前回の記事で、faiss のインストールと、サービス(faiss_service.py)の作成(2025/4/28追記)を行いましたが、使いやすさのために補足します。すべてを使用しているわけではありません。
OpenAPI (Swagger) 対応
pip install flasgger
# from flask の下
from flasgger import Swagger
# app = ... の下
swagger = Swagger(app)
# """クエリベクトルで検索""" を以下に変更
"""
クエリベクトルで検索
---
tags:
- Document Management
description: クエリをベクトル化してKnowledge Baseから関連ドキュメントを検索します。
parameters:
- in: body
name: body
required: true
schema:
type: object
required:
- knowledge_id
- query
- retrieval_setting
properties:
knowledge_id:
type: string
description: 検索対象のKnowledge BaseのID
example: "AAA-BBB-CCC"
query:
type: string
description: 検索クエリのテキスト
example: "売上レポートについて教えてください。"
retrieval_setting:
type: object
description: 検索の詳細設定
properties:
top_k:
type: integer
description: 取得する上位ドキュメント数
example: 5
score_threshold:
type: number
format: float
description: スコアの最低しきい値(小さいとヒットしやすい)
example: 0.5
responses:
200:
description: 検索結果が返された場合
schema:
type: object
properties:
records:
type: array
items:
type: object
properties:
content:
type: string
description: ドキュメント本文
example: "売上レポート2023年第1四半期版"
title:
type: string
description: ドキュメントタイトル
example: "2023年Q1 売上まとめ.txt"
metadata:
type: object
description: メタデータ(任意)
example: {"category": "sales"}
score:
type: number
format: float
description: 類似スコア(1に近いほど類似)
example: 0.87
500:
description: サーバーエラー
schema:
type: object
properties:
error_code:
type: integer
example: 5000
error_msg:
type: string
example: "Internal Server Error"
"""
# """新しいドキュメント追加""" を以下に変更
"""
新しいドキュメント追加
---
tags:
- Document Management
description: 新しいドキュメントをKnowledge Baseに追加します。
parameters:
- in: body
name: body
required: true
schema:
type: object
required:
- knowledge_id
- content
- title
properties:
knowledge_id:
type: string
description: 登録するKnowledge BaseのID
example: "AAA-BBB-CCC"
content:
type: string
description: 登録するドキュメントの本文
example: "これはサンプルドキュメントの本文です。"
title:
type: string
description: ドキュメントのタイトル
example: "サンプルドキュメント.txt"
metadata:
type: object
description: 任意のメタデータ情報
example: {"category": "example"}
responses:
200:
description: 正常に登録された場合
schema:
type: object
properties:
status:
type: string
example: "success"
id:
type: string
description: 登録されたドキュメントのUUID
example: "d290f1ee-6c54-4b01-90e6-d701748f0851"
500:
description: サーバーエラー(登録に失敗した場合)
schema:
type: object
properties:
error_code:
type: integer
example: 5000
error_msg:
type: string
example: "Internal Server Error"
"""
# """ドキュメントを削除""" を以下に変更
"""
ドキュメントを削除
---
tags:
- Document Management
description: 指定されたドキュメントIDを削除します。
parameters:
- in: body
name: body
required: true
schema:
type: object
required:
- id
properties:
id:
type: string
description: 削除対象のドキュメントID(UUID形式)
example: "d290f1ee-6c54-4b01-90e6-d701748f0851"
responses:
200:
description: 正常に削除された場合
schema:
type: object
properties:
status:
type: string
example: "success"
id:
type: string
