はじめに
何の記事?
Fortran Advent Calendar 2023 11日目 で curand
を使って乱数を生成したので, その乱数を使って Fortran
っぽく数値計算の例を見せようという記事.
Isingスピンとは?
2つの状態を持つ離散的なスピン. スピンが一つの場合は何も面白いことが起きないが, 2次元格子上
にスピンを配置して相互作用がある
場合は相転移をする.
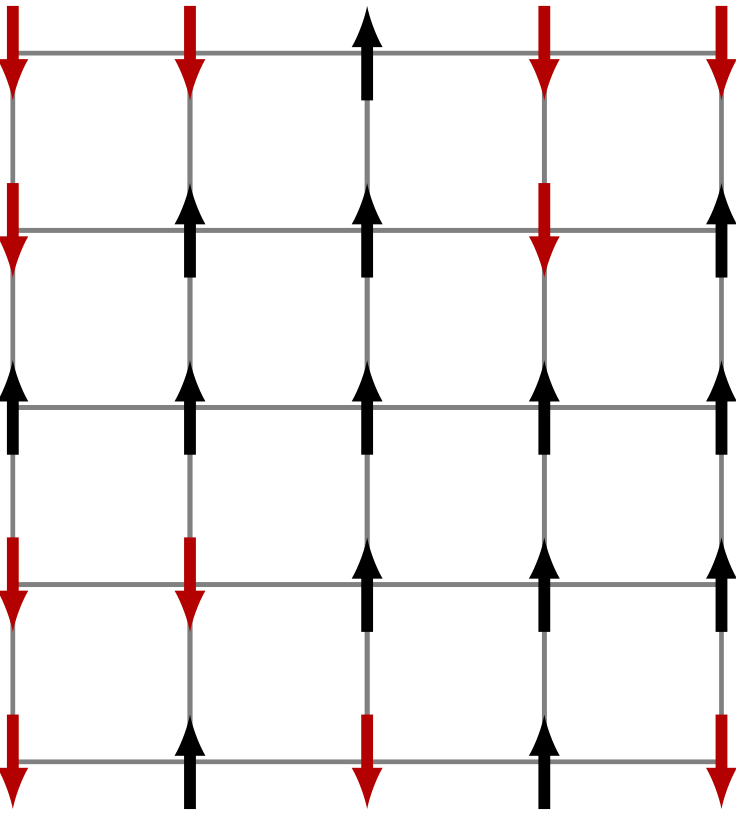
(上の図: 黒と赤の2状態のスピンがあり, 2次元格子状に配置された2次元Ising模型. 相互作用は一番近い4つのスピンとのボンドにある.)
詳しいことは省略. この模型をMonte Carlo
シミュレーションでシミュレートする.
確率でスピンを反転させる!(Monte Carlo シミュレーション)
- 2次元Ising模型は全部の状態を生成すれば物理的が分かる.
- が, $2^{(スピン数)}$個の状態があるため, 全部は無理.
- なので, 模型のエネルギーがBoltzmann分布 $\exp(-\beta E)$ の分布に従うようにスピンを反転させる.
($\beta = 1/T$, Boltzmann定数 $k_{B} = 1$ とする.)
- スピン反転のときはエネルギーが $\Delta E$ 変わるので, $\exp(-\beta \Delta E)$ の確率でスピンを反転させると模型がBoltzmann分布に従う.
- 全部の格子に対して順番に $\Delta E$を求めて更新するかしないかを決めるだけ.
- doループとif文だけの簡単なプログラム!
このシミュレーションはGPU計算向き!
- あるスピンを更新することを考えると, そのスピンの更新は最近接の4つのスピンにのみ依存する.
- よって, チェス盤の白マスのスピンを更新→黒マスのスピンを更新, のように交互に更新することで 全体の半分を並列に計算することが可能 である.
GPU計算してみた.
ソースコードはマイGithubに(https://github.com/osada-yum/CUDA_Fortran_MC_simulation_spin).
