとか。
どうすれば楽しくなるのか考えましたが、結局のところ、全然勝てないから楽しくないのだと気づきました。
オセロで勝てるようになるにはどうすれば良いか。元将棋部の私なりに出した結論は、定石や基本の手筋を学ぶことと、対局の振り返りをすることです。
定石や基本の手筋はググることにして、ここでは、対局の振り返り機能を追加してみましょう。
まずは先ほどの GameUI クラスに history というメンバ変数を追加し、各手番で選択された action を保存するように修正します。
# 元コード(https://github.com/suragnair/alpha-zero-general)のpit.py, Arena.py, OthelloPlayers.pyを参考に作成
from matplotlib import pylab as plt
from MCTS import MCTS
from othello.OthelloGame import OthelloGame
from othello.pytorch.NNet import NNetWrapper as NNet
from utils import dotdict
import numpy as np
class GameUI(object):
def __init__(self, is_human_first=True):
"""
Args:
is_human_first (bool) Trueなら人間が先手、Falseなら人間が後手
"""
self.game = OthelloGame(8)
self.curPlayer = 1
# human
self.player1 = lambda x: 'Human'
# AlphaZero
nnet = NNet(self.game)
nnet.load_checkpoint('./pretrained_models/othello/pytorch/', '8x8_100checkpoints_best.pth.tar')
args = dotdict({'numMCTSSims': 50, 'cpuct':1.0})
mcts = MCTS(self.game, nnet, args)
self.player2 = lambda x: np.argmax(mcts.getActionProb(x, temp=1.0))
# game情報の初期化
if is_human_first:
self.players = {-1: self.player2, 1:self.player1}
self.player_names = {-1:'AlphaZero', 1:'You'}
else:
self.players = {-1: self.player1, 1:self.player2}
self.player_names = {-1:'You', 1:'AlphaZero'}
self.board = self.game.getInitBoard()
self.board_size = self.board.shape
self.it = 0
self.history = []
self._init_plot()
self.fig.show()
self._play()
def _play(self):
while self.game.getGameEnded(self.board, self.curPlayer) == 0:
self.it += 1
self._make_plot_wo_draw()
self.fig.canvas.draw()
action = self.players[self.curPlayer](self.game.getCanonicalForm(self.board, self.curPlayer))
if action == 'Human': # human
valids = self.game.getValidMoves(self.game.getCanonicalForm(self.board, self.curPlayer), 1)
if valids[self.game.n ** 2] == 1: # パスしかできないとき
action = self.game.n ** 2
self.history.append(action)
self.board, self.curPlayer = self.game.getNextState(self.board, self.curPlayer, action)
else:
self.cid = self.fig.canvas.mpl_connect('button_press_event', self.onclick) # クリック待ち
break
else: # AlphaZero
self.history.append(action)
self.board, self.curPlayer = self.game.getNextState(self.board, self.curPlayer, action)
if self.game.getGameEnded(self.board, self.curPlayer):
win_player = self.game.getGameEnded(self.board, 1)
self._make_plot_wo_draw()
self.ax.set_title('Game over: Turn {} {} Win!'.format(self.it, self.player_names[win_player]))
self.fig.canvas.draw()
def onclick(self, event):
x = int(event.xdata)
y = int(event.ydata)
action = self.game.n * x + y if x != -1 else self.game.n ** 2
valids = self.game.getValidMoves(self.game.getCanonicalForm(self.board, self.curPlayer), 1)
if valids[action] == 1:
self.history.append(action)
self.board, self.curPlayer = self.game.getNextState(self.board, self.curPlayer, action)
self.fig.canvas.mpl_disconnect(self.cid)
self._play()
else:
pass
def _make_plot_wo_draw(self):
x, y = np.where(self.board == 1)
self.sc1.set_offsets(np.array([x, y]).T + 0.5)
x, y = np.where(self.board == -1)
self.sc2.set_offsets(np.array([x, y]).T + 0.5)
self.ax.set_title('Turn {}: {}'.format(self.it, self.player_names[self.curPlayer]))
def _init_plot(self):
self.fig = plt.figure(figsize=(4, 4), dpi=100)
self.ax = self.fig.add_subplot(111)
self.ax.set_xlim([0, self.board_size[1]])
self.ax.set_ylim([self.board_size[0], 0])
self.ax.axes.set_xticks(np.arange(0, self.board_size[0]+1))
self.ax.axes.set_yticks(np.arange(0, self.board_size[1]+1))
self.ax.tick_params(length=0)
self.ax.set_xticklabels([])
