1 この記事は
株式投資のバックテストを行うクラウドサービスとしてQuantopianがあり高機能ですが、メインは米国株です。(日本株の銘柄データを読み込むことができますが、最新の株価は読み込むことはできません。)
ライブラリZiplineをjupyterにインストールし、日本株のバックテストを行うことを考えましたが、Ziplineの環境設定は簡単ではなく試行錯誤が必要でした。この記事では、Ziplineを使うための環境設定の方法を書きたいと思います。
2 本文
前提条件
Windows10, Jupyter, Anacondaはインストール済み
2-1 python3.5をjupyter仮想環境上にインストールする。
ライブラリziplineは、pythonのversionが3.5以下でないと動作しません。よって、python ver3.5を入手うることが必要です。
JupyterをインストールするとPythonのversionは最新のものがインストールされます(2020年6月現在Python ver3.7がインストールされます)。違うversionのpython (ver3.5)をjupyter上に同居させる場合、仮想環境を構築し、仮想環境上にpython ver3.5をインストールする方法をとりました。仮想環境の構築の方法はこちらの記事をご覧ください。
2-2 仮想環境上にziplineをインストールする
(1)anaconda3プロンプトを立ち上げます。
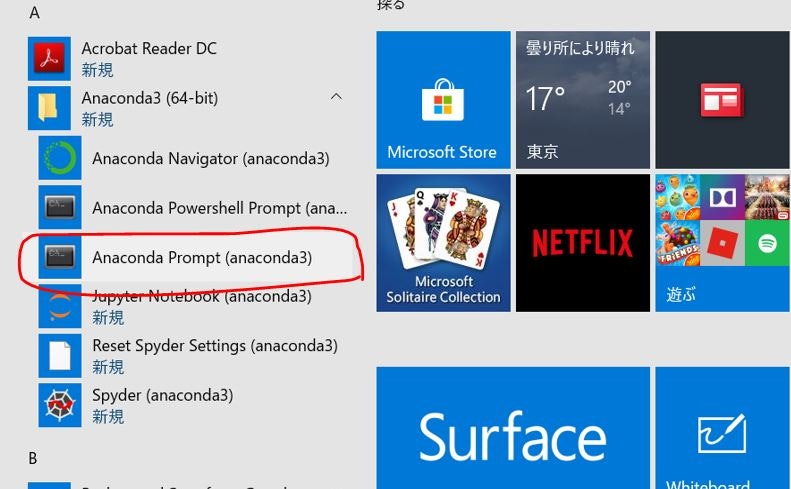
(2)仮想環境python355に切り替えを行います。
(base) C:\Users\***\anaconda3>activate python355 #仮想環境python355に切り替え
(3)仮想環境上でziplineをインストールします。pipよりもcondaを使用した方がインストールが楽です。
(python355) C:\Users\***\anaconda3>>conda install -c Quantopian zipline
2-3 benchmarks.pyとloaders.pyの修正
ziplineはバックテスト実施時にベンチマークデータ(S&P500)を取得しに行きますが、デフォルトのコードでは、2020年6月現在ではすでに使われいないAPI(IEX API)をbenchmarks.pyの中でたたきにいくため、コード実行時にエラー**JSONDecodeError: Expecting value: line 1 column 1 (char 0)**が発生します。
出現するエラーの詳細内容 ・・・リンク先参照
そのため、benchmarks.pyとloaders.pyを修正する必要があります。benchmarks.pyとloaders.pyを下記のとおり書き直してください。
下記のコードをコピペしてbenchmarks.pyに貼り付けてください。
import pandas as pd
from trading_calendars import get_calendar
def get_benchmark_returns(symbol, first_date, last_date):
cal = get_calendar('NYSE')
dates = cal.sessions_in_range(first_date, last_date)
data = pd.DataFrame(0.0, index=dates, columns=['close'])
data = data['close']
return data.sort_index().iloc[1:]
下記のコードをコピペしてloaders.pyに貼り付けてください。
#
# Copyright 2016 Quantopian, Inc.
#
# Licensed under the Apache License, Version 2.0 (the "License");
# you may not use this file except in compliance with the License.
# You may obtain a copy of the License at
#
# http://www.apache.org/licenses/LICENSE-2.0
#
# Unless required by applicable law or agreed to in writing, software
# distributed under the License is distributed on an "AS IS" BASIS,
# WITHOUT WARRANTIES OR CONDITIONS OF ANY KIND, either express or implied.
# See the License for the specific language governing permissions and
# limitations under the License.
import os
import logbook
import pandas as pd
from six.moves.urllib_error import HTTPError
from trading_calendars import get_calendar
from .benchmarks import get_benchmark_returns
from . import treasuries, treasuries_can
from ..utils.paths import (
cache_root,
data_root,
)
logger = logbook.Logger('Loader')
# Mapping from index symbol to appropriate bond data
INDEX_MAPPING = {
'SPY':
(treasuries, 'treasury_curves.csv', 'www.federalreserve.gov'),
'^GSPTSE':
(treasuries_can, 'treasury_curves_can.csv', 'bankofcanada.ca'),
'^FTSE': # use US treasuries until UK bonds implemented
(treasuries, 'treasury_curves.csv', 'www.federalreserve.gov'),
}
ONE_HOUR = pd.Timedelta(hours=1)
def last_modified_time(path):
"""
Get the last modified time of path as a Timestamp.
