はじめに
だいたい1年くらい前にChainerで書いたアニメ顔を分類するプログラムですが,今回はKerasで書きました.プログラムはGitHubにあげました.
データセット
データセットはanimeface-character-datasetから入手することができます.
参考:DenoisingAutoEncoderでアニメ顔の特徴を抽出してみた
データセット前処理
前より少しだけ改良しました.32×32のRGBのデータ(shape=(3, 32, 32))にリサイズします.前回と違うのは,データの入っていない空のフォルダを削除しなくても多分ちゃんと動くところです.
Requirementsは
- six
- numpy
- opencv
- progressbar2
となっています.
# ! -*- coding: utf-8 -*-
import os
import six.moves.cPickle as pickle
import numpy as np
try:
import cv2 as cv
except:
pass
from progressbar import ProgressBar
class AnimeFaceDataset:
def __init__(self):
self.data_dir_path = u"./animeface-character-dataset/thumb/"
self.data = None
self.target = None
self.n_types_target = -1
self.dump_name = u'animedata'
self.image_size = 32
def get_dir_list(self):
tmp = os.listdir(self.data_dir_path)
if tmp is None:
return None
ret = []
for x in tmp:
if os.path.isdir(self.data_dir_path+x):
if len(os.listdir(self.data_dir_path+x)) >= 2:
ret.append(x)
return sorted(ret)
def get_class_id(self, fname):
dir_list = self.get_dir_list()
dir_name = filter(lambda x: x in fname, dir_list)
return dir_list.index(dir_name[0])
def get_class_name(self, id):
dir_list = self.get_dir_list()
return dir_list[id]
def load_data_target(self):
if os.path.exists(self.dump_name+".pkl"):
print "load from pickle"
self.load_dataset()
print "done"
else:
dir_list = self.get_dir_list()
ret = {}
self.target = []
self.data = []
print("now loading...")
pb = ProgressBar(min_value=0, max_value=len(dir_list)).start()
for i, dir_name in enumerate(dir_list):
pb.update(i)
file_list = os.listdir(self.data_dir_path+dir_name)
for file_name in file_list:
root, ext = os.path.splitext(file_name)
if ext == u'.png':
abs_name = self.data_dir_path+dir_name+'/'+file_name
# read class id i.e., target
class_id = self.get_class_id(abs_name)
self.target.append(class_id)
# read image i.e., data
image = cv.imread(abs_name)
image = cv.resize(image, (self.image_size, self.image_size))
image = image.transpose(2,0,1)
image = image/255.
self.data.append(image)
pb.finish()
print("done.")
self.data = np.array(self.data, np.float32)
self.target = np.array(self.target, np.int32)
self.dump_dataset()
def dump_dataset(self):
pickle.dump((self.data,self.target), open(self.dump_name+".pkl", 'wb'), -1)
def load_dataset(self):
self.data, self.target = pickle.load(open(self.dump_name+".pkl", 'rb'))
if __name__ == '__main__':
dataset = AnimeFaceDataset()
dataset.load_data_target()
実際に読み込んでみると
In [1]: from animeface import AnimeFaceDataset
In [2]: dataset = AnimeFaceDataset()
In [3]: dataset.load_data_target()
load from pickle
done
In [4]: x = dataset.data
In [5]: y = dataset.target
In [6]: print x.shape, y.shape
(14490, 3, 32, 32) (14490,)
となり,データ数14490,クラス数(キャラクター数)176の分類問題となります.(ここまではほとんど前回と同じです.)
