2stage detectorと1stage detector
R-CNN, Fast-RCNN, Faster-RCNNが遅かったのはRegion Proposalを用いた2stageのネットワークをだったから。 Region Proposalは多くの物体を提案してしまい処理速度が遅くなる。 それを解決すべく登場したのがYoloでも使われているAnchor Box。Anchor Boxとは?
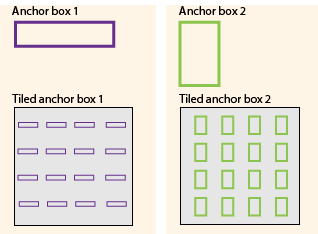様々なサイズや縦横比の長方形のBoxをAnchor Boxという。
Anchor Boxを用いた物体検出
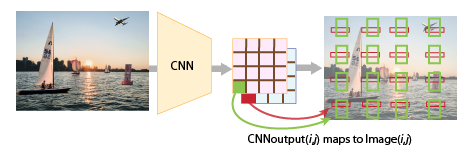CNNによって画像を畳み込み、それぞれのAnchor Boxのサイズのオフセット、位置のオフセット等を学習する事で物体の位置を推測する。
上の画像を例にしてみる。まず2種類のAnchor Boxを16エリアに分けて定義する。右上の飛行機がいるエリアの緑色のAnchor Boxは得られたFeature Mapにより左にOffsetをするように学習してくれれば、飛行機の位置が検出出来る。サイズに関しても同じくオフセットを学習することで推測する事が出来る。
R-CNN系は物体の位置のRegion Proposalによって推定されたBounding Boxのオフセットを計算していたのに対してAnchorBoxでは定義されたAnchorBoxからのoffsetを計算するだけで良いので、計算コストを大幅に削減出来る。