"""
return pd.Timestamp(os.path.getmtime(path), unit='s', tz='UTC')
def get_data_filepath(name, environ=None):
"""
Returns a handle to data file.
Creates containing directory, if needed.
"""
dr = data_root(environ)
if not os.path.exists(dr):
os.makedirs(dr)
return os.path.join(dr, name)
def get_cache_filepath(name):
cr = cache_root()
if not os.path.exists(cr):
os.makedirs(cr)
return os.path.join(cr, name)
def get_benchmark_filename(symbol):
return "%s_benchmark.csv" % symbol
def has_data_for_dates(series_or_df, first_date, last_date):
"""
Does `series_or_df` have data on or before first_date and on or after
last_date?
"""
dts = series_or_df.index
if not isinstance(dts, pd.DatetimeIndex):
raise TypeError("Expected a DatetimeIndex, but got %s." % type(dts))
first, last = dts[[0, -1]]
return (first <= first_date) and (last >= last_date)
def load_market_data(trading_day=None, trading_days=None, bm_symbol='SPY',
environ=None):
"""
Load benchmark returns and treasury yield curves for the given calendar and
benchmark symbol.
Benchmarks are downloaded as a Series from IEX Trading. Treasury curves
are US Treasury Bond rates and are downloaded from 'www.federalreserve.gov'
by default. For Canadian exchanges, a loader for Canadian bonds from the
Bank of Canada is also available.
Results downloaded from the internet are cached in
~/.zipline/data. Subsequent loads will attempt to read from the cached
files before falling back to redownload.
Parameters
----------
trading_day : pandas.CustomBusinessDay, optional
A trading_day used to determine the latest day for which we
expect to have data. Defaults to an NYSE trading day.
trading_days : pd.DatetimeIndex, optional
A calendar of trading days. Also used for determining what cached
dates we should expect to have cached. Defaults to the NYSE calendar.
bm_symbol : str, optional
Symbol for the benchmark index to load. Defaults to 'SPY', the ticker
for the S&P 500, provided by IEX Trading.
Returns
-------
(benchmark_returns, treasury_curves) : (pd.Series, pd.DataFrame)
Notes
-----
Both return values are DatetimeIndexed with values dated to midnight in UTC
of each stored date. The columns of `treasury_curves` are:
'1month', '3month', '6month',
'1year','2year','3year','5year','7year','10year','20year','30year'
"""
if trading_day is None:
trading_day = get_calendar('NYSE').trading_day
if trading_days is None:
trading_days = get_calendar('NYSE').all_sessions
first_date = trading_days[0]
now = pd.Timestamp.utcnow()
# we will fill missing benchmark data through latest trading date
last_date = trading_days[trading_days.get_loc(now, method='ffill')]
br = ensure_benchmark_data(
bm_symbol,
first_date,
last_date,
now,
# We need the trading_day to figure out the close prior to the first
# date so that we can compute returns for the first date.
trading_day,
environ,
)
tc = ensure_treasury_data(
bm_symbol,
first_date,
last_date,
now,
environ,
)
# combine dt indices and reindex using ffill then bfill
all_dt = br.index.union(tc.index)
br = br.reindex(all_dt, method='ffill').fillna(method='bfill')
tc = tc.reindex(all_dt, method='ffill').fillna(method='bfill')
benchmark_returns = br[br.index.slice_indexer(first_date, last_date)]
treasury_curves = tc[tc.index.slice_indexer(first_date, last_date)]
return benchmark_returns, treasury_curves
def ensure_benchmark_data(symbol, first_date, last_date, now, trading_day,
environ=None):
"""
Ensure we have benchmark data for `symbol` from `first_date` to `last_date`
Parameters
----------
symbol : str
The symbol for the benchmark to load.
first_date : pd.Timestamp
First required date for the cache.
last_date : pd.Timestamp
Last required date for the cache.
now : pd.Timestamp
The current time. This is used to prevent repeated attempts to
re-download data that isn't available due to scheduling quirks or other
failures.
trading_day : pd.CustomBusinessDay
A trading day delta. Used to find the day before first_date so we can
get the close of the day prior to first_date.
