この記事は、富士通システムズウェブテクノロジー Advent Calendarの12日目の記事です。
(お約束)本記事の掲載内容は私自身の見解であり、所属する組織を代表するものではありません
はじめに
本記事ではGoogleの画像認識API「Cloud Vision API」を利用する最低限の手順をまとめています。
最後に食べ物の写真の判定を試しています。
余談
個人的な趣味がメシテロ1でして、「おなかすいたなー」と思わせることを目的にLINEのタイムラインに食べ物の写真を投稿しております。
よりおいしそうな写真を撮り、メシテロのクオリティをあげるために画像認識APIで何かできないかと思い、今回はGoogle Cloud Vision APIを試すところから始めています。
今回使ったもの
- Google Cloud Platform
- Cloud Vision APIを利用するにあたり必要なサービス
- Anaconda
- Python本体とよく使われるライブラリを含んだパッケージ
API利用の事前準備
Google Cloud Platformの利用登録
まずはCloud Vision APIを使うためにGoogle Cloud Platformの利用登録を行います。
以下のサイトで「無料で始める」をクリックすると登録手続きが開始されます。
Googleのアカウントで利用可能です。
クラウド コンピューティング サービス | Google Cloud
※無料で利用する場合にもクレジットカードの登録が必要となります。
プロジェクトの作成
登録が完了したらこのような画面が表示され、デフォルトのプロジェクトが作成されています。
赤枠の部分から新規プロジェクトを作成できます。
デフォルトのプロジェクトでもAPIを利用可能ですが、ここでは「Meshitero」プロジェクトを作成しました。
Cloud Vision APIの有効化
検索フォームに「Visoin API」と入力するとCloud Vision APIがヒットするのでクリックし
遷移後の画面で「有効にする」を選択します。
余談:ちなみに「このAPIを試す」を選択すると画面上でデモが試せます。
有効化するとこのような画面が表示されます。
APIキーの発行
今回はPythonで呼び出すので、認証情報を作成します。
Cloud Vision APIの画面で「認証情報を作成」をクリックすると「APIとサービス」の認証情報のページに遷移します。
「認証情報の作成」のプルダウン内から「APIキー」を選択すると、APIキーが発行されます。
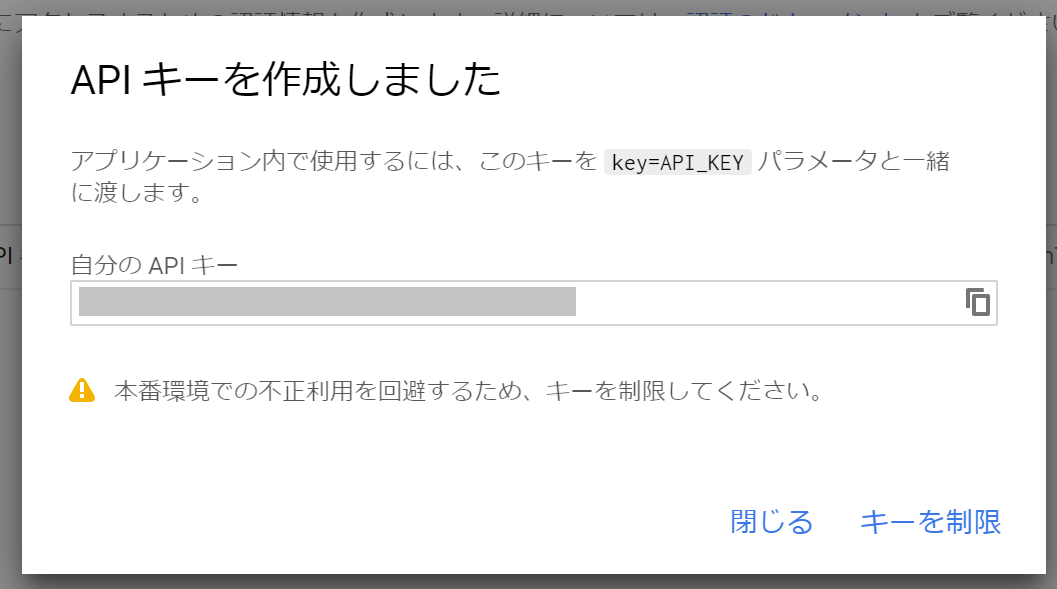
不正利用回避のためキーに制限をかけます。
上記画面で「キーを制限」をクリックするとキーの制限ができる画面に移動するので、
必要に応じた制限をかけましょう。
今回は、IPアドレスで利用の制限をかけ、また利用できるAPIもいったんCloud Vision APIに制限しました。
APIを利用するための設定は以上です。
APIの呼び出し
Anacondaのインストール
APIをPythonで呼び出すため、
以下を参考にAnacondaをインストールしました。
ソースの作成