description: 削除されたドキュメントのID
500:
description: サーバーエラー(削除に失敗した場合)
schema:
type: object
properties:
error_code:
type: integer
example: 5000
error_msg:
type: string
example: "Internal Server Error"
"""
ウェブページを表示
http://192.168.1.13:8000/apidocs/
/add の「Try it out」ボタンを押下したとき
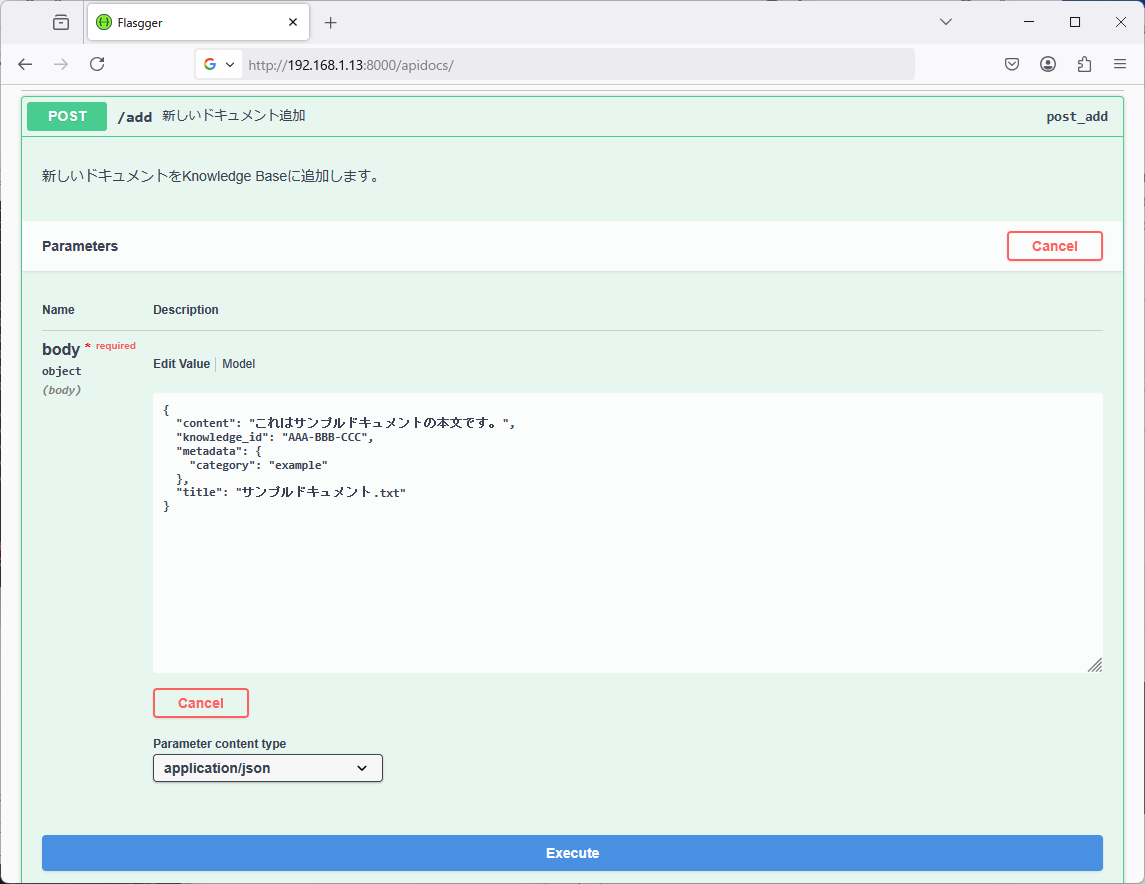
content に改行を入れる際は「¥n」を記入します(JSON の仕様)。テキストエディタで編集するときは、正規表現を利用して「¥r¥n」を「¥¥n」(¥を2つ)に置換します。
「"」は「¥¥"」に置換します。
タブは空白に置換します。正規表現を利用して「¥t」を「 」(半角空白4つ)に置換します。
「¥」(バックスラッシュ)は「¥¥」(¥を2つ)に置換します。
登録データの削除
rm faiss.index
rm doc_id_map.json
rm vector_map.npy # vector_map を使用している場合
psql -U webmaster -d webmaster -h localhost
DELETE FROM knowledge_documents;
登録データのバックアップ
mkdir backup
cp faiss.index ./backup/faiss_$(date '+%Y%m%d_%H%M%S').index
cp doc_id_map.json ./backup/doc_id_map_$(date '+%Y%m%d_%H%M%S').json
cp vector_map.npy ./backup/vector_map_$(date '+%Y%m%d_%H%M%S').npy # vector_map を使用している場合
pg_dump -U webmaster -d webmaster -t knowledge_documents -F p -f ./backup/knowledge_documents_$(date '+%Y%m%d_%H%M%S').sql
(「$」は半角文字)
Dify の構築
Ubuntu に Dify を構築する方法については、次のウェブページに記載しています。以下には、作業の順序と、Ubuntu Server で異なる部分のみ示します。
Docker をインストールします。今回は Ubuntu Server を使用しており、Docker Desktop は使用しないので、インストール作業のうち前半部分のみ行います。
sudo apt-get update
sudo apt-get install ca-certificates curl
sudo install -m 0755 -d /etc/apt/keyrings
sudo curl -fsSL https://download.docker.com/linux/ubuntu/gpg -o /etc/apt/keyrings/docker.asc
sudo chmod a+r /etc/apt/keyrings/docker.asc
echo \
"deb [arch=$(dpkg --print-architecture) signed-by=/etc/apt/keyrings/docker.asc] https://download.docker.com/linux/ubuntu \
$(. /etc/os-release && echo "${UBUNTU_CODENAME:-$VERSION_CODENAME}") stable" | \
sudo tee /etc/apt/sources.list.d/docker.list > /dev/null
sudo apt-get update
sudo apt-get install docker-ce docker-ce-cli containerd.io docker-buildx-plugin docker-compose-plugin
途中で選択画面が表示されますが、デフォルトのまま「OK」で進みます
動作することを確認します
sudo docker run hello-world
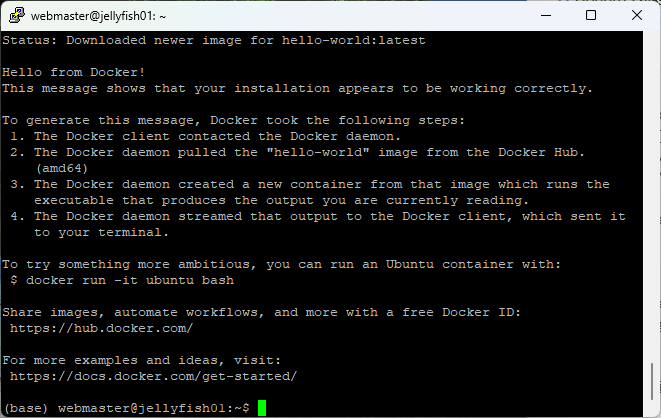
Dify をインストールします。
mkdir Repos
cd Repos
git clone https://github.com/langgenius/dify.git
cd dify/docker
cp .env.example .env
docker compose up -d
delete で OpenAI API の再ベクトル化を行わない方法
以下のように変更します。
# doc_id_map の下
vector_map = {}
# それぞれ追加
# ロード
vectors = np.load("vector_map.npy")
vector_map = {doc_id: vectors[i] for i, doc_id in enumerate(doc_id_map)}
# 新規作成(上記で初期化)
# 保存
vectors = np.array([vector_map[doc_id] for doc_id in doc_id_map], dtype=np.float32)
np.save("vector_map.npy", vectors)
# def add()
# doc_id_map.append(new_id) の下
vector_map[new_id] = vector
@app.route('/delete', methods=['POST'])
def delete():
"""ドキュメントを削除"""
try:
data = request.get_json()
doc_id = data['id'] # 削除対象のUUID文字列
# knowledge が複数ある場合は、複数 faiss_service_n.py を作成して、ポートで分ける
# 関数内で値を変更しする際は、global宣言が必要
global gpu_index
global doc_id_map
global vector_map