実行環境
- Ubuntu 20.04.6 LTS
- CUDA 12.2
- NVIDIA GeForce RTX4090
$ cat fpm_run.sh
FCFLAGS="-O2 -acc -cuda -cudalib=curand"
fpm --verbose --compiler='nvfortran' --flag="${FCFLAGS}" run
サイズ$N$ | Monte Carlo Step $t$ | サンプル数$N_{s}$ | スピンの更新回数$M$ | シミュレーションの掛かった時間 |
---|---|---|---|---|
$1001\times1000$ | $1000$ | $100$ | $10^{11}$ くらい | 12秒くらい |
$1501\times1500$ | $1000$ | $100$ | $2.3\times10^{11}$ くらい | 15秒くらい |
$2001\times2000$ | $1000$ | $100$ | $4\times10^{11}$ くらい | 20秒くらい |
$3001\times3000$ | $1000$ | $100$ | $9\times10^{11}$ くらい | 60秒くらい |
$4001\times4000$ | $1000$ | $100$ | $1.6\times10^{12}$ くらい | 120秒くらい |
$5001\times5000$ | $1000$ | $100$ | $2.5\times10^{12}$ くらい | 190秒くらい |
$10001\times10000$ | $1000$ | $100$ | $\times10^{13}$ くらい | 750秒くらい |
- $N = 2001\times2000$ くらいでは計算時間がO$(M)$でないため, GPUが計算を持て余している?
- $N = 5001\times5000$ から $N = 10001\times10000$ では計算時間が$O(M)$のため, GPUをフルに使えている?
結論
- めちゃくちゃ速い.
副産物 (nvfortran
でできたこと)
type-bound-procedure
が使えた
-
device
側(GPU側)で確保する変数も定義することができた.
ising2d_gpu_m.f90
type :: ising2d_gpu
private
real(real64) :: beta_
integer(int64) :: nx_, ny_, nall_
integer(int64) :: norishiro_begin_, norishiro_end_
integer(int32), allocatable, device :: spins_(:)
real(real64), allocatable, device :: exparr_(:)
real(real64), allocatable, device :: randoms_(:)
type(curandGenerator) :: rand_gen_
contains
procedure, pass :: init => init_ising2d_gpu
! 省略
end type ising2d_gpu
type-bound-procedure から attributes(global)
や attributes(device)
なやつらを呼び出せる.
シェブロン <<<*, *>>>
が付いているやつを呼び出せる.
ising2d_gpu_m.f90
impure subroutine update_ising2d_gpu(this)
class(ising2d_gpu), intent(inout) :: this
integer(int64) :: lb, ub
lb = lbound(this%spins_, dim = 1, kind = int64)
ub = ubound(this%spins_, dim = 1, kind = int64)
ising2d_gpu_stat = curandGenerate(this%rand_gen_, this%randoms_(1:this%nall_), this%nall_)
call update_sub <<<(this%nall_ + NUM_THREADS - 1) / NUM_THREADS, NUM_THREADS>>> &
& (lb, ub, this%nx_, this%nall_, this%spins_, this%randoms_(1:this%nall_), this%exparr_, 1)
call this%update_norishiro()
call update_sub <<<(this%nall_ + NUM_THREADS - 1) / NUM_THREADS, NUM_THREADS>>> &
& (lb, ub, this%nx_, this%nall_, this%spins_, this%randoms_(1:this%nall_), this%exparr_, 2)
call this%update_norishiro()
end subroutine update_ising2d_gpu
OpenACCプラグマもOK
!$acc parallel loop reduction(+:res)
がちゃんと仕事する.
ising2d_gpu_m.f90
pure integer(int64) function calc_energy_sum_ising2d_gpu(this) result(res)
class(ising2d_gpu), intent(in) :: this
integer(int64) :: i
res = 0_int64
!$acc parallel loop reduction(+:res)
do i = 1, this%nall_
res = res - int(this%spins_(i) * (this%spins_(i + 1) + this%spins_(i + this%nx_)), int64)
end do
end function calc_energy_sum_ising2d_gpu