はじめに
pythonで $n$ 本腕バンディットタスクを実装しました.
教科書として『強化学習』を使いました.
本記事の構成
- はじめに
- 強化学習
- 概要
- 構成要素
- バンディットタスク
- ルール
- 標本平均手法
- 行動選択規則
- 実装
- 結果
- おわりに
強化学習
概要
強化学習は,報酬を最大にするためにどの行動を選択すべきかを学習します.
教師あり学習では,選択すべき正解の行動を与えられますが,強化学習では,「ある指針」に基づいて行動を選択し,それにより得られる報酬を使って,行動を評価・更新します.
学習された「ある指針」に従って行動することで,報酬を最大化できます.
構成要素
強化学習の構成要素とその簡単な説明をします.
- エージェント:周囲の環境を感知し,環境と直接相互作用する個体
- 環境:エージェントと独立した周囲の状況
- 報酬:エージェントが行動することで環境から得られる値
- 価値関数:エージェントの現在の状況を基点として将来にわたって蓄積することのできる報酬の総量
エージェントは,環境を感知し,感知した状態と価値関数に基づいて行動を選択します.
行動に対して環境から報酬がフィードバックされ,この報酬を使って行動の価値を更新します.
バンディットタスク
ルール
$n$ 本のレバーを持つスロットマシンを $n$ 本腕バンディットと呼びます.$n$ 本のレバーの中から $1$ 本を選び,そのレバーを引くと,賞金が得られます.
まず,平均 $0$ 分散 $1$ のガウス分布から,ある行動 $a$ の報酬 $Q^{\ast}(a)$ を生成します.次に,平均 $Q^{\ast}(a)$ 分散 $1$ のガウス分布から,バンディットマシンから得られる報酬を生成します.
このバンディットマシンを複数台生成し,すべてのバンディットマシンを $1$ 回ずつ引くことを $1$ プレイとします.複数プレイを通して学習することで,報酬の最大化を図ります,
標本平均手法
ある行動が選ばれたときに得られた報酬を平均化することで,その行動の価値を推定できます.これを標本平均手法と呼びます.
行動 $a$ の真の価値を $Q^{\ast}(a)$ とし,$t$ 番目のプレイでの推定量を $Q_t(a)$ とします.
$t$ 回目のプレイにおいて $a$ という行動が $k_a$ 回選択され,それぞれの選択における報酬が $r_{1}, r_{2},..., r_{k_a}$ とすると,
行動 $a$ の $t$ 回目までの価値は以下のように定義されます.
Q_{t}(a) = \frac{r_{1} + r_{2} + \cdots + r_{k_a}}{k_a}
$k_a \rightarrow \infty$ において,大数の法則より $Q_t(a) \rightarrow Q^{\ast}(a)$ に収束します.
行動選択規則
行動選択規則として,グリーディ手法および $\varepsilon$ グリーディ手法を説明します.
グリーディ手法では,最も高いと推定された行動価値をもつ行動を選択します.つまり,$t$ 回目のプレイにおいて,$Q_t(a^{\ast}) = \max_{a}Q_t(a)$ となるようなグリーディな行動の $1$ つ $a^{\ast}$ を選択します.
$\varepsilon$ グリーディ手法では,$\varepsilon$ の確率でランダムに行動し,$1 - \varepsilon$ の確率でグリーディな行動を選択します.
グリーディ手法では,常に最良の価値をもつ行動を選択し,それ以外の行動を試すことは一切ありません.
これは,試すことのない行動の中に,現在最良としている行動よりもさらに良い行動があったとしても,それを見つけられないことを意味します.
一方 $\varepsilon$ グリーディ手法では,基本的にグリーディに行動選択するが,たまに $\varepsilon$ の確率でランダムに行動します.これにより,現在最良としている行動よりもさらに良い行動を見つけられるかもしれません.
実装
以下のように実装しました.
$10$ 本腕のバンディットマシンを $2000$ 台用意し,$2000$ プレイを通して学習しました.
ここでは,$2000$ 台すべてのマシンを $1$ 回ずつ引くことを $1$ プレイとします.
