こちらの続きです。
MLflowでLLMを記録できるということは、MLflowのモデルサービングを使ってLLMをサービングできるのでは、そして、streamlitと組み合わせたらチャットボットを動かせるのではと思い立ちました。結論、いけました。
ここで使う用語をまとめておきます。
- モデルサービング: REST API経由でアクセスできるように機械学習モデルをホスティングする機能。
- モデルレジストリ: 機械学習モデルを集中管理するためのハブのようなものです。バージョン管理も可能です。
- エンドポイント: 機械学習モデルのREST APIエンドポイントです。
LLMのロギング
以下のようなコードを実行します。
import transformers
import mlflow
chat_pipeline = transformers.pipeline(model="microsoft/DialoGPT-medium")
with mlflow.start_run():
model_info = mlflow.transformers.log_model(
transformers_model=chat_pipeline,
artifact_path="chatbot",
input_example="Hi there!"
)
これでHugging Faceで公開されているLLMがロギングされます。
MLflowランの画面にアクセスして、モデルレジストリにモデルを登録します。モデルをサービングするにはモデルレジストリに登録する必要があります。
REST APIエンドポイントの作成
注意
以降では、サーバレスリアルタイム推論を用いたDatabricksのモデルサービングで説明されているサーバレスエンドポイントを使用しています。この記事の執筆時点では日本リージョンではまだ利用できません。
サイドメニューのサービングにアクセスし、エンドポイントを作成します。少し待つとモデルがサービングされるようになります。そうすると、REST API経由でモデル、すなわちLLMにアクセスできるようになります。アクセスする際にはパーソナルアクセストークンが必要になります。
なお、この時点でも動作確認が可能です。右上のQuery endpointをクリックして、エンドポイントにリクエストを投げることができます。
GUIの作成
こちらで行ったのと同じアプローチで、streamlitでチャットボットのGUIを作成します。
import streamlit as st
import numpy as np
from PIL import Image
import base64
import io
import os
import requests
import numpy as np
import pandas as pd
import json
#st.title('Chatbot')
st.header('Chatbot on Databrikcs')
#st.write('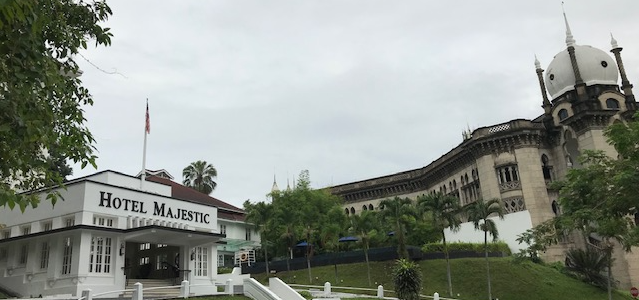')
st.write('''
- [MLflow 2\.3のご紹介:ネイティブLLMのサポートと新機能による強化 \- Qiita](https://qiita.com/taka_yayoi/items/431fa69430c5c6a5e741)
- [MLflow 2\.3のHugging Faceトランスフォーマーのサポートを試す \- Qiita](https://qiita.com/taka_yayoi/items/ad370a7f57c4eae58800)
''')
def score_model(prompt):
# 1. パーソナルアクセストークンを設定してください
# 今回はデモのため平文で記載していますが、実際に使用する際には環境変数経由で取得する様にしてください。
token = "<personal access token>"
#token = os.environ.get("DATABRICKS_TOKEN")
# 2. モデルエンドポイントのURLを設定してください
url = '<endpoint url>'
headers = {'Authorization': f'Bearer {token}'}
#st.write(token)
data_json_str = f"""
{{
"inputs": [
[
"{prompt}"
]
]
}}
"""
#st.write(data_json_str)
data_json = json.loads(data_json_str)
response = requests.request(method='POST', headers=headers, url=url, json=data_json)
if response.status_code != 200:
raise Exception(f'Request failed with status {response.status_code}, {response.text}')
return response.json()
prompt = st.text_input("プロンプト")
if prompt != "":
response = score_model(prompt)
st.write(response['predictions'])
今回はローカルマシンで起動します。
streamlit run chatbot.py
今回使用しているサーバレスエンドポイントは、リクエストがない場合には計算資源がゼロにまでスケールダウンするので経済的です。そして、エンドポイントではリクエストの状況などをモニタリングすることもできます。
モデルの改善も進めていきますが、足回りも整っていく感じがして良いですね。