はじめに
Webアプリ作成初挑戦、Qiita初投稿ですm(_ _)m
参考にした投稿
環境
- Mac
- Visual Code
- Python3.7.5
作りたいもの
猫画像を送信したら品種を予測してくれるWebアプリ
(Tensolflowの転移学習モデルInception-v3を用いて猫の品種を予測して
近い品種上位3位を確率を添えて教えてくれるやつ)
環境構築
ファイル構成
├── path/to/cat/image
│ └── (学習データ 猫画像)
├── cat_list.txt(猫の品種リスト)
├── image_process.py
├── model.h5(save_model.pyの出力)
├── save_model.py(転移モデルを再学習する関数)
├── sever.py
├── static
│ └── (入力した画像が保存される)
└── templates
└── index.html
importしたモジュールとバージョン
- Flask 1.1.1
- numpy 1.18.1
- pandas 1.0.1
- tensorflow 2.1.0
- scikit-learn 0.22.2.post1
- opencv-python 4.2.0.32
- Pillow 7.0.0
機械学習モデル構築
###モデル構築のイメージ
###猫画像データセットを用意
The Oxford-IIIT Pet DatasetのDownloadsのDatasetから得ました
犬の画像も含まれています
###モデル構築
Inception-v3を再学習させてmodel.h5を作ります
学習データは200枚/クラス
クラスは以下の12個
- Abyssinian
- Bengal
- Birman
- Bombay
- British_Shorthair
- Egyptian_Mau
- Maine_Coon
- Persian
- Ragdoll
- Russian_Blue
- Siamese
- Sphynx
事前にモデル構築をします
import glob
import numpy as np
import pandas as pd
#kerasのload_imgには手動でpillowのinstall必要
from tensorflow.keras.preprocessing.image import load_img, img_to_array
from tensorflow.keras.applications.inception_v3 import InceptionV3, preprocess_input
from tensorflow.keras.models import Model
from tensorflow.keras.layers import Dense
from sklearn.model_selection import train_test_split
x_size = 299
y_size = 299
kind_label = []
cat_img = []
# 識別する品種のリスト
cat_list = ['Abyssinian','Bengal','Birman','Bombay','British_Shorthair','Egyptian_Mau','Maine_Coon','Persian'
,'Ragdoll','Russian_Blue','Siamese','Sphynx']
f = open('cat_list.txt', 'w')
for x in cat_list:
f.write(str(x) + "\n")
f.close()
#データセットのロード
for cat_kind in cat_list:
file_list = glob.glob(f'path/to/cat/images/{cat_kind}*.jpg')
#print(f"kind: {cat_kind}, num of images: {len(file_list)}")
for cat_file in file_list:
# img_path = file
img = load_img(cat_file, target_size=(x_size, y_size))
x = img_to_array(img)
x = preprocess_input(x)
cat_img.append(x)
kind_label.append(cat_kind)
#品種ラベルをダミー化
Y_dummy = pd.get_dummies(kind_label)
X_train, X_test, y_train, y_test = train_test_split(