self.ax.set_yticklabels([])
self.ax.grid()
self.ax.set_facecolor('#2e8b57')
self.sc1 = self.ax.scatter([], [], c='black', edgecolors='gray', marker='o', s=400)
self.sc2 = self.ax.scatter([], [], c='white', edgecolors='gray', marker='o', s=400)
gameui = GameUI(is_human_first=True)
では、対局の振り返りができるようにします。
同じクラスに機能を追加しても良いのですが、今回は別クラスとして作成しました。
# 元コード(https://github.com/suragnair/alpha-zero-general)のpit.py, Arena.py, OthelloPlayers.pyを参考に作成
from matplotlib import pylab as plt
from MCTS import MCTS
from othello.OthelloGame import OthelloGame
from othello.pytorch.NNet import NNetWrapper as NNet
from utils import dotdict
import numpy as np
class GameViewer(object):
def __init__(self, history, numMCTSSims=50):
self.game = OthelloGame(8)
self.curPlayer = 1
self.history = history
# AlphaZero
nnet = NNet(self.game)
nnet.load_checkpoint('./pretrained_models/othello/pytorch/', '8x8_100checkpoints_best.pth.tar')
args = dotdict({'numMCTSSims': numMCTSSims, 'cpuct':1.0})
self.mcts = MCTS(self.game, nnet, args)
# game情報の初期化
self.player_names = {-1:'White', 1:'Black'}
self.board = self.game.getInitBoard()
self.board_size = self.board.shape
self.it = 0
self.fig = plt.figure(figsize=(4, 4), dpi=100)
self.ax = self.fig.add_subplot(111)
self.it += 1
self._init_plot()
self.fig.show()
self._make_plot_wo_draw()
self.fig.canvas.draw()
self.cid = self.fig.canvas.mpl_connect('button_press_event', self.onclick) # クリック待ち
def _play(self):
action = self.history[self.it - 1]
self.board, self.curPlayer = self.game.getNextState(self.board, self.curPlayer, action)
self._make_plot_wo_draw()
self.fig.canvas.draw()
if self.game.getGameEnded(self.board, self.curPlayer):
win_player = self.game.getGameEnded(self.board, 1)
self.ax.set_title('Game over: Turn {} {} Win!'.format(self.it, self.player_names[win_player]))
self.fig.canvas.draw()
self.fig.canvas.mpl_disconnect(self.cid)
else:
self.it+=1
def onclick(self, event):
if event.button == 1:
self._play()
def _make_plot_wo_draw(self):
x, y = np.where(self.board == 1)
self.sc1.set_offsets(np.array([x, y]).T + 0.5)
x, y = np.where(self.board == -1)
self.sc2.set_offsets(np.array([x, y]).T + 0.5)
obs = self.game.getCanonicalForm(self.board, self.curPlayer)
if self.game.getGameEnded(self.board, self.curPlayer) == 0:
policy = self.mcts.getActionProb(obs, temp=1.0)
self.pcf.set_data(np.array(policy[:-1]).reshape(self.board_size).T)
else:
self.pcf.set_data(np.zeros(self.board_size))
self.ax.set_title('Turn {}: {}'.format(self.it, self.player_names[self.curPlayer]))
def _init_plot(self):
self.ax.set_xlim([0, self.board_size[1]])
self.ax.set_ylim([self.board_size[0], 0])
self.ax.axes.set_xticks(np.arange(0, self.board_size[0]+1))
self.ax.axes.set_yticks(np.arange(0, self.board_size[1]+1))
self.ax.tick_params(length=0)
self.ax.set_xticklabels([])
self.ax.set_yticklabels([])
self.pcf = self.ax.pcolorfast(np.zeros(self.board_size), cmap=plt.cm.Greens, vmin=0, vmax=0.5)
self.ax.grid()
self.sc1 = self.ax.scatter([], [], c='black', edgecolors='gray', marker='o', s=400)
self.sc2 = self.ax.scatter([], [], c='white', edgecolors='gray', marker='o', s=400)
簡単にコードの補足をします。
基本的には、左クリックで着手を再現して振り返るだけの機能です。
__init__ の中で今回も MCTS のインスタンスを用意しています。これは、各局面でどの手が良かったかを教えてくれる AI として使います。
_init_plot で AI が各局面でどの手が良いと判断しているかを表示する機能を追加しています。具体的には pcolorfast により、マスの色の濃淡で表現しています。そうすると player2 が白色の丸型マーカーだと背景色に埋もれてしまうため、灰色の線で囲むようにしました。
これで、対局を振り返りながら、どうすれば良かったのかを教えてもらえるようになりました。
tmp = GameViewer(gameui.history)

ヒントモード
これで対局の振り返りができるようになり、どうすれば良かったのかをAlphaZeroに教えてもらえるようになりました!
