データサイエンス・機械学習を行うに当たって、Numpyの基本的な操作と個人的によく使う機能をまとめました。
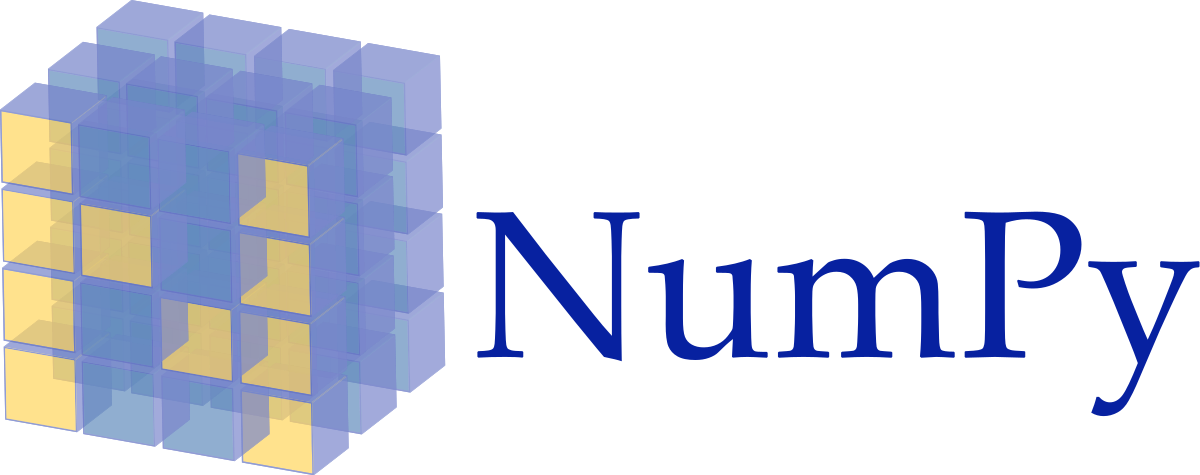
NumpyはPythonの科学技術計算に特化したライブラリで、ベクトルや行列などの表現が可能。
公式ドキュメント: http://www.numpy.org/
Numpy基本機能
###0.ライブラリのインポート
import numpy as np
###1.配列の作成
■ 1次元配列 np.array()
>>> np.array([1, 2, 3])
array([1, 2, 3])
■ 2次元配列 np.array()
>>> np.array([[1, 2, 3], [4, 5, 6]])
array([1, 2, 3],
[4, 5, 6]])
■ print関数で配列をすっきり表示
a = np.array([[1, 2, 3], [4, 5, 6]])
print(a)
[[1 2 3]
[4 5 6]]
■ 全ての要素が0である行列を返す np.zeros()
>>> np.zeros((2, 4)) #引数に配列の形態を入力
array([0, 0, 0, 0],
[0, 0, 0, 0]])
■ 全ての要素が1である行列を返す np.ones()
>>> np.ones((3, 3)) #引数に配列の形態を入力
array([[1., 1., 1.],
[1., 1., 1.],
[1., 1., 1.]])
■ range関数のような連続する1次元の行列を作る(Numpy版 range関数) np.arange()
>>> np.arange(11) #0から1までの連続値
array([0, 1, 2, 3, 4, 5, 6, 7, 8, 9, 10])
>>> np.arange(0, 16, 3) #0から15までの3ずつ大きくなる連続値
array([0, 3, 6, 9, 12, 15])
■ 指定した範囲内で等間隔に分割された値の行列を作る np.linspace()
>>> np.linspace(0, 5, 9) #0から5までの範囲を9つに均等に分割 (9つの分割点を返す)
array([0., 0.625, 1.25, 1.875, 2.5, 3.125, 3.75, 4.375, 5.])
■ 単位行列の生成 np.eye()
>>> np.eye(3, 3)
array([[1., 0., 0.],
[0., 1., 0.],
[0., 0., 1.]])
