背景
Semantic SegmentationでDeepLabというのが有名だったから調べてみる事にした。 特徴は 1. Dilated Convolutionを使ってること(画像全体の特徴を取るため) 2. Fully Connected CRFを使っていること(画像全体でrefinementするため)全体像
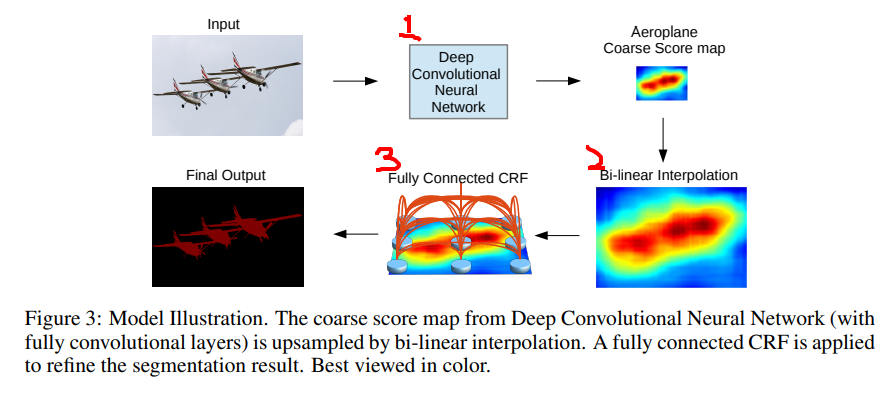DeepLabでは3stepだけ
- CNN(Dilated Conv含む)で畳こむ
- Bi-linear Interpolationで画像サイズを拡大する
- CRFでRefineする
CRFってなんじゃい?!
一度クラスに分類された結果をよりよく修正する事が出来るのがCRF。「同じような色や近くにあるpixelは同じクラスに分類されるはずだ!」
という仮定から
近隣pixelとの類似度(事前に自分で定義した関数)を元にして結果をupdateする
いうなればsmoothingですね
お隣さんとの比較で先端が良くなっている!
詳しくはこちらを
https://news.mynavi.jp/article/cv_future-36/
Fully Connected CRFってなんじゃい?!
従来のCRFは近場だけで比べるからlocalな結果のupdateは出来るんだけど、細い物体とかの精度を上げるのが難しい。(だから飛行機を例に出したのね)
「もっと全体をみなきゃダメだ!」という事でCRFをFully Connectedにしてみたらしい。
計算量ヤバそう笑
左側はCNNによって推定されたclass probability scoreの逆数=>コスト
右側はpixel iとpixel jが違うクラスと分類された時の色情報と距離情報が近ければ値が大きくなる=>コスト
このコストを最小化する事でsmoothing出来る
結論
CRFを使うとsmoothing出来るんだなってのがわかった。
コストの最小の仕方に関して少し分からなかったので、また調べたいと思う
参考文献
SEMANTIC IMAGE SEGMENTATION WITH DEEP CONVOLUTIONAL NETS AND FULLY CONNECTED CRFS https://arxiv.org/pdf/1412.7062.pdfhttps://news.mynavi.jp/article/cv_future-36/
Conditional Random Fields as Recurrent Neural Networks
https://www.cv-foundation.org/openaccess/content_iccv_2015/papers/Zheng_Conditional_Random_Fields_ICCV_2015_paper.pdf