APIのReferenceの内容を参考にソースを作成します。
APIに渡すリクエストデータの作成
画像のデータはbase64でエンコードされた文字列として渡す必要があるようです。
また、画像分析の種類と、返却する結果の最大数を「features」に指定します。
今回はラベル検出(LABEL_DETECTION)を指定します。
※画像分析の種類は他には顔検出やロゴ・ランドマーク検出があります
APIに渡す際にはJson形式にするため、Json形式へのエンコードも行います。
img_request = []
with open(filename, 'rb') as f:
ctxt = b64encode(f.read()).decode()
img_requests.append({
'image': {'content': ctxt},
'features': [{
'type': 'LABEL_DETECTION',
'maxResults': 10
}]
})
request_data = json.dumps({"requests": img_request }).encode()
APIの呼び出し部分
リクエストデータと、前段で出力したAPIキーを指定し、APIリクエストを送信します。
API_URL = 'https://vision.googleapis.com/v1/images:annotate'
response = requests.post(API_URL,
data=request_data ,
params={'key': api_key},
headers={'Content-Type': 'application/json'})
結果の出力
APIから帰ってきたデータを出力します。
for resp in enumerate(response.json()['responses']):
print (json.dumps(resp, indent=2))
実行
作成したソースにGCPで発行したAPIキーと画像のパスを渡し実行します。
食べ物の画像を何枚か試します。
$ Python Meshitero.py [APIキー] [画像パス]
ソース全体(Meshitero.py)
from base64 import b64encode
from sys import argv
import json
import requests
API_URL = 'https://vision.googleapis.com/v1/images:annotate'
if __name__ == '__main__':
api_key = argv[1]
filename = argv[2]
img_request = []
with open(filename, 'rb') as f:
ctxt = b64encode(f.read()).decode()
img_request.append({
'image': {'content': ctxt},
'features': [{
'type': 'LABEL_DETECTION',
'maxResults': 10
}]
})
request_data = json.dumps({"requests": img_request }).encode()
