本記事では、scikit-learnのv0.23から搭載された、インタラクティブなパイプライン確認の実装、そしてそれをHTML化して保存、活用する方法を解説します。
環境
- scikit-learn==0.23.2
- Google Colaboratory
本記事の実装コードはこちらに置いています
https://github.com/YutaroOgawa/Qiita/tree/master/sklearn
実装
[1] バージョン更新
まず、Google Colaboratoryのscikit-learnのバージョンが2020年9月ではv0.22なので、v0.23へと更新します。
!pip install scikit-learn==0.23.2
pipで更新したあとは、Google Colaboratoryの「ランタイム」→「ランタイムを再起動」を実行し、
ランタイムを再起動します。
(これで、scikit-learnがpipで入れた新しいv0.23になります)
[2] パイプライン構築
例えば、以下のようにして、前処理と機械学習モデルを組み合わせた
機械学習パイプラインを構築します。
[必要なimportを実施]
python
from sklearn.pipeline import make_pipeline
from sklearn.preprocessing import OneHotEncoder, StandardScaler
from sklearn.impute import SimpleImputer
from sklearn.compose import make_column_transformer
from sklearn.linear_model import LogisticRegression
[パイプラインを構築]
```python```
# 数値データの前処理(中央値で欠損値補完して、標準化)
num_proc = make_pipeline(SimpleImputer(strategy='median'), StandardScaler())
# カテゴリデータの前処理(欠損値には"misssing"を代入補完し、ワンホットエンコーディング)
cat_proc = make_pipeline(
SimpleImputer(strategy='constant', fill_value='missing'),
OneHotEncoder(handle_unknown='ignore'))
# 前処理クラスを作成
preprocessor = make_column_transformer((num_proc, ('feat1', 'feat3')),
(cat_proc, ('feat0', 'feat2')))
# 前処理と機械学習モデルを1つのパイプラインにする
clf = make_pipeline(preprocessor, LogisticRegression())
[3] インタラクティブにパイプラインを可視化
インタラクティブにパイプラインを可視化するには、単純で、
sklearn.set_config(display="diagram")
を加えるだけです。
[インタラクティブに可視化]
python
パイプラインを表示させる設定
from sklearn import set_config
set_config(display="diagram")
描画
clf
すると、JupyterNotebook(Google Colabortory)の結果欄に、以下のようにパイプラインが描画されます。
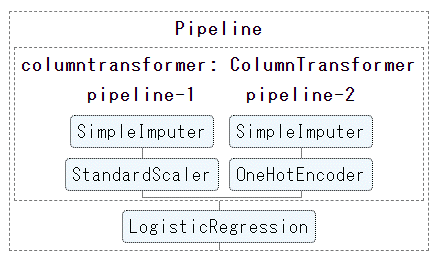
このパイプラインの図の各要素をクリックすると、
インタラクティブに画像が変化し、その要素の詳細設定が表示されます。