(教科書のルールを一部変えているため,結果が多少異なります)
import numpy
from matplotlib import pyplot
import random
import sys
class Bandit:
# constuctor
def __init__(self, n_machine, n_action):
for i in range(n_action):
_qstar = numpy.random.normal(0.0, 1.0)
_machine = numpy.random.normal(_qstar, 1.0, n_machine).reshape((-1, 1))
if i == 0:
self.machine = _machine
else:
self.machine = numpy.hstack((self.machine, _machine))
# public method
def play(self, n_play, epsilon):
self.q = numpy.zeros(self.machine.shape)
self.q_count = numpy.zeros(self.machine.shape)
average_reward = numpy.zeros(n_play)
n_machine = self.machine.shape[0]
for _p in range(n_play):
total = 0.0
for mac_index in range(n_machine):
act_index = self.__select_action(mac_index, epsilon)
reward = self.machine[mac_index, act_index]
total += reward
self.__update_qtable(reward, mac_index, act_index)
average_reward[_p] = total / n_machine
self.__display(_p, average_reward[_p])
return average_reward
# private method
def __select_action(self, mac_index, epsilon):
if numpy.random.rand() > epsilon:
act_index = self.__select_greedy_action(mac_index)
else:
act_index = self.__select_random_action()
return act_index
def __select_greedy_action(self, mac_index):
_max = self.q[mac_index, :].max()
indexes = numpy.argwhere(self.q[mac_index, :] == _max)
random.shuffle(indexes)
return indexes[0]
def __select_random_action(self):
return numpy.random.randint(10)
def __update_qtable(self, reward, mac_index, act_index):
_q = self.q[mac_index, act_index]
self.q_count[mac_index, act_index] += 1
self.q[mac_index, act_index] = _q + (reward - _q) / self.q_count[mac_index, act_index]
def __display(self, play, average_reward):
if (play + 1) % 100 == 0:
print u'play: %d, average reward: %f' % (play + 1, average_reward)
from bandit import *
if __name__ == '__main__':
# param
param = sys.argv
# init
n_machine = 2000
n_action = 10
n_play = 2000
epsilon = [0.0, 0.01, 0.1, 1.0]
# draw init
mergin = 5
color = ['b', 'g', 'r', 'k']
pyplot.figure(figsize = (8, 6))
pyplot.xlim(-mergin, n_play + mergin)
# bandit machine
bandit = Bandit(n_machine, n_action)
# play
for i in range(len(epsilon)):
print u'play count: %d, epsilon: %.2f' % (n_play, epsilon[i])
average_reward = bandit.play(n_play, epsilon[i])
_label = 'e = %.2f' % epsilon[i]
pyplot.plot(numpy.arange(n_play), average_reward, color = color[i], label = _label)
print '!!!finish!!!\n'
# save and show
if '-d' in param or '-s' in param:
pyplot.legend(loc = 'center right')
if '-s' in param:
pyplot.savefig('bandit2.png')
if '-d' in param:
pyplot.show()
結果
以下のような結果が得られました.
$\varepsilon = 0.0$ では,常にグリーディな行動を選択するため,$0$ 以上の価値をもつ行動が見つかった時点で,その行動を常に選択し続けるようになります.そのため,得られる報酬の平均値は常に一定となっています.
$\varepsilon = 1.0$ では,常にランダムに行動するため,プレイ回数が増えていっても得られる報酬の平均値はある一定の域を出ていません.
$\varepsilon = 0.1$ では,$10%$ の確率でランダムに行動し,$90%$ の確率でグリーディな行動を選択します.
最も早く最適な行動を見つけていますが,学習が終わった後でも $10%$ の確率でランダムに行動するため,平均報酬は $1.8$ 程度にとどまっています.
$\varepsilon = 0.01$ では,$1%$ の確率でランダムに行動し,$99%$ の確率でグリーディな行動を選択します.
収束速度は $\varepsilon = 0.1$ に比べて遅くなりますが,$1000$ プレイを超えたあたりから,性能が最も良くなっています.
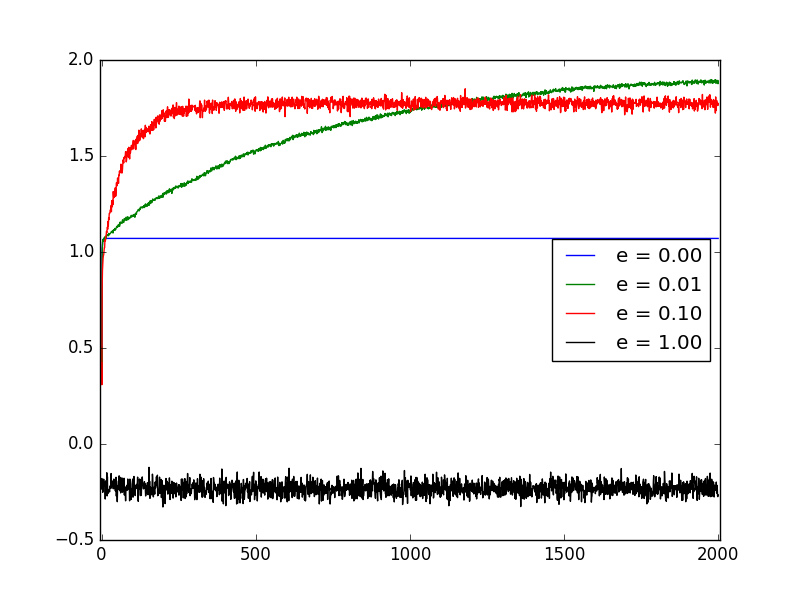
おわりに
$n$ 本腕バンディットタスクをpythonで実装し,$\varepsilon$ の変化による学習過程の違いを確認できました.