KerasによるConvolutional Neural Networksの実装
モデルの構築
from keras.layers.convolutional import Convolution2D
from keras.layers.convolutional import MaxPooling2D
from keras.layers.core import Activation
from keras.layers.core import Dense
from keras.layers.core import Dropout
from keras.layers.core import Flatten
from keras.models import Sequential
def build_deep_cnn(num_classes=3):
model = Sequential()
model.add(Convolution2D(96, 3, 3, border_mode='same', input_shape=(3, 32, 32)))
model.add(Activation('relu'))
model.add(Convolution2D(128, 3, 3))
model.add(Activation('relu'))
model.add(MaxPooling2D(pool_size=(2, 2)))
model.add(Dropout(0.5))
model.add(Convolution2D(256, 3, 3, border_mode='same'))
model.add(Activation('relu'))
model.add(Convolution2D(256, 3, 3))
model.add(Activation('relu'))
model.add(MaxPooling2D(pool_size=(2, 2)))
model.add(Dropout(0.5))
model.add(Flatten())
model.add(Dense(1024))
model.add(Activation('relu'))
model.add(Dropout(0.5))
model.add(Dense(num_classes))
model.add(Activation('softmax'))
return model
最初にmodel = Sequential()
でシーケンシャルモデルを生成して,それにConvolutional2D
やDense
を追加していくだけでネットワークを構築できます.
Convolutional2D
が畳み込み層,Dense
が全結合層に相当する感じです.
一番最初の,
Convolution2D(96, 3, 3, border_mode='same', input_shape=(3, 32, 32))
だけはinput_shape
を指定する必要があります.
Convolutional2D
について簡単に説明すると,最初の引数が,畳み込みカーネルの枚数,第2,3引数が畳み込みカーネル大きさを指定するものです.border_mode
にはsame
とvalid
の2種類がありますが,same
はpaddingがカーネルの大きさの半分,つまり出力の縦横の大きさは入力の縦横の大きさは変わりません.valid
はpaddingがない状態,つまり出力の縦横の大きさは入力の縦横の大きさよりも小さくなります.paddingについはこちらがわかりやすいです.今回はsame
で畳み込みカーネルのshape
が(96, 3, 3)
,入力のshape
が(3, 32, 32)
のためこの層の出力のshape
は(96, 32, 32)
となります.valid
の場合の出力のshape
は(96, 32-(3-1), 32-(3-1))=(96, 30, 30)
となります.その他にstride
なども設定することができます.
モデルの学習
from keras.callbacks import EarlyStopping
from keras.callbacks import LearningRateScheduler
from keras.optimizers import Adam
from keras.optimizers import SGD
from animeface import AnimeFaceDataset
class Schedule(object):
def __init__(self, init=0.01):
self.init = init
def __call__(self, epoch):
lr = self.init
for i in xrange(1, epoch+1):
if i%5==0:
lr *= 0.5
return lr
def get_schedule_func(init):
return Schedule(init)
dataset = AnimeFaceDataset()
dataset.load_data_target()
x = dataset.data
y = dataset.target
n_class = len(set(y))
perm = np.random.permutation(len(y))
x = x[perm]
y = y[perm]
model = build_deep_cnn(n_class)
model.summary()
init_learning_rate = 1e-2
opt = SGD(lr=init_learning_rate, decay=0.0, momentum=0.9, nesterov=False)
model.compile(loss='sparse_categorical_crossentropy', optimizer=opt, metrics=["acc"])
early_stopping = EarlyStopping(monitor='val_loss', patience=3, verbose=0, mode='auto')
lrs = LearningRateScheduler(get_schedule_func(init_learning_rate))
hist = model.fit(x, y,
batch_size=128,
nb_epoch=50,
validation_split=0.1,
verbose=1,
callbacks=[early_stopping, lrs])
学習するfit
関数の引数のcallbacks
には,収束判定したら自動的に学習を終了するEarlyStopping
や,epoch
毎に学習率を調整することができるLearningRateScheduler
などを指定することができ,便利です.
簡単にLearningRateScheduler
について説明すると,「引数に現在のepoch
数を(0はじまり)を与えた時に学習率を返してくれる関数」を引数に取ります.例えば,
class Schedule(object):
def __init__(self, init=0.01):
self.init = init
def __call__(self, epoch):
lr = self.init
for i in xrange(1, epoch+1):
if i%5==0:
lr *= 0.5
return lr
def get_schedule_func(init):
return Schedule(init)
lrs = LearningRateScheduler(get_schedule_fun(0.01))
のようにやると,初期学習率0.01で5epoch毎に学習率が半減する,といった感じになります.