response = requests.post(API_URL,
data=request_data,
params={'key': api_key},
headers={'Content-Type': 'application/json'})
if response.status_code != 200 or response.json().get('error'):
print(response.text)
else:
for resp in enumerate(response.json()['responses']):
print (json.dumps(resp, indent=2))

実行結果(牡蠣)
{
"labelAnnotations": [
{
"mid": "/m/0_cp5",
"description": "Oyster",
"score": 0.9910632,
"topicality": 0.9910632
},
{
"mid": "/m/02wbm",
"description": "Food",
"score": 0.9903261,
"topicality": 0.9903261
},
{
"mid": "/m/06nwz",
"description": "Seafood",
"score": 0.9609892,
"topicality": 0.9609892
},
{
"mid": "/m/01cqy9",
"description": "Bivalve",
"score": 0.9138548,
"topicality": 0.9138548
},
{
"mid": "/m/02q08p0",
"description": "Dish",
"score": 0.8472096,
"topicality": 0.8472096
},
{
"mid": "/m/01ykh",
"description": "Cuisine",
"score": 0.811229,
"topicality": 0.811229
},
{
"mid": "/m/07xgrh",
"description": "Ingredient",
"score": 0.8011539,
"topicality": 0.8011539
},
{
"mid": "/m/088kg2",
"description": "Oysters rockefeller",
"score": 0.70525026,
"topicality": 0.70525026
},
{
"mid": "/m/0fbdv",
"description": "Shellfish",
"score": 0.6510715,
"topicality": 0.6510715
},
{
"mid": "/m/0ffhy",
"description": "Clam",
"score": 0.6364975,
"topicality": 0.6364975
}
]
}
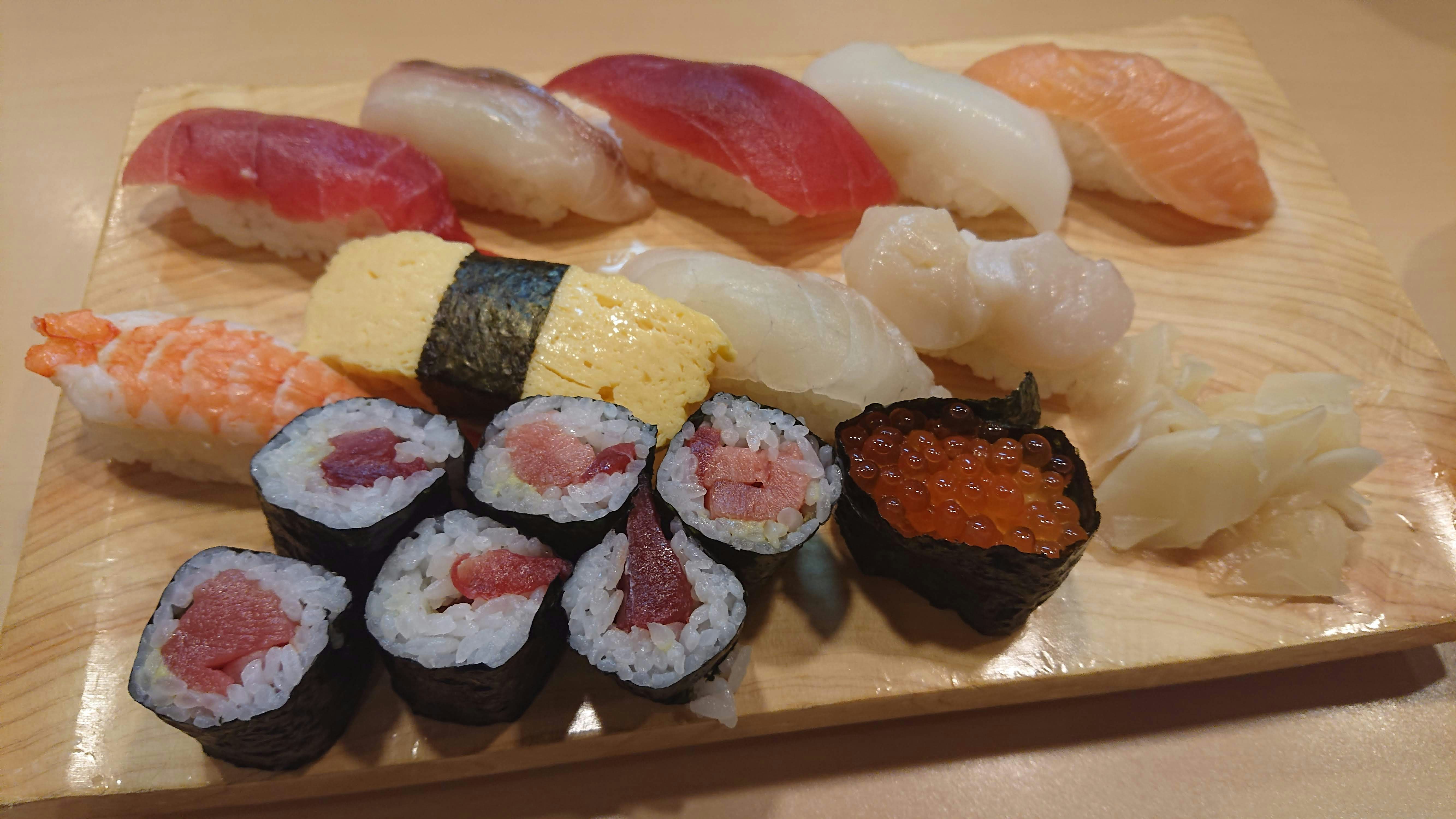
実行結果(寿司)
{
"labelAnnotations": [
{
"mid": "/m/02q08p0",
