はじめに
Neural Style Transfer を PyTorch で動かすという趣旨の記事であり、
研究の背景をアカデミックに解説している記事ではありません。
備忘録用ににNeural Style Transferの内容を説明し、実装にうつります。
* 導入に興味がないという方は後半の実装から確認し、実際にコードを動かして貰えると楽しめると思います
[参考]
PythonとKerasによるディープラーニング
Deep Learning: Advanced Computer Vision
PyTorch for Deep Learning and Computer Vision
目次
- 導入 : Neural Style Transfer とは
- 誤差関数
- 実装 : PyTorch
導入 : Neural Style Transfer の確認
ニューラルスタイル変換とはターゲット画像のコンテンツを維持した上で、リファレンス画像のスタイルをターゲット画像に適用するというもの。
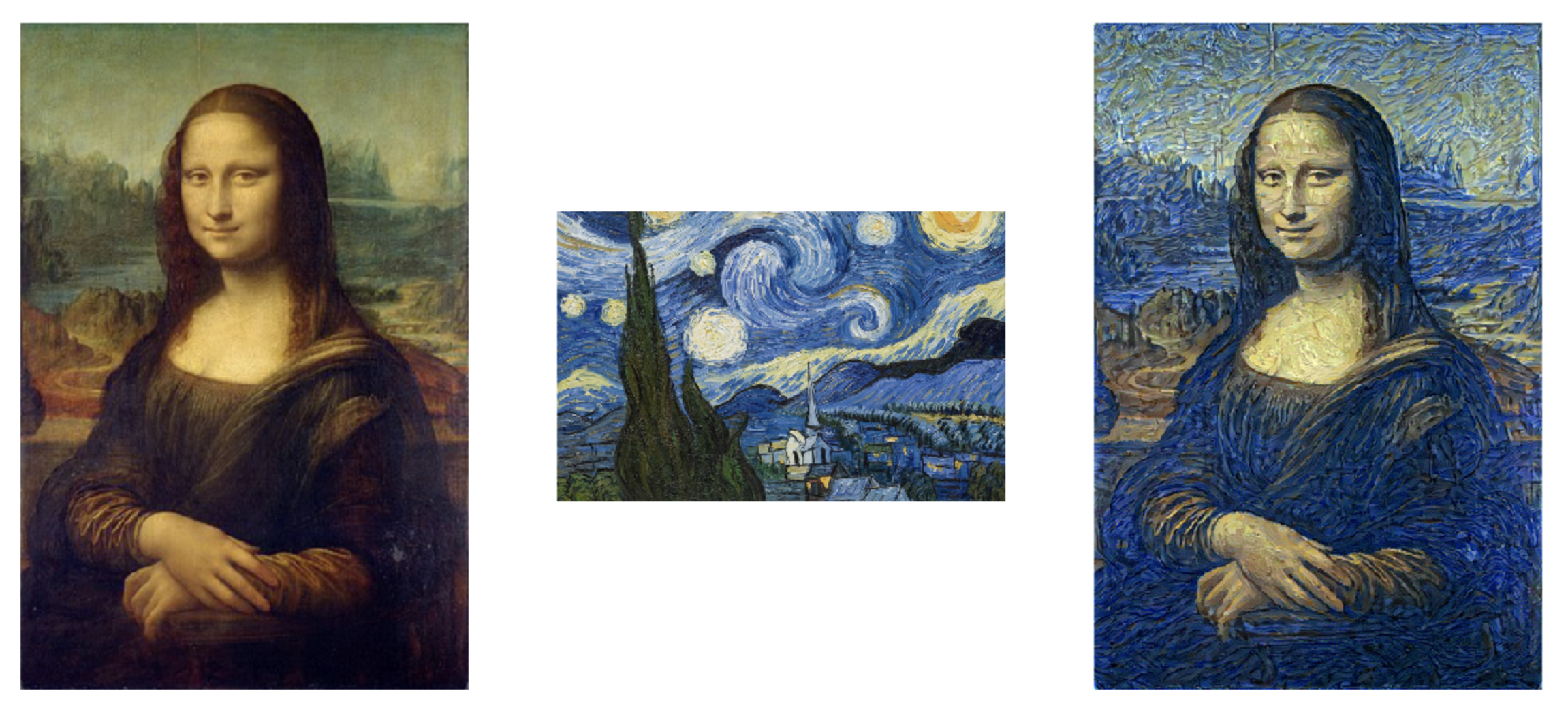
__style__は様々な空間規模での画像のテクスチャ、色、視覚パターンを意味する。
__content__は画像の俯瞰的なマクロ構造を意味。
スタイル変換を実装する時の主な考え方は、ディープラーニングアルゴリズムの中心にある考え方と同じであり、何を達成したいのかを指定するための損失関数を定義し、この損失関数を最小化する。
何を達成したいか : 元のコンテンツ画像のコンテンツを維持した上でリファレンス画像のスタイルを取り入れる。
損失関数
loss = distance(style(reference_image)) - style(generated_image)) +
distance(content(original_image)) - content(generated_image))
ここでdistanceはL2ノルムなどのノルム関数。
__content__は画像からそのコンテンツの表現を計算する関数であり、__style__は画像からそのスタイルの表現を計算する関数である。
>この損失関数を最小化するとstyle(generated_image)がstyle(reference_image)に近づき、content(generated_image)がcontent(reference_image)に近づく。
```math
\mathcal{L}_{total} = \alpha\mathcal{L}_{content} + \beta\mathcal{L}_{style}
上記が最小化するべき損失関数。
[参考・引用]
PythonとKerasによるディープラーニング
誤差関数
Contentの損失関数
CNNの出力側の層の表現は画像のコンテンツをより大域的かつ抽象的に捕捉したものになることが期待される。
CNNにおいて入力に近い層は画像の「局所的な」情報が含まれ、出力側に近づくほど「大域的で抽象的な」情報が含まれる
コンテンツの損失関数 = L2ノルム
すなわちcontent画像で計算された出力側の層と、生成された画像ので計算された同じ層の活性化との間の距離。
Styleの損失関数
スタイルの損失関数は複数層を使用。スタイルの損失関数はグラム行列を使用し、与えられた特徴マップ同士の内積を計算する。
この内積についてはその層の特徴量同士の相関関係を表す。