しかし、私が強くなるまでには時間がかかります。アドベントカレンダーの記事の公開日も迫っています。
こうなったら!
どうすれば良かったのかをAlphaZeroに対局の振り返りで教えてもらうのではなく、対局中に教えてもらいましょう!
常にAlphaZeroが教えてくれている状態だとゲームの体を成さないので、裏ワザ的に、hキーを押すとAlphaZeroが良い手を教えてくれるようにします。ヒントモードの搭載です!
# 元コード(https://github.com/suragnair/alpha-zero-general)のpit.py, Arena.py, OthelloPlayers.pyを参考に作成
from matplotlib import pylab as plt
from MCTS import MCTS
from othello.OthelloGame import OthelloGame
from othello.pytorch.NNet import NNetWrapper as NNet
from utils import dotdict
import numpy as np
class GameUI(object):
def __init__(self, is_human_first=True):
"""
Args:
is_human_first (bool) Trueなら人間が先手、Falseなら人間が後手
"""
self.game = OthelloGame(8)
self.curPlayer = 1
# human
self.player1 = lambda x: 'Human'
# AlphaZero
nnet = NNet(self.game)
nnet.load_checkpoint('./pretrained_models/othello/pytorch/', '8x8_100checkpoints_best.pth.tar')
args = dotdict({'numMCTSSims': 50, 'cpuct':1.0})
mcts = MCTS(self.game, nnet, args)
self.player2 = lambda x: np.argmax(mcts.getActionProb(x, temp=1.0))
# ForHintMode
args = dotdict({'numMCTSSims': 100, 'cpuct':1.0})
self.mcts0 = MCTS(self.game, nnet, args)
# game情報の初期化
if is_human_first:
self.players = {-1: self.player2, 1:self.player1}
self.player_names = {-1:'AlphaZero', 1:'You'}
else:
self.players = {-1: self.player1, 1:self.player2}
self.player_names = {-1:'You', 1:'AlphaZero'}
self.board = self.game.getInitBoard()
self.board_size = self.board.shape
self.it = 0
self.history = []
self._init_plot()
self.fig.show()
self._play()
def _play(self):
while self.game.getGameEnded(self.board, self.curPlayer) == 0:
self.it += 1
self._make_plot_wo_draw()
self.fig.canvas.draw()
action = self.players[self.curPlayer](self.game.getCanonicalForm(self.board, self.curPlayer))
if action == 'Human': # human
valids = self.game.getValidMoves(self.game.getCanonicalForm(self.board, self.curPlayer), 1)
if valids[self.game.n ** 2] == 1: # パスしかできないとき
action = self.game.n ** 2
self.history.append(action)
self.board, self.curPlayer = self.game.getNextState(self.board, self.curPlayer, action)
else:
self.cid = self.fig.canvas.mpl_connect('button_press_event', self.onclick) # クリック待ち
self.cid_k = self.fig.canvas.mpl_connect('key_press_event', self.onkey)
break
else: # AlphaZero
self.history.append(action)
self.board, self.curPlayer = self.game.getNextState(self.board, self.curPlayer, action)
if self.game.getGameEnded(self.board, self.curPlayer):
win_player = self.game.getGameEnded(self.board, 1)