■ 全て指定の数値の配列の生成 np.full()
>>> np.full((4, 2), 123)
array([[123, 123],
[123, 123],
[123, 123],
[123, 123]])
###2.配列の情報を確認
- .ndim : 行列の次元を表します。最初に何個 "[" が続いているかに対応します。
- .shape : 各次元の要素数を表示。
- .size : 行列の要素の全個数です。
- .dtype : 要素のデータ型を表します。
a = np.array([[1, 2, 3], [4, 5, 6]])
>>> a.shape #各次元の要素数を確認
(2, 3)
###3.配列の参照・変形など
■ インデックス・スライス操作
a = np.array([[1, 2, 3], [4, 5, 6]])
print(a)
[[1 2 3]
[4 5 6]]
>>> a[0, 2] #1行目の3列目の値を表示
3
>>> a[-1, 1] #後ろから1行目(この場合は2列目)の2列目の値を表示
5
print(a[:, 2]) #全行の3列目を表示(:は全てを意味する)
[3 6]
a = np.array([0, 1, 2, 3, 4, 5])
>>> a
[0 1 2 3 4 5]
print(a[:4]) #4つ目まで表示
[0 1 2 3]
print(a[2:5]) #3つ目から5つ目まで表示
[2 3 4]
■ 配列の変形 .reshape()
a = np.array([0, 1, 2, 3, 4, 5])
>>> a
[0 1 2 3 4 5]
>>> a.reshape(3, 2) #3×2に変形
array([[0, 1],
[2, 3],
[4, 5]])
#指定した配列の要素数が合わない場合はエラーになる
■ その他
- .ravel() : 行列を1次元に変換する
- .flatten() : 行列を1次元に変換する
- .T : 行列を転置させる
- .diagonal() : 対角要素を表示
###4.乱数
Python標準のrandomモジュールとは別に、Numpyにも乱数発生機能がある。
■ 0以上1未満の乱数を生成 np.random.random()
>>> np.random.random(10)
array([0.03554718, 0.74723843, 0.27291435, 0.26284813, 0.79078377,
0.00814339, 0.41940143, 0.81117724, 0.98409866, 0.38093226])
■ 乱数の固定 np.random.seed()
np.random.seed(0) #同じ乱数が生成される
■ 0以上1未満の高次元の乱数配列生成 np.random.rand()
>>> np.random.rand(3, 4)
array([[0.85371393, 0.87247222, 0.09849451, 0.84980366],
[0.17332104, 0.84787819, 0.98709454, 0.99205639],
[0.78306371, 0.8567189 , 0.75677948, 0.60070442]])
■ 指定範囲内の乱数を生成 np.random.randint() / np.random.uniform()
>>> np.random.randint(1, 10, (2, 3))
#1次元の場合はタプルでなく数値を入力
#1から10までの整数をランダム生成 <10は出ない>
array([[3, 1, 4],
[8, 9, 2]])
>>> np.random.uniform(1, 10, (2, 3))
#1から10までの小数値をランダム生成
array([[6.04938728, 8.58981204, 2.71022283],
[5.7859627 , 7.2292891 , 9.7463006 ]])
■ 平均0、標準偏差1の標準正規分布に従う乱数の出力 np.random.randn()
>>> np.random.randn(3, 2)
array([[ 0.48735179, 1.70962469],
[-0.1878482 , -0.22249371],
[-0.71046488, -1.26965019]])
■ 任意の平均、標準偏差の正規分布に従う乱数の出力 np.random.normal()
>>> np.random.normal(0, 1, (3, 2)) #平均0、標準偏差1、3行2列 で指定
array([[ 0.44271636, 0.0011947 ],
[-0.11190774, 0.88837843],
[-0.32281972, 0.62059076]])
###5.ユニバーサル関数
Numpyの関数はメソッド呼び出し可能
- .sum() : 合計
- .max() : 最大値
- .min() : 最小値
- .mean() : 平均値
- .var() : 分散
- .std() : 標準偏差
- .abs() : 絶対値
- .floor() : 四捨五入
axisで行<0>と列<1>を指定可能
- .min(axis=0) 最小の行を表示
- .min(axis=1) 最小の列を表示
###6.その他機能
■ 内積の計算 np.dot()
vector_a = np.array([1, 2, 3])
vector_b = np.array([4, 5, 6])
>>> np.dot(vector_a, vector_b)
32
■ 配列に対して自作の関数を適用したい場合 np.vectorize()
np.vectorizeは、関数を配列に適用できるように変換する関数
a = np.array([[1, 2, 3],
[4, 5, 6],
[7, 8, 9]])
# ラムダ式で1000を足す関数を作成
add_1000 = lambda x: x + 1000
# np.vectorize()に関数を読み込む
vectorized_add_1000 = np.vectorize(add_1000)
# 配列aに適用
>>> vectorized_add_1000(a)
array([[1001, 1002, 1003],
[1004, 1005, 1006],
[1007, 1008, 1009]])