スタイルの損失関数の目的はスタイル画像と生成された画像とで様々な層の活性化に含まれる相関関係を同じに保つことになる。それにより特定の空間規模で抽出されたテクスチャが、スタイル画像でも同じように見える。
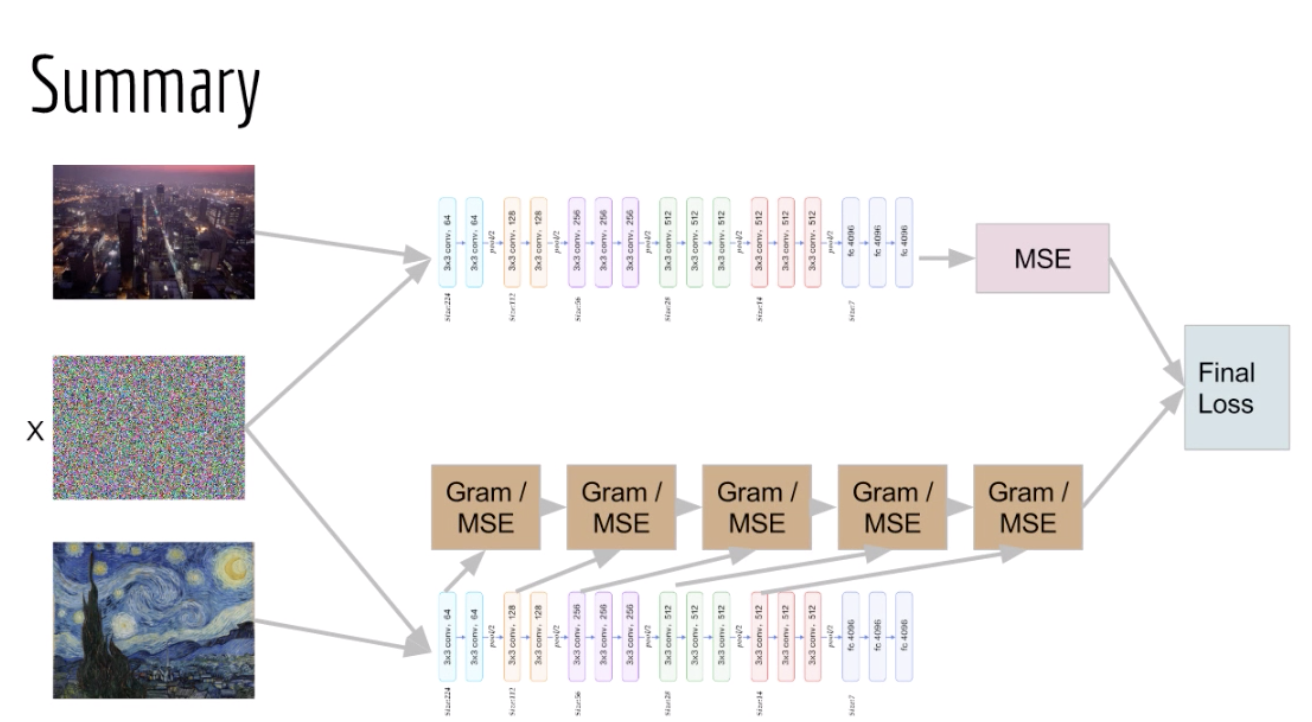
[参考・引用]
PythonとKerasによるディープラーニング
Keras: CNN中間層出力の可視化
Deep Learning: Advanced Computer Vision
実装 : PyTorch
実際に動かしたい方はcontentとstyleに用いたい画像のパスを入力してください。
import matplotlib.pyplot as plt
%matplotlib inline
import torch
import torch.optim as optim
from torchvision import transforms, models, utils
from PIL import Image
import numpy as np
# 逆伝搬の際に影響を受けないように
vgg = models.vgg19(pretrained=True).features
for param in vgg.parameters():
param.requires_grad_(False)
# GPUが使えるときは使用し、不可の際はCPU
device = torch.device('cuda' if torch.cuda.is_available() else 'cpu')
vgg.to(device)
def load_image(img_path, max_size=400, shape=None):
"""
note : image画像の編集
----------
max_size : max_size of image
shape : shape of the tensor
----------
"""
image = Image.open(img_path).convert('RGB')
if shape is not None:
size = shape
if max(image.size) > max_size:
size = max_size
else:
size = max(image.size)
in_transform = transforms.Compose([
transforms.Resize(size),
transforms.ToTensor(),
transforms.Normalize((0.5, 0.5, 0.5),
(0.5, 0.5, 0.5))
])
image = in_transform(image).unsqueeze(0)
return image
content = load_image('Images/MonaLisa.jpg').to(device)
style = load_image('Images/StarryNight.jpg', shape=content.shape[-2:]).to(device)
def im_convert(tensor):
"""
note : tensor to numpy
----------
tensor : tensor
----------
"""
image = tensor.clone().detach().numpy()