"description": "Dish",
"score": 0.9934035,
"topicality": 0.9934035
},
{
"mid": "/m/01ykh",
"description": "Cuisine",
"score": 0.9864208,
"topicality": 0.9864208
},
{
"mid": "/m/02wbm",
"description": "Food",
"score": 0.97343695,
"topicality": 0.97343695
},
{
"mid": "/m/048wsd",
"description": "Gimbap",
"score": 0.96859926,
"topicality": 0.96859926
},
{
"mid": "/m/07030",
"description": "Sushi",
"score": 0.9650486,
"topicality": 0.9650486
},
{
"mid": "/m/0cjyd",
"description": "Sashimi",
"score": 0.9185767,
"topicality": 0.9185767
},
{
"mid": "/m/04q6ng",
"description": "Comfort food",
"score": 0.8544887,
"topicality": 0.8544887
},
{
"mid": "/m/07xgrh",
"description": "Ingredient",
"score": 0.8450334,
"topicality": 0.8450334
},
{
"mid": "/m/05jrv",
"description": "Nori",
"score": 0.8431285,
"topicality": 0.8431285
},
{
"mid": "/m/027lnr6",
"description": "Sakana",
"score": 0.8388547,
"topicality": 0.8388547
}
]
}

実行結果(ハンバーガー)
{
"labelAnnotations": [
{
"mid": "/m/02q08p0",
"description": "Dish",
"score": 0.9934035,
"topicality": 0.9934035
},
{
"mid": "/m/02wbm",
"description": "Food",
"score": 0.9903261,
"topicality": 0.9903261
},
{
"mid": "/m/01ykh",
"description": "Cuisine",
"score": 0.9864208,
"topicality": 0.9864208
},
{
"mid": "/m/0h55b",
"description": "Junk food",
"score": 0.9851551,
"topicality": 0.9851551
},
{
"mid": "/m/01_bhs",
"description": "Fast food",
"score": 0.97022384,