(以下の図は、カラム前処理の欠損値処理方法を詳細確認する場合:pipeline-2のSimpleImputerをクリック)
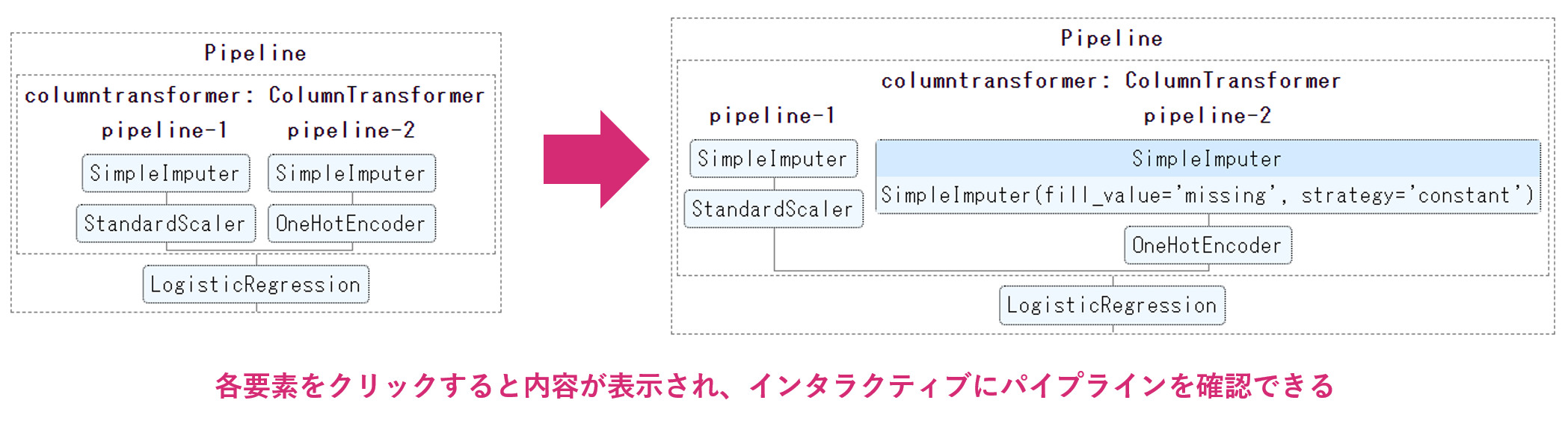
## パイプラインをHTMLとして保存する方法
コメント欄でいただいたように、このインタラクティブなパイプラインをHTML化して保存することができます。
「JupyterNotebook上でしか動かないのでは、ちょっとな・・・」
と思っていたので、非常に嬉しい情報です。
@DataSkywalker さま、誠にありがとうございます。
実装としては最後に、
```python
from sklearn.utils import estimator_html_repr
with open('my_estimator.html', 'w') as f:
f.write(estimator_html_repr(clf))
を実行します。すると、my_estimator.htmlとして、インタラクティブなパイプラインのHTMLが保存されます。
Google Colaboratoryであれば、
# Google Colaboratoryからダウンロード
from google.colab import files
files.download('my_estimator.html')
を実行することで、my_estimator.htmlをダウンロードすることができます
(HTMLファイルにCSSのstyleも含まれ300行ほどの内容でした)。
インタラクティブにパイプラインを説明する資料として、
HTMLをドキュメントなどに貼り付ける、などができそうです。
mdファイルにリンクとして入れても良いですし、無理やりmdファイルをhtml化してから結合しても良いです。
(mdファイルにそのままhtmlを読み込むのは難しい・・・?)
このあたりのファイルもすべて、こちらに置いています
https://github.com/YutaroOgawa/Qiita/tree/master/sklearn
まとめ
scikit-learnのバージョンをv0.23以上にして、
sklearn.set_config(display="diagram")
を加えるだけで、
パイプラインをインタラクティブに可視化(そして、HTMLで保存)することができます。
ぜひお試しください♪
備考
**【執筆者】**電通国際情報サービス(ISID)AIトランスフォーメーションセンター 開発Gr
小川 雄太郎(主書「つくりながら学ぶ! PyTorchによる発展ディープラーニング」 、その他「自己紹介詳細」)
【Twitter】
IT・AI関連やビジネス・経営系を中心に、私が面白いと思った記事や最近読んだ新刊書籍の感想などを発信しています。これらの分野の情報を収集したい方はぜひフォローしてみてください♪(海外情報が多めです)
【その他】
私がリードする、「AIトランスフォーメーションセンター 開発チーム」ではメンバを募集中です。ご興味、ご関心をお持ちの方は、こちらのページから、応募をお待ちしております。
【そくめん君】
いきなり応募は・・・という方は、カジュアル面談を「そくめん君」で行わせていただいております。
こちらもぜひご利用ください♪
https://sokumenkun.com/2020/08/17/yutaro-ogawa/
【免責】本記事の内容そのものは著者の意見/発信であり、著者が属する企業等の公式見解ではございません
(参考)
https://scikit-learn.org/stable/auto_examples/release_highlights/plot_release_highlights_0_23_0.html
https://towardsdatascience.com/9-things-you-should-know-about-scikit-learn-0-23-9426d8e1772c