はじめに
今更感があるけど、SRGANをまとめてみた。
[1] C. Ledig, et. al. "Photo-Realistic Single Image Super-Resolution Using a Generative Adversarial Network"
コードも既にいろいろ転がってる。例えばtensorflowの実装。
https://github.com/tensorlayer/srgan
概要
- SRGANという名の超解像度のモデル
- residual blockをベースとしたGANで学習した
- perceptual lossを導入した
以下の図の右から2つ目がSRGANで生成された画像。一番右が元の高解像度画像。元の画像とは違うものの、それっぽい画像が生成されている。
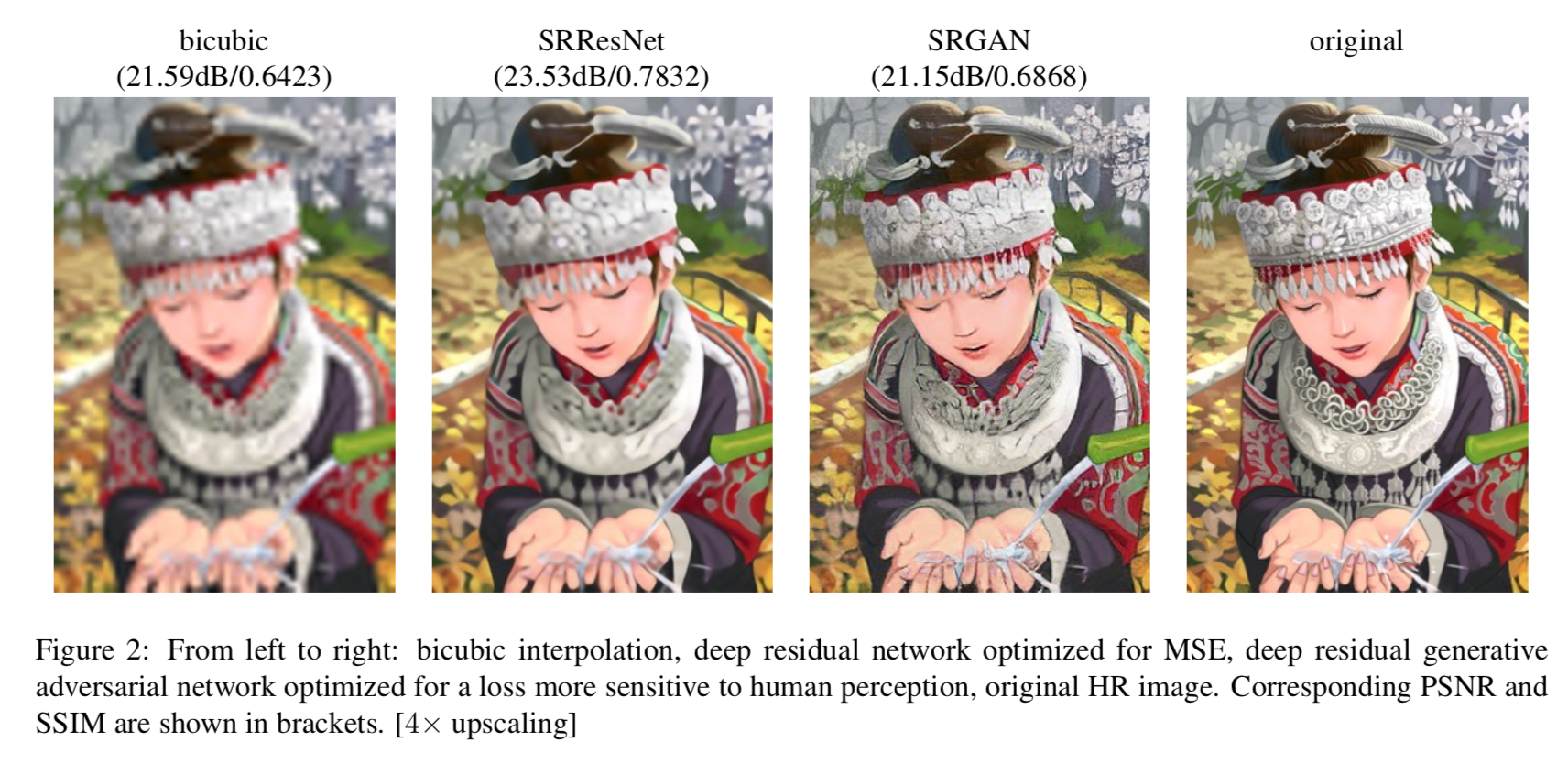
目的関数
Perceptual loss
Perceptual loss はgeneratorを学習させる際のloss。まず loss 全体 $l^{SR}$ は
l^{SR} = l_X^{SR} + 10^{-3} l_{Gen}^{SR}
右辺1項目が content loss 、2項目が adversarial loss。
content loss
この論文の最大の特徴はこの content loss。
これは学習済みのVGGNetに目標値となる高解像度画像 $I^{HR}$ 、及びgeneratorで生成された超解像度画像 $I^{LR}$ を入れ、j 番目のpoolingの前のi番目convolutionの後の特徴量 $\phi_{i,j}$ 同士でMSEを計算する。
l^{SR}_{VGG/i,j} = \frac{1}{W_{i,j}H_{i,j}}\sum^{W_{i,j}}_{x=1}\sum^{H_{i,j}}_{y=1}(\phi_{i,j}(I^{HR})_{x,y} - \phi_{i,j}(G_{\theta_G}( I^{LR}))_{x,y})^2
単に $I^{HR}$ 、と $I^{LR}$ とからMSEをとると、平均的に両者が近い、ぼやけた画像となるが、特徴量同士で比較することでより鮮明で多様な画像を生み出すことが期待される。
以下の図の中央青枠が直接pixel wiseなMSEをとった場合。考えられうる多様な画像(赤枠)の平均的な画像が生成される。perceptual loss(黄色枠)ではより自然な画像に近いものが生成される。

