M5StickV/Maix1 シリーズの深層学習モデルのkmodelの作リ方について説明していきます。学習データkmodelのトレーニングは、Tensorflowをフレームワークとして使用します。sipeedからGitHubで提供されているmaix_trainのコードを使います。
maix_trainを使うと、2種類の機械学習のモデルを作成することができます。
・クラス分類(Mobilenet V1を使用): 写真に写っている物体が何であるかを識別します。
・オブジェクト認識(YOLO V2を使用): 写真に写っている物体を検出し、同時に座標とサイズを求めます。
PC環境
構築手順に入る前に、まずPCに以下のバージョンのUbuntuをインストールした環境で、構築しました。
Ubuntu 22.04.3 LTS(x64)
Minicondaのインストール
まず最初に、Minicondaをインストールして、Pythonの環境を作ります。
Minicondaのインストーラは、Minicondaのウェブサイトからダウンロードして取得します。
% wget https://repo.anaconda.com/miniconda/Miniconda3-latest-Linux-x86_64.sh
% sh Miniconda3-latest-Linux-x86_64.sh
Miniconda上で、pythonの仮想環境を作成します。
% conda create -n maix python=3.7
condaをアクティブ化します。condaのアクティブ化する作業は、シェル立ち上げるごとに必要です。condaは、隔離したPython環境を作成してくれるので、システムのPython環境を誤って変更することがなく、安心してプログラミングができます。
% conda activate maix
maix_trainのダウンロード
sipeedからGitHubで提供されているmaix_trainのコードをダウンロードします。
% git clone https://github.com/sipeed/maix_train --recursive
% cd maix_train/
必要なライブラリをpipでインストールします。
% pip install -r requirements.txt
% pip install pynvml matplotlib requests
一度、初期化を行います。
python3 train.py init
学習データ変換ツールnncaseをインストールする
nncaseは、KerasやTensorFlowで作成した学習データを、KPUの学習データkmodelへ変換します。Kendryteがリリースしており、GitHubからダウンロードできます。
nncaseは、CNNのMobileNetV1/V2やYOLOV1/YOLOV3をサポートしています。
kmodel作成には、nccaseはv0.1.0-rc5のバージョンを使います。
% cd maix_train/
% mkdir -p tools/ncc/ncc_v0.1/