# PostgreSQLから削除
cur = db_conn.cursor()
cur.execute("DELETE FROM knowledge_documents WHERE id = %s", (doc_id,))
db_conn.commit()
# doc_id_map から削除対象の index を取得
if doc_id not in doc_id_map or doc_id not in vector_map:
return jsonify({"error_code": 4004, "error_msg": "Document ID not found"}), 404
index_to_remove = doc_id_map.index(doc_id)
# doc_id_map から削除し、vector_map からも削除
doc_id_map.pop(index_to_remove)
del vector_map[doc_id]
# FAISS 再構築
dimension = 1536
index = faiss.IndexFlatL2(dimension)
gpu_index = faiss.index_cpu_to_gpu(res, 0, index)
for kept_id in doc_id_map:
vector = vector_map[kept_id]
gpu_index.add(np.array([vector]))
# 保存
save_faiss()
return jsonify({"status": "success", "id": doc_id})
except Exception as e:
return jsonify({"error_code": 5000, "error_msg": str(e)}), 500
ドキュメント一覧を表示
@app.route('/list', methods=['GET'])
def list():
"""
ドキュメント一覧を表示
---
tags:
- Document Management
summary: 登録されたドキュメントの一覧を表示します
description:
登録されている全ドキュメントを取得し、index、doc_id、knowledge_id、title、文字数、作成日を表示します。
さらに、データベース内レコード数、doc_id_map数、vector_map数の統計も返します。
responses:
200:
description: ドキュメント一覧と統計情報
schema:
type: object
properties:
documents:
type: array
description: ドキュメントのリスト
items:
type: object
properties:
index:
type: integer
description: リスト内の連番
example: 0
doc_id:
type: string
description: ドキュメントの一意ID
example: "abc123"
knowledge_id:
type: string
description: ナレッジベースのID
example: "AAA-BBB-CCC"
title:
type: string
description: ドキュメントのタイトル
example: "Sample Title"
char_number:
type: integer
description: コンテンツの文字数
example: 1342
created_at:
type: string
format: datetime
description: 作成日時
example: "2024-07-10 12:34:56"
summary:
type: object
description: 登録数の統計
properties:
database_records:
type: integer
description: データベースのドキュメント総数
example: 25
doc_id_map_count:
type: integer
description: メモリ上のdoc_id_map件数
example: 25
vector_map_count:
type: integer
description: メモリ上のvector_map件数
example: 25
500:
description: サーバー内部エラー
schema:
type: object
properties:
error_code:
type: integer
example: 5000
error_msg:
type: string
example: "Database connection failed"
"""
global doc_id_map
global vector_map
try:
cur = db_conn.cursor()
cur.execute("SELECT id, knowledge_id, title, content, created_at FROM knowledge_documents ORDER BY created_at ASC")
rows = cur.fetchall()
documents = []
for index, row in enumerate(rows):
doc_id, knowledge_id, title, content, created_at = row
documents.append({
"index": index,
"doc_id": doc_id,
"knowledge_id": knowledge_id,
"title": title,
"char_number": len(content or ""),
"created_at": created_at.strftime("%Y-%m-%d %H:%M:%S")
})
summary = {
"database_records": len(rows),
"doc_id_map_count": len(doc_id_map),
"vector_map_count": len(vector_map)
}
return jsonify({
"documents": documents,
"summary": summary
})
except Exception as e:
return jsonify({"error_code": 5000, "error_msg": str(e)}), 500