self._make_plot_wo_draw()
self.ax.set_title('Game over: Turn {} {} Win!'.format(self.it, self.player_names[win_player]))
self.fig.canvas.draw()
def onclick(self, event):
x = int(event.xdata)
y = int(event.ydata)
action = self.game.n * x + y if x != -1 else self.game.n ** 2
valids = self.game.getValidMoves(self.game.getCanonicalForm(self.board, self.curPlayer), 1)
if valids[action] == 1:
self.history.append(action)
self.board, self.curPlayer = self.game.getNextState(self.board, self.curPlayer, action)
self.pcf.set_data(np.ones(self.board_size)*0.34)
self.fig.canvas.mpl_disconnect(self.cid)
self.fig.canvas.mpl_disconnect(self.cid_k)
self._play()
else:
pass
def onkey(self, event):
if event.key == 'h':
obs = self.game.getCanonicalForm(self.board, self.curPlayer)
policy = self.mcts0.getActionProb(obs, temp=1.0)
self.pcf.set_data(np.array(policy[:-1]).reshape(self.board_size).T)
def _make_plot_wo_draw(self):
x, y = np.where(self.board == 1)
self.sc1.set_offsets(np.array([x, y]).T + 0.5)
x, y = np.where(self.board == -1)
self.sc2.set_offsets(np.array([x, y]).T + 0.5)
self.ax.set_title('Turn {}: {}'.format(self.it, self.player_names[self.curPlayer]))
def _init_plot(self):
self.fig = plt.figure(figsize=(4, 4), dpi=100)
self.ax = self.fig.add_subplot(111)
self.ax.set_xlim([0, self.board_size[1]])
self.ax.set_ylim([self.board_size[0], 0])
self.ax.axes.set_xticks(np.arange(0, self.board_size[0]+1))
self.ax.axes.set_yticks(np.arange(0, self.board_size[1]+1))
self.ax.tick_params(length=0)
self.ax.set_xticklabels([])
self.ax.set_yticklabels([])
self.pcf = self.ax.pcolorfast(np.ones(self.board_size)*0.34, cmap=plt.cm.Greens, vmin=0, vmax=0.5)
self.ax.grid()
self.sc1 = self.ax.scatter([], [], c='black', edgecolors='gray', marker='o', s=400)
self.sc2 = self.ax.scatter([], [], c='white', edgecolors='gray', marker='o', s=400)
簡単にコードの補足をします。
__init__ で、ヒント用のAlphaZeroを追加しています。しれっとヒント用のMCTSだけシミュレーション数を100に増やしているのは、勝ちたい気持ちの表れです。
_onkey で、ヒントを表示する処理を書いています。このあたりのコードはViewerの時と同じです。
gameui = GameUI(is_human_first=True)
さあ、いざ勝負のときです!
下記は倍速で表示しています。
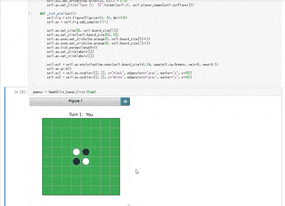
おわりに
今回はおふざけな題材で、Matplotlib でインタラクティブなプロットを作る例について紹介しました。
最後は禁断のヒントモードまでフル活用して、それでも AlphaZero に勝てなかったのですが、
本記事の主目的である、Matplotlib でのインタラクティブなプロットの作り方については、概ね伝えられたのではないかと思います。
私は実際の業務でも、深層強化学習を用いたプロジェクトや画像案件などでこれらの機能を利用したことがあります。特に、深層強化学習をするような場合はデバッグやモデル改善のために、データサイエンティストが自分で可視化できた方が良いでしょう。
知っておいて損はない機能だと思いますので、皆様もぜひ遊んでみて頂ければと思います。