% cd tools/ncc/ncc_v0.1/
% tar Jxf ncc-linux-x86_64.tar.xz
% wget https://github.com/kendryte/nncase/releases/download/v0.1.0-rc5/ncc-linux-x86_64.tar.xz
% tar Jxf ncc-linux-x86_64.tar.xz
nncaseのバージョンを確認します。
% ./ncc --version
ncc 1.0.0
nncaseは、コマンドの引数でオプションを設定します。
-i,--input-format 必須. 入力ファイルのフォーマットを指定します
-o,--output-format 必須. 出力ファイルのフォーマットを指定します
--dataset 必須. データセット(画像データ)のパスを指定します
--dataset-format (Default: image) データセットのフォーマットです
--help ヘルプを表示します
--version Versionを表示します
input (pos. 0) オプション指定ない引数の0個目で、入力ファイルのpathを指定します
output (pos. 1) オプション指定ない引数の1個目で、出力ファイルのpathを指定します
クラス分類(Mobilenet V1)の学習モデル作成
データセットを準備します。画像サイズは224x224で、フォーマットはmaix_train/datasetsのデータセット例を参照してください。
python3 train.py -t classifier -z datasets/test_classifier_datasets.zip train
outフォルダにzipファイルが生成されます。
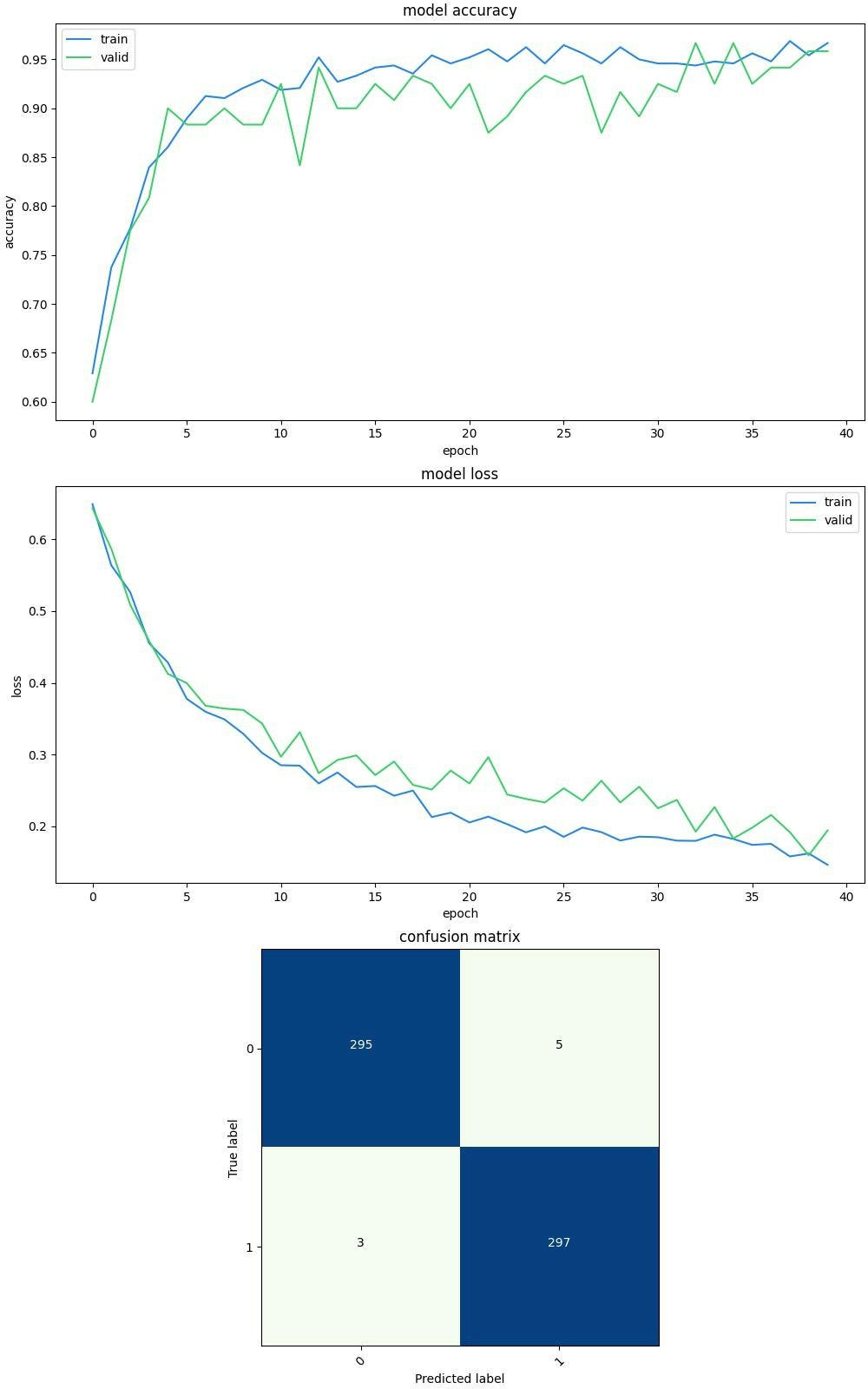
エラーが出た場合の対処
[Errno 2] No such file or directory: 'maix_train/out/datasets/images/1/xxx.jpg'
エラーが出る場合、出力フォルダに画像をコピーします。
cp ./datasets/
nzip test_classifier_datasets.zip
cp -r datasets/images/ ./out/datasets/
オブジェクト認識(YOLO V2を使用)の学習モデル作成
python3 train.py -t detector -z datasets/test_detector_xml_format.zip train
MaixhubやV-Trainで学習したモデルと同様に、outフォルダに結果を含むzipファイルが生成されます。ファイルをSDカードのルートディレクトリにコピーし、M5StickVの電源を入れて実行します。
参考資料
Sipeed Wiki:Local model training
https://wiki.sipeed.com/soft/maixpy/en/course/ai/train/local.html