We attempt to download data unless we already have data stored at the data
cache for `symbol` whose first entry is before or on `first_date` and whose
last entry is on or after `last_date`.
If we perform a download and the cache criteria are not satisfied, we wait
at least one hour before attempting a redownload. This is determined by
comparing the current time to the result of os.path.getmtime on the cache
path.
"""
filename = get_benchmark_filename(symbol)
data = _load_cached_data(filename, first_date, last_date, now, 'benchmark',
environ)
if data is not None:
return data
# If no cached data was found or it was missing any dates then download the
# necessary data.
logger.info(
('Downloading benchmark data for {symbol!r} '
'from {first_date} to {last_date}'),
symbol=symbol,
first_date=first_date - trading_day,
last_date=last_date
)
try:
data = data = get_benchmark_returns(symbol, first_date, last_date)
data.to_csv(get_data_filepath(filename, environ))
except (OSError, IOError, HTTPError):
logger.exception('Failed to cache the new benchmark returns')
raise
if not has_data_for_dates(data, first_date, last_date):
logger.warn(
("Still don't have expected benchmark data for {symbol!r} "
"from {first_date} to {last_date} after redownload!"),
symbol=symbol,
first_date=first_date - trading_day,
last_date=last_date
)
return data
def ensure_treasury_data(symbol, first_date, last_date, now, environ=None):
"""
Ensure we have treasury data from treasury module associated with
`symbol`.
Parameters
----------
symbol : str
Benchmark symbol for which we're loading associated treasury curves.
first_date : pd.Timestamp
First date required to be in the cache.
last_date : pd.Timestamp
Last date required to be in the cache.
now : pd.Timestamp
The current time. This is used to prevent repeated attempts to
re-download data that isn't available due to scheduling quirks or other
failures.
We attempt to download data unless we already have data stored in the cache
for `module_name` whose first entry is before or on `first_date` and whose
last entry is on or after `last_date`.
If we perform a download and the cache criteria are not satisfied, we wait
at least one hour before attempting a redownload. This is determined by
comparing the current time to the result of os.path.getmtime on the cache
path.
"""
loader_module, filename, source = INDEX_MAPPING.get(
symbol, INDEX_MAPPING['SPY'],
)
first_date = max(first_date, loader_module.earliest_possible_date())
data = _load_cached_data(filename, first_date, last_date, now, 'treasury',
environ)
if data is not None:
return data
# If no cached data was found or it was missing any dates then download the
# necessary data.
logger.info(
('Downloading treasury data for {symbol!r} '
'from {first_date} to {last_date}'),
symbol=symbol,
first_date=first_date,
last_date=last_date
)
try:
data = loader_module.get_treasury_data(first_date, last_date)
data.to_csv(get_data_filepath(filename, environ))
except (OSError, IOError, HTTPError):
logger.exception('failed to cache treasury data')
if not has_data_for_dates(data, first_date, last_date):
logger.warn(
("Still don't have expected treasury data for {symbol!r} "
"from {first_date} to {last_date} after redownload!"),
symbol=symbol,
first_date=first_date,
last_date=last_date
)
return data
def _load_cached_data(filename, first_date, last_date, now, resource_name,
environ=None):
if resource_name == 'benchmark':
def from_csv(path):
return pd.read_csv(
path,
parse_dates=[0],
index_col=0,
header=None,
# Pass squeeze=True so that we get a series instead of a frame.
squeeze=True,
).tz_localize('UTC')
else:
def from_csv(path):
return pd.read_csv(
path,
parse_dates=[0],
index_col=0,
).tz_localize('UTC')
# Path for the cache.
path = get_data_filepath(filename, environ)
# If the path does not exist, it means the first download has not happened
# yet, so don't try to read from 'path'.
if os.path.exists(path):
try:
data = from_csv(path)
if has_data_for_dates(data, first_date, last_date):
return data
# Don't re-download if we've successfully downloaded and written a
# file in the last hour.
last_download_time = last_modified_time(path)
if (now - last_download_time) <= ONE_HOUR:
logger.warn(
"Refusing to download new {resource} data because a "
"download succeeded at {time}.",
resource=resource_name,
time=last_download_time,
)
return data
except (OSError, IOError, ValueError) as e:
# These can all be raised by various versions of pandas on various
# classes of malformed input. Treat them all as cache misses.
logger.info(
"Loading data for {path} failed with error [{error}].",
path=path,
error=e,
)
logger.info(
"Cache at {path} does not have data from {start} to {end}.\n",
start=first_date,
end=last_date,
path=path,
)
return None
def load_prices_from_csv(filepath, identifier_col, tz='UTC'):
data = pd.read_csv(filepath, index_col=identifier_col)
data.index = pd.DatetimeIndex(data.index, tz=tz)
data.sort_index(inplace=True)
return data
def load_prices_from_csv_folder(folderpath, identifier_col, tz='UTC'):
data = None
for file in os.listdir(folderpath):
if '.csv' not in file:
continue
raw = load_prices_from_csv(os.path.join(folderpath, file),
identifier_col, tz)
if data is None:
data = raw
else:
data = pd.concat([data, raw], axis=1)
return data
上記情報の出典先はこちらを参照くださいです。
2-4 銘柄データの取得
バックテストを実施するには銘柄データが必要です。ziplineのDocumentではQuandle APIを使って株価データを取得しておりますが、無料アカウントの場合2018年以降のデータは取得できないようです。よって、ziplineを使う上で銘柄データは自分で用意するのがベストであると思われます。ziplineでは、「bundle」で銘柄データを管理しております。「bundle」にデータセットが登録されていないとバックテスト時に銘柄データを読み出すことができませんので、「bundle」へのデータ登録は必須です。
(python355) C:\Users\xxxxx\anaconda3>zipline bundles #ziplineが管理するbundle
csvdir <no ingestions>
custom-csvdir-bundle 2020-06-13 11:56:14.025797
custom-csvdir-bundle 2020-06-13 11:50:49.932352
custom-csvdir-bundle 2020-06-13 11:47:58.074644
quandl 2020-06-13 03:54:52.594770
quandl 2020-05-26 11:58:22.253861
quantopian-quandl 2020-06-13 07:46:19.699674
(1)銘柄データをcsv形式で用意する。
/.zipline/dataの下にフォルダを作り、銘柄データ(csvデータ形式)を入れます。date,open,high,low,close,volume,dividend,splitの順番の列名を持つデータ構造であることが必要です。
銘柄番号1570(日経レバ,期間:2020/6/1-2020/6/5)を登録bundleに登録してみます。
下記が期間:2020/6/1-2020/6/5における銘柄番号1570(日経レバ)の銘柄データです。
date,open,high,low,close,volume,dividend,split
2020/6/1,18490,18870,18440,18700,11776152,0,1
2020/6/2,18880,19280,18790,19170,12187992,0,1
2020/6/3,19900,20000,19370,19650,15643729,0,1
2020/6/4,20100,20120,19440,19760,15125070,0,1
2020/6/5,19660,20070,19510,20060,9999219,0,1
(2)各取引所のカレンダーをインストールする。
ライブラリの詳細内容はこちらをご覧ください。
(python355) C:\Users\fdfpy\anaconda3>pip install trading-calendars
(3)extension.pyの書き換え
./ziplineの下のextension.pyを下記ソースコードのように書き換えてください。
import pandas as pd
from zipline.data.bundles import register
from zipline.data.bundles.csvdir import csvdir_equities
from zipline.utils.calendars import register_calendar
from pandas import Timestamp
register(
'custom-csvdir-bundle', #bundle名を書く
csvdir_equities(
['test'], #銘柄データcsv形式が格納されているフォルダ名
'C:/Users/fdfpy/.zipline/data/', #「銘柄データcsv形式が格納されているフォルダ名」の上位パス
),
calendar_name='XTKS', #取引所のカレンダーを指定default NYSE , Tokyo:'XTKS'
)
(3)bundleの登録
上記の銘柄株価を'custom-csvdir-bundle'に登録します。銘柄番号1570日経レバはsid番号0と採番されたことが分かります。
(python355) C:\Users\fdfpy\anaconda3>zipline ingest -b custom-csvdir-bundle
| n1570: sid 0 #銘柄番号1570日経レバ
| test: sid 1
3 バックテストを行ってみる
バックテストというほどではありませんが、毎日大引けで日経レバを1株買うというコードを書いて実際にrunさせてみたいと思います。
%reload_ext zipline #ziplineコマンドを読み込みます(%はマジックコマンドを示す)
from zipline.api import order, record, symbol
def initialize(context):
pass
def handle_data(context, data):
order(symbol('n1570'), 1) #毎日大引けで日経レバを1株買う。
record(N1570=data[symbol('n1570')].price) #銘柄名n1570の株価情報等を書き出す。
# 実際に上記のコードを走らせる(%はマジックコマンドを示す)
%zipline --bundle custom-csvdir-bundle --start 2020-6-1 --end 2020-6-5 -o start.pickle
下記に上記コードの出力結果を張り付けています。毎日大引けで1株ずつ購入していることが分かります。
ziplineをインストールしてHello worldができるまでいろいろとカットアンドトライしながらやってみましたが、やっとHello worldまでたどり着けました。今からはもっと複雑なバックテスト等を試すことができそうで楽しみです。