また,fit
の返り値のオブジェクトの.history
には辞書型で各epoch毎の誤差関数等を保存してくれます.PandasとMatplotlibを使うと便利に可視化することができます.
import pandas as pd
import matplotlib.pyplot as plt
plt.style.use("ggplot")
df = pd.DataFrame(hist.history)
df.index += 1
df.index.name = "epoch"
df[["acc", "val_acc"]].plot(linewidth=2)
plt.savefig("acc_history.pdf")
df[["loss", "val_loss"]].plot(linewidth=2)
plt.savefig("loss_history.pdf")
実験結果
結果は以下の通りとなり,検証用の正解率は約6割弱となりました.
実は最適化手法にAdamを使うと検証用の正解率は7割を超えるのでやってみてください.
誤差関数の推移
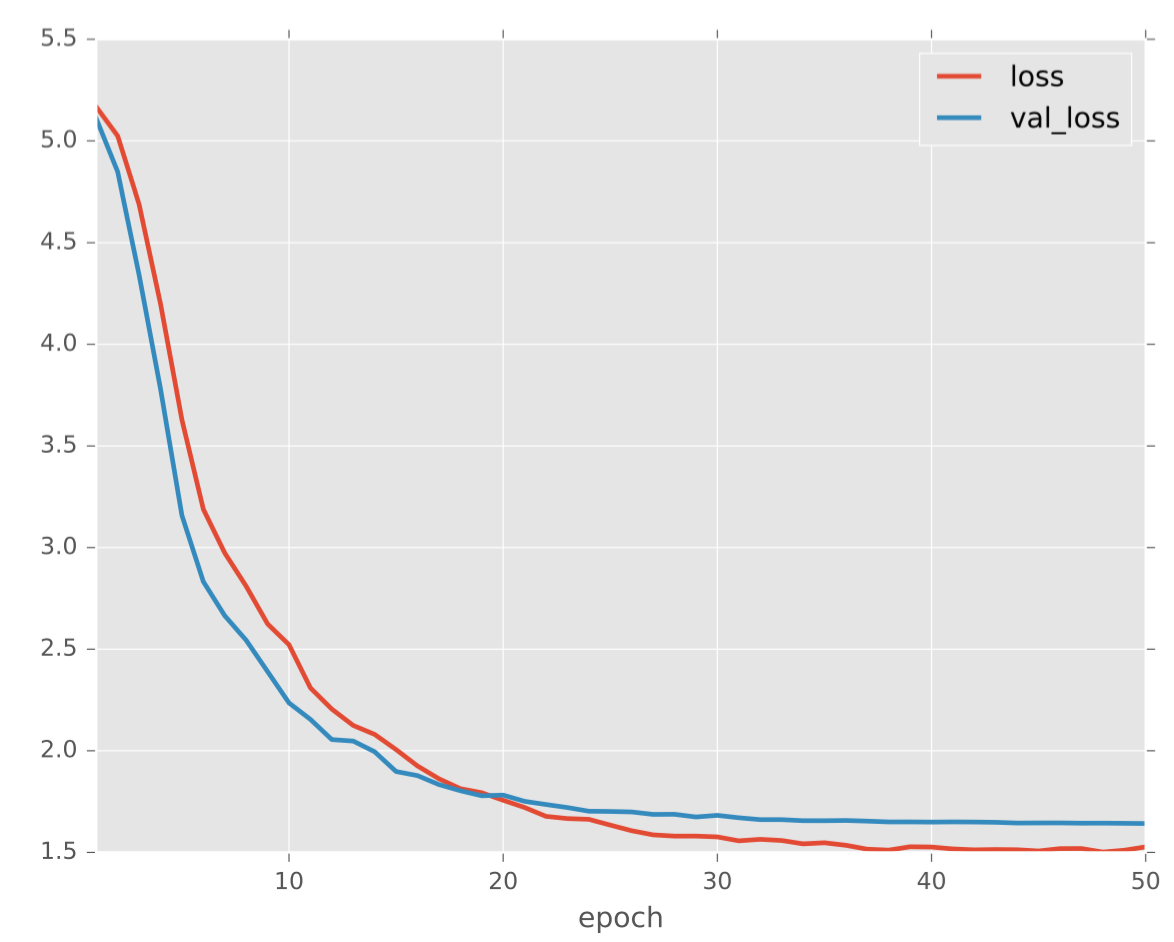
正解率の推移
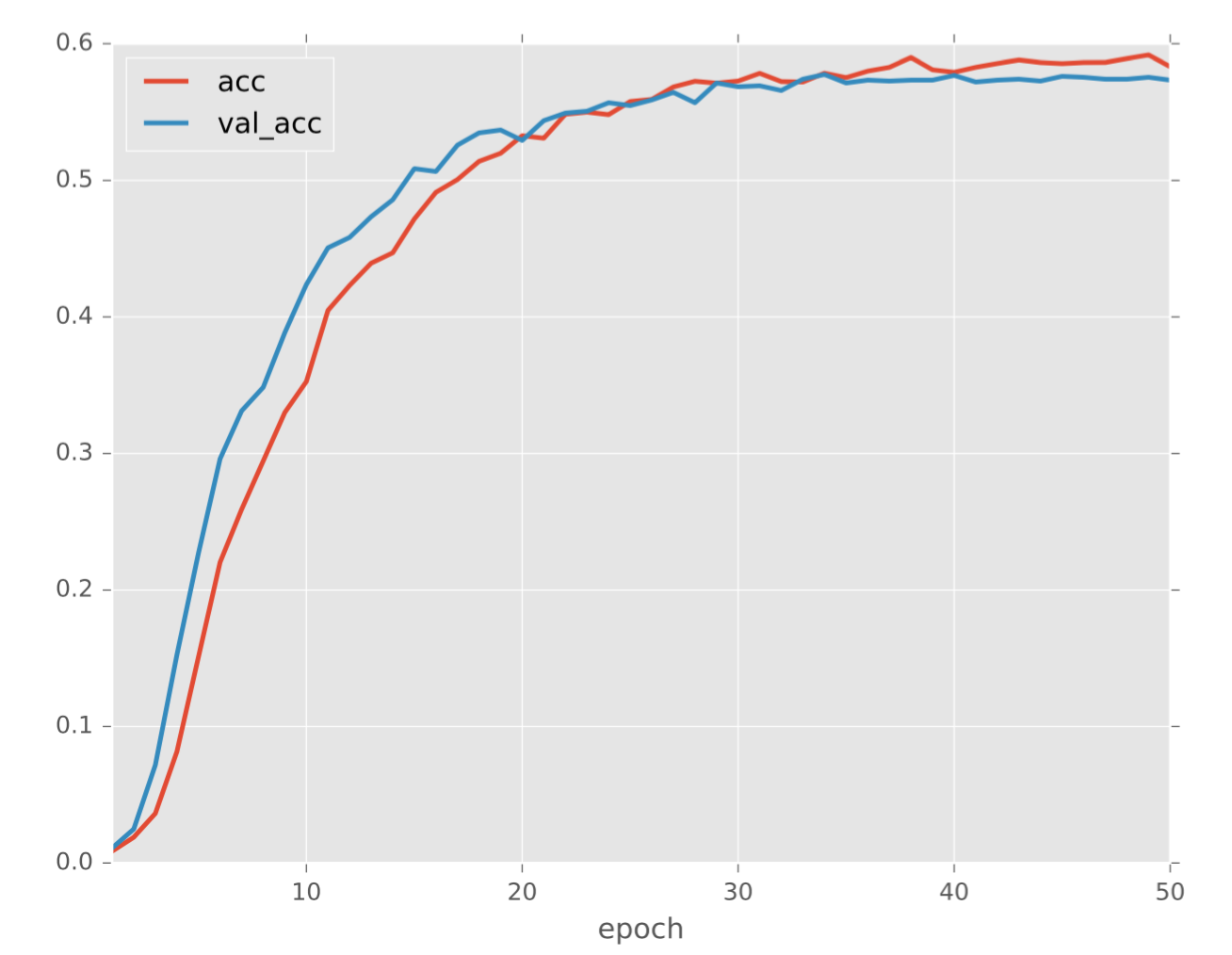