adversarial loss
adversarial な loss は通常のもの。
l_X^{SR} = \sum^{N}_{n=1} - \log D_{\theta_D} (G \theta_G (I^{LR}))
ネットワークのアーキテクチャ
generatorとdiscriminatorのアーキテクチャは以下のresidual blockをベースとしたもの。

実験と結果
使用したデータセット
- Set5
- Set14
- BSD100
- BSD300(テスト用)
metrics
- PSNR(dB)
- SSIM (structural similarity)[2]
- MOS(Mean opinion score)
MOSは評価者に超解像された画像の質をスコアリングする手法。
MSEで学習するとPSNRとSSIMは上がるが、MOSは低いと思われる。一方でcontent loss(あるいはperceptual loss)で学習するとPSNRやSSIMは期待できないがMOSがよくなると期待される。
SRResNetとの比較
SRResNetとの比較は以下。
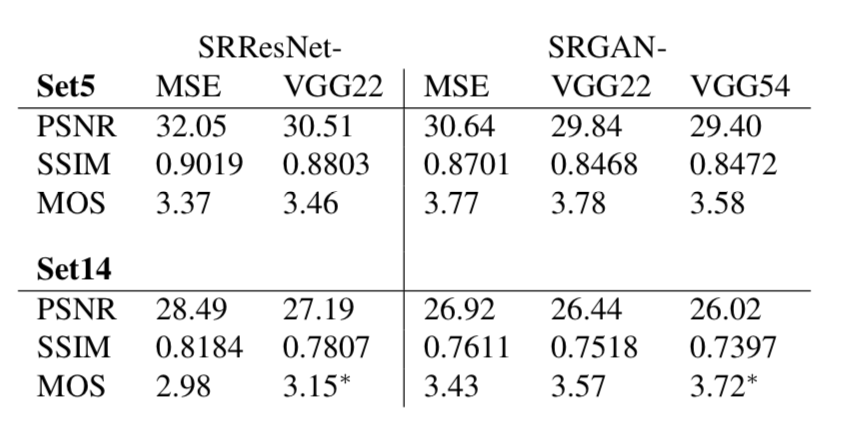
確かにSRRNetと比較した場合、SRGANはPSNRとSSIMは劣るが、MOSが高くなっている。
種々の手法とMOSで比較
SRResNet以外にSRCNNなど多くの手法とMOSを比較した結果は以下。

SRGANが一番いい。
種々の手法とPSNR、SSIM、MOSで比較
種々の手法と3つのメトリクスで比較した結果は以下。

reference
[2] Image Quality Assessment: From Error Visibility to Structural Similarity