# squeeze : Returns a tensor with all the dimensions of input of size 1 removed.
image = image.squeeze()
image = image.transpose(1, 2, 0)
image = image * np.array((0.5, 0.5, 0.5)) + np.array((0.5, 0.5, 0.5))
image = image.clip(0, 1)
return image
# 用いる画像の可視化(確認)
fig, (ax1, ax2) = plt.subplots(1, 2, figsize=(20, 10))
ax1.imshow(im_convert(content))
ax1.axis('off')
ax2.imshow(im_convert(style))
ax2.axis('off')
def get_features(image, model):
"""
note : CNNの特定層から特徴量を抽出する関数
----------
image : tensor
model : type of CNN(今回はVGG19)
----------
"""
layers = {
"0" : "conv1_1",
"5" : "conv2_1",
"10" : "conv3_1",
"19" : "conv4_1",
"21" : "conv4_2",
"28" : "conv5_1",
}
features = {}
#odict_items([('0', Conv2d(3, 64, kernel_size=(3, 3), stride=(1, 1), padding=(1, 1))), ('1', ReLU...
for name, layer in model._modules.items():
image = layer(image)
if name in layers:
# {'conv1_1': tensor([...
features[layers[name]] = image
return features
content_features = get_features(content, vgg)
style_features = get_features(style, vgg)
def gram_matrix(tensor):
"""
note : グラム行列の計算(X.T @ X)
----------
tensor : tensor
----------
"""
# arbitrary vector times vector.T
_, d, h, w = tensor.size()
tensor = tensor.view(d, h*w)
gram = torch.mm(tensor, tensor.t())
return gram
style_grams = {layer : gram_matrix(style_features[layer]) for layer in style_features}
style_weights = {
"conv1_1" : 1.,
"conv2_1" : 0.75,
"conv3_1" : 0.2,
"conv4_1" : 0.2,
"conv5_1" : 0.2
}
content_weight = 1
style_weight = 1e6
# target imgの最適化
target = content.clone().requires_grad_(True).to(device)
# 300iterlationごとに可視化
show_every = 300
optimizer = optim.Adam([target], lr=0.003)
steps = 2100
heights, widths, channels = im_convert(target).shape
image_array = np.empty(shape=(300, heights, widths, channels))
capture_frame = steps / 300
counter = 0
for ii in range(1, steps+1):
target_features = get_features(target, vgg)
content_loss = torch.mean((target_features['conv4_2'] - content_features['conv4_2']) **2)
style_loss = 0
# layerは辞書のキー
for layer in style_weights:
target_feature = target_features[layer]
target_gram = gram_matrix(target_feature)
style_gram = style_grams[layer]
layer_style_loss = style_weights[layer] * torch.mean((target_gram - style_gram) **2)
_, d, h, w = target_feature.shape
# unit lossを得るためにnormalizeする必要がある
style_loss += layer_style_loss / (d * h * w)
total_loss = content_weight * content_loss + style_weight * style_loss
optimizer.zero_grad()
total_loss.backward()
optimizer.step()
if ii % show_every == 0:
print('total loss' , total_loss.item())
print('iterlation' , ii)
plt.imshow(im_convert(target))
plt.show()
if ii % capture_frame == 0:
image_array[counter] = im_convert(target)
counter = counter + 1
# content, style, targetの全画像の可視化
fig, (ax1, ax2, ax3) = plt.subplots(1, 3, figsize=(20,10))
ax1.imshow(im_convert(content))
ax1.axis('off')
ax2.imshow(im_convert(style))
ax2.axis('off')
ax3.imshow(im_convert(target))
# target画像の保存
utils.save_image(target, 'target.png', nrow=4)
ax3.axis('off')
[参考]
PyTorch for Deep Learning and Computer Vision
おわりに
今回はPytorchに慣れるためにNeural Style Transferの実装を行った。