RedSVD
RedSVD は修正BSDライセンスで公開されている行列分解ライブラリです.
C++の線形代数ライブラリである Eigen を利用しており,簡単に利用することができます.
Eigenは疎行列を扱うためのクラスを提供していますが(SparseMatrix),特異値分解は密行列のみにしか対応しておりません.
RedSVDは SparseMatrix の特異値分解を計算することができるため,非常に便利です.
Wikiによるとheaderだけの RedSVDも公開されているため,今回はこちらのheaderを用いて実装してみたいと思います.
headerは /usr/local/include などに配置すれば利用することができます.
また,RedSVDや特異値分解の詳細は以下の記事を御覧ください.
DO++ 「行列分解ライブラリredsvdを公開しました」
http://hillbig.cocolog-nifty.com/do/2010/08/redsvd-aa59.html
実装例
実装例として,6×5の疎行列 $M$ の特異値分解を求めるプログラムを実装しました.
具体的には,$M = USV^T$ を満たす行列 $U$,$S$,$V$を求めます.
行列 $M$ は以下の通りです.
1 0 1 0 0
0 1 0 0 0
1 1 0 0 0
1 0 0 0 1
1 0 0 0 1
0 0 0 1 0
Eigenでは,Eigen::Triplet
を用いて疎行列 SparseMatrix
を作成することが一般的です.
vector<Eigen::Triplet<value_type>>
に非零要素の位置と値を挿入し,SparseMatrix
に対して setFromTriplets() を実行します.
このようにして疎行列を作成すれば,あとは RedSVD のコンストラクタを用いるだけで行列 $U$,$S$,$V$ を求めることができます.
RedSVD::RedSVD<Eigen::SparseMatrix<double>> svd(M);
コンストラクタ内の compute() によって既に特異値分解が計算されているので,あとは matrixU() といった関数で行列を取り出すだけです.
行列 $U$ は matrixU(),行列 $S$ は singularValues().asDiagonal(),行列 $V$ は matrixV() を実行します.
ここで注意なのですが,singularValues() は特異値が格納されたベクトルが得られるため,asDiagonal() 関数を用いて対角行列に変換する必要があります.
以上を踏まえ,疎行列 $M$ の特異値分解を求めるプログラムを以下に示します.
# include <iostream>
# include <vector>
# include <Eigen/Sparse>
# include <Eigen/SVD>
# include <RedSVD/RedSVD-h>
using namespace std;
using T = Eigen::Triplet<double>;
int main()
{
Eigen::SparseMatrix<double> M(6, 5);
vector<T> t;
t.push_back(T(0, 0, 1));
t.push_back(T(0, 2, 1));
t.push_back(T(1, 1, 1));
t.push_back(T(2, 0, 1));
t.push_back(T(2, 1, 1));
t.push_back(T(3, 0, 1));
t.push_back(T(3, 4, 1));
t.push_back(T(4, 0, 1));
t.push_back(T(4, 4, 1));
t.push_back(T(5, 3, 1));
M.setFromTriplets(begin(t), end(t));
cout << M << endl;
RedSVD::RedSVD<Eigen::SparseMatrix<double>> svd(M);
Eigen::MatrixXd U = svd.matrixU();
Eigen::MatrixXd S = svd.singularValues().asDiagonal();
Eigen::MatrixXd V = svd.matrixV();
cout << "U: " << endl << U << endl;
cout << "S: " << endl << S << endl;
cout << "V: " << endl << V << endl;
cout << "M_approx: " << endl << U*S*V.transpose() << endl;
}
実行結果
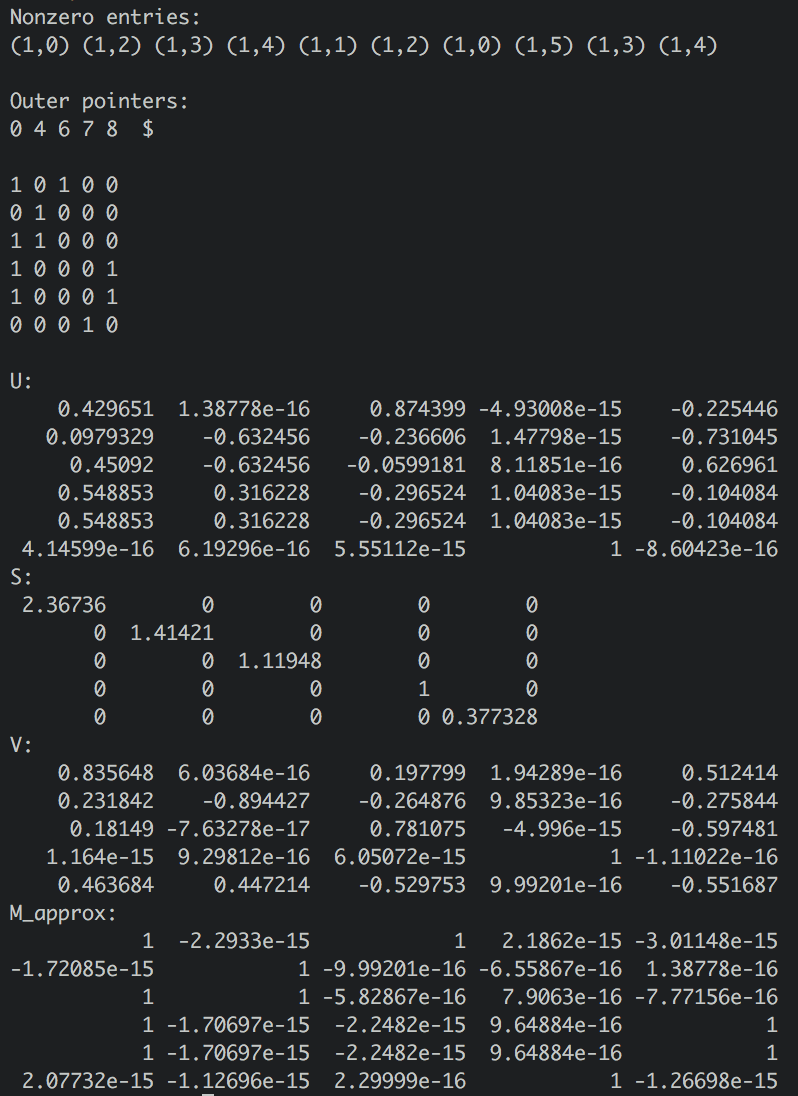
まとめ
RedSVD を用いることで,簡単に疎行列の特異値分解を計算することができました.
今回の例では小さな行列に対して処理を行いましたが,より大きな行列に対しても実行可能です.
少しでも実験や開発の参考になれば幸いです.