cat_img, Y_dummy, test_size=0.2, random_state=42)
# model読み込み
model = InceptionV3(weights='imagenet')
# 中間層を出力するモデル
intermediate_layer_model = Model(inputs=model.input, outputs=model.layers[311].output)
# Denseレイヤーを接続
x = intermediate_layer_model.output
x = Dense(1024, activation='relu')(x)
predictions = Dense(len(cat_list), activation='softmax')(x)
# 転移学習モデル
transfer_model = Model(inputs=intermediate_layer_model.input, outputs=predictions)
# 一旦全レイヤーをフリーズ
for layer in transfer_model.layers:
layer.trainable = False
# 最終段のDenseだけ再学習する
transfer_model.layers[312].trainable = True
transfer_model.layers[313].trainable = True
transfer_model.compile(loss='categorical_crossentropy',
optimizer='adam',
metrics=['accuracy'])
#転移学習
transfer_model.fit(np.array(X_train), np.array(y_train), epochs=10,
validation_data=(np.array(X_test), np.array(y_test)))
#精度確認用に出力(必要に応じて)
loss, acc = transfer_model.evaluate(np.array(X_test), np.array(y_test))
print('Loss {}, Accuracy {}'.format(loss, acc))
transfer_model.save("./model.h5")
#Webアプリ
①saver.py
- モデル読み込み
- 入力データ読み込み
- 品種予測(②image_process.py)
- 入力データと予測結果表示(③index.html)
from flask import Flask, render_template, request
from tensorflow.keras.preprocessing.image import load_img
from tensorflow.keras.models import load_model
import numpy as np
from image_process import examine_cat_breeds
from datetime import datetime
import os
import cv2
import pandas as pd
app = Flask(__name__)
# モデル(model.h5)とクラスのリスト(cat_list)を読み込み
model = load_model('model.h5')
cat_list = []
with open('cat_list.txt') as f:
cat_list = [s.strip() for s in f.readlines()]
print('= = cat_list = =')
print(cat_list)
@app.route("/", methods=["GET","POST"])
def upload_file():
if request.method == "GET":
return render_template("index.html")
if request.method == "POST":
# アプロードされたファイルをいったん保存する
f = request.files["file"]
filepath = "./static/" + datetime.now().strftime("%Y%m%d%H%M%S") + ".png"
f.save(filepath)
# 画像ファイルを読み込む
# 画像ファイルをリサイズ
input_img = load_img(filepath, target_size=(299, 299))
# 猫の種別を調べる関数の実行
result = examine_cat_breeds(input_img, model, cat_list)
