Real-time self-adaptive deep stereo
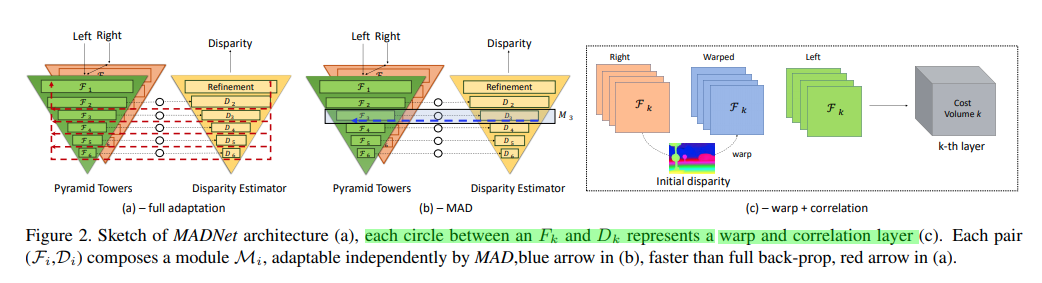 この論文のメインのオンラインアダプションは無視して、ネットワークの構造だけを見ていきたいと思う。新規性
Feature Extraction
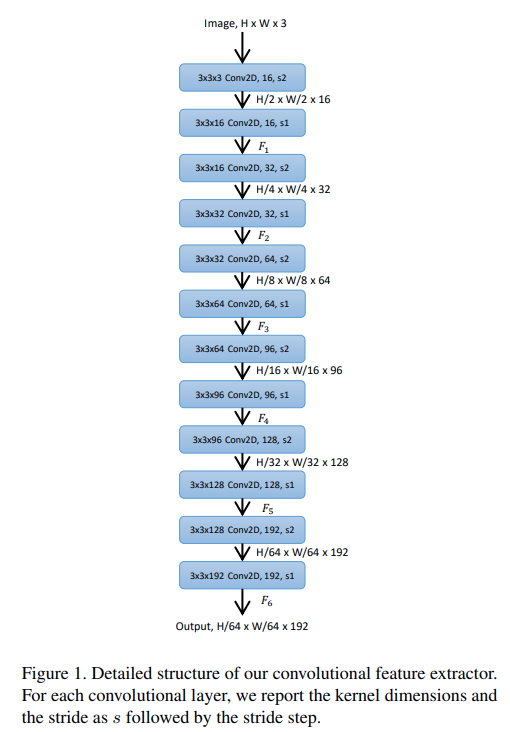 右と左の特徴量をそれぞれ同じネットワークで抽出する。Disparity Estimation at resolution n
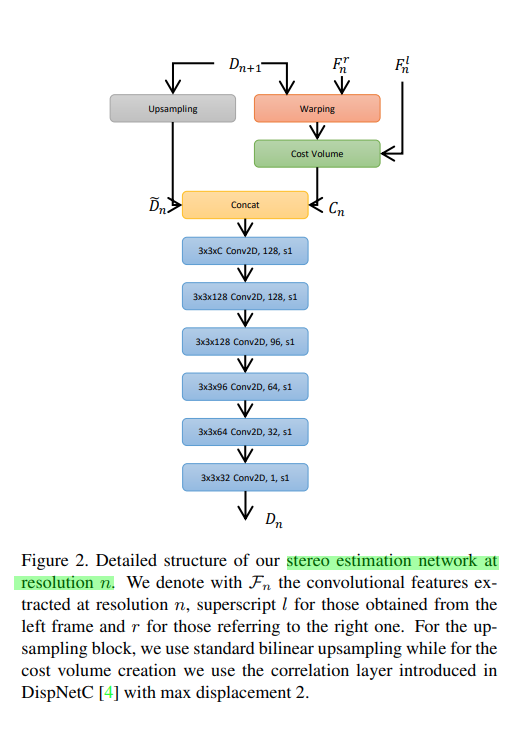 一つ前のDisparityに対して右の特徴量をwarpする事でCost Volumeを作り、Concat(結合)する事で新しいDisparityを推定する。ただし一番低解像度のDisparityを推定する場合は前のDisparityがないので、Correlation Layer(内積でSimilarityを計算する方法)によって最初のDisparityを推定する