print("result")
print(result)
no1_cat = result[0,0]
no2_cat = result[1,0]
no3_cat = result[2,0]
no1_cat_pred = result[0,1]
no2_cat_pred = result[1,1]
no3_cat_pred = result[2,1]
return render_template("index.html", filepath=filepath,
no1_cat=no1_cat, no2_cat=no2_cat, no3_cat=no3_cat,
no1_cat_pred=no1_cat_pred, no2_cat_pred=no2_cat_pred, no3_cat_pred=no3_cat_pred)
if __name__ == '__main__':
app.debug = True
app.run(host='localhost', port=5000)
②image_process.py
引数は
- 入力データ(予測したい猫画像)
- モデル(猫モデル)
- クラスリスト(猫の品種リスト)
返り値は予測された品種上位3位と確率が格納された行列
from tensorflow.keras.preprocessing.image import img_to_array
import numpy as np
from tensorflow.keras.applications.inception_v3 import preprocess_input
def examine_cat_breeds(image, model, cat_list):
# 行列に変換
img_array = img_to_array(image)
# 3dim->4dim
img_dims = np.expand_dims(img_array, axis=0)
# Predict class(preds:クラスごとの確率が格納された12×1行列)
preds = model.predict(preprocess_input(img_dims))
preds_reshape = preds.reshape(-1,preds.shape[0])
# cat_list(リスト)を12×1行列に変換
cat_array = np.array(cat_list).reshape(len(cat_list),-1)
# 確率高い順にソートする
preds_sort = preds_reshape[np.argsort(preds_reshape[:, 0])[::-1]]
# 確率の降順に合わせて猫の順番も変える
cat_sort = cat_array[np.argsort(preds_reshape[:, 0])[::-1]]
# preds_reshape と cat_arrayを結合
set_result = np.concatenate([cat_sort, preds_sort], 1)
return set_result[0:3, :]
③index.html
綺麗に実装できませんでした(^_^;)
<!DOCTYPE html>
<html>
<body>
{% if no1_cat %}
<img src="{{filepath}} " border="1"> <br>
予測結果<br>
{{no1_cat}}:{{no1_cat_pred}}<br>
{{no2_cat}}:{{no2_cat_pred}}<br>
{{no3_cat}}:{{no3_cat_pred}}<br>
<hr>
{% endif %}
ファイルを選択して送信してください<br>
<form action = "./" method = "POST"
enctype = "multipart/form-data">
<input type = "file" name = "file" />
<input type = "submit"/>
</form>
</body>
</html>
実行
python sever.py
+Enterで実行して
http://localhost:5000/
にアクセスするすると以下が表示される
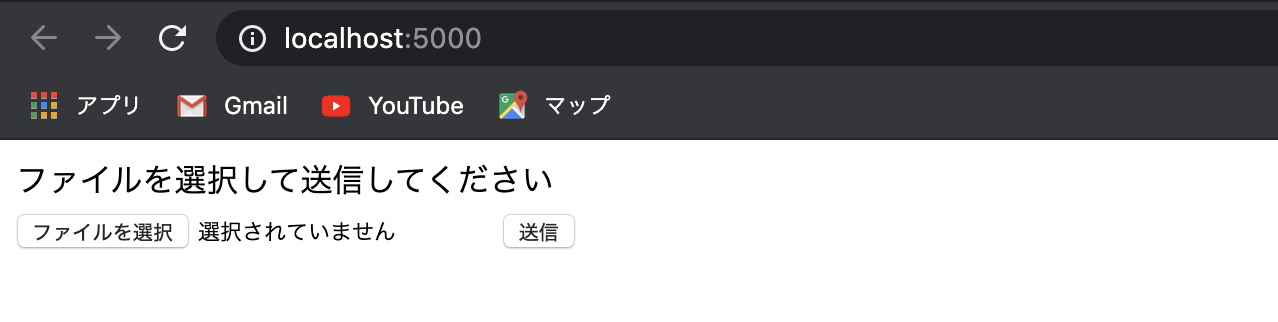
かわいい猫画像を送信すると品種予測結果が表示されます
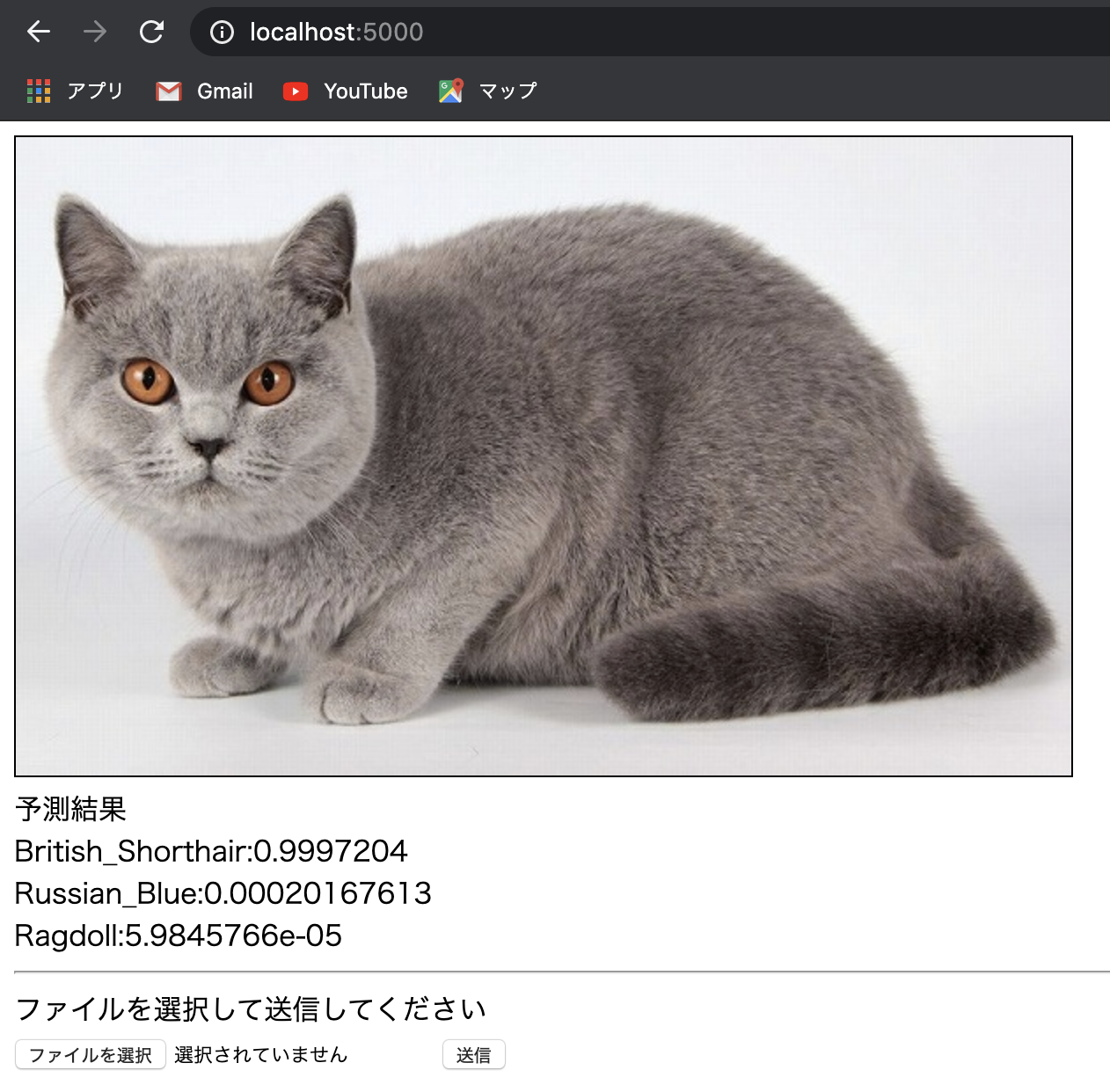
#まとめ
そういえばめちゃくちゃかわいい地域猫はキジ白っぽいんですよね
今回学習したクラスにいない…(^_^;)
キジ白など日本に多い品種の学習データを集める手法を探してみようかな
スクレイピングというやつ?
スマホアプリ化したいなぁ