"topicality": 0.97022384
},
{
"mid": "/m/0cdn1",
"description": "Hamburger",
"score": 0.9571771,
"topicality": 0.9571771
},
{
"mid": "/m/0cc7bks",
"description": "Buffalo burger",
"score": 0.94575346,
"topicality": 0.94575346
},
{
"mid": "/m/03f476",
"description": "Veggie burger",
"score": 0.9283731,
"topicality": 0.9283731
},
{
"mid": "/m/0bp3f6m",
"description": "Fried food",
"score": 0.9257971,
"topicality": 0.9257971
},
{
"mid": "/m/02y6n",
"description": "French fries",
"score": 0.92217153,
"topicality": 0.92217153
}
]
}
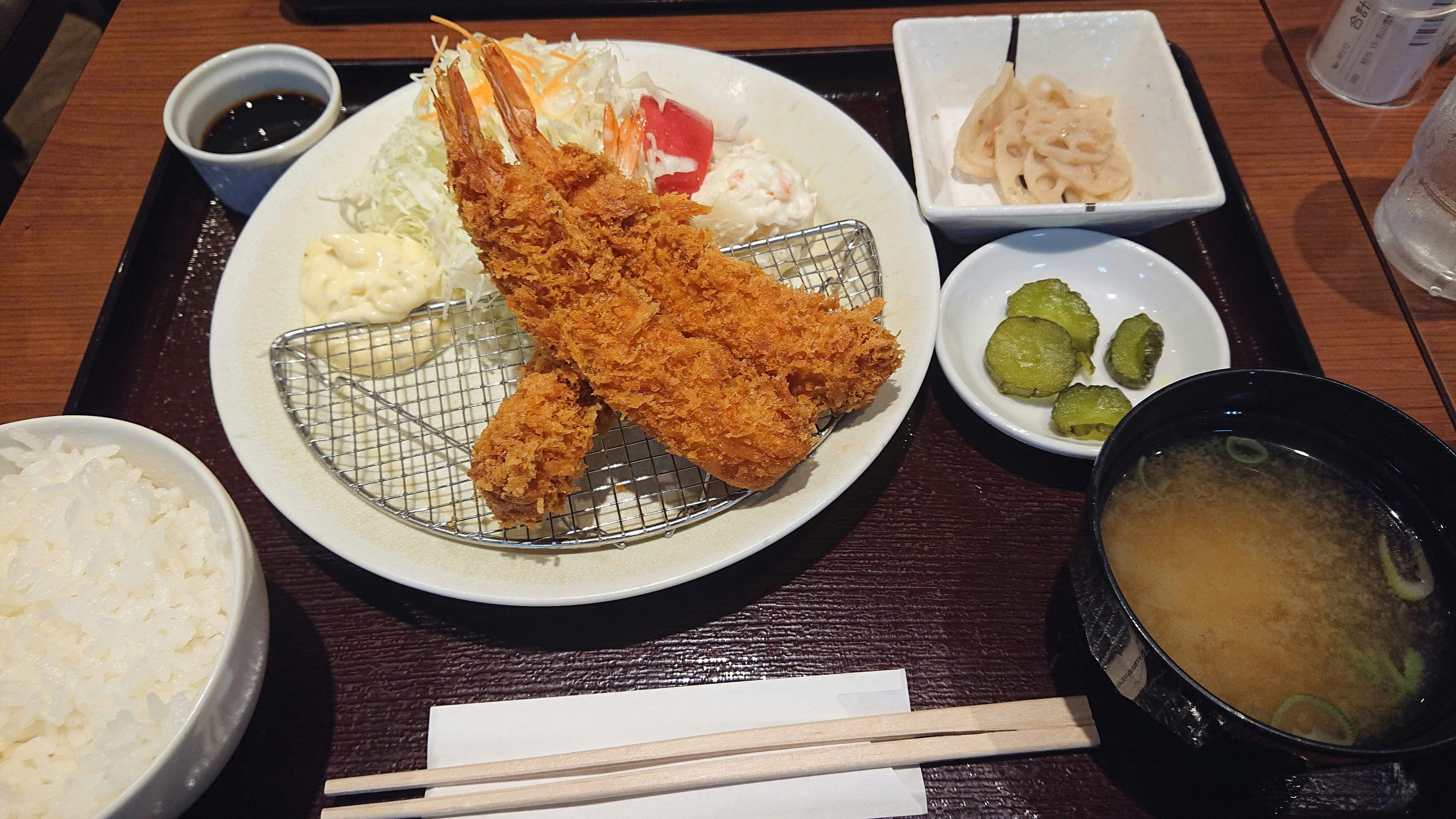
実行結果(エビフライ)
{
"labelAnnotations": [
{
"mid": "/m/02q08p0",
"description": "Dish",
"score": 0.9934035,
"topicality": 0.9934035
},
{
"mid": "/m/02wbm",
"description": "Food",
"score": 0.9903261,
"topicality": 0.9903261
},
{
"mid": "/m/01ykh",
"description": "Cuisine",
"score": 0.9864208,
"topicality": 0.9864208
},
{
"mid": "/m/0g9vs81",
"description": "Steamed rice",
"score": 0.9271187,
"topicality": 0.9271187
},
{
"mid": "/m/07xgrh",
"description": "Ingredient",
"score": 0.9207317,
"topicality": 0.9207317
},
{
"mid": "/m/0bp3f6m",
"description": "Fried food",
"score": 0.9098738,
"topicality": 0.9098738
},
{
"mid": "/m/0dxjn",
"description": "Deep frying",
"score": 0.9049985,
"topicality": 0.9049985
},
{
"mid": "/m/0f99t",
"description": "Tonkatsu",
"score": 0.901048,
"topicality": 0.901048
},
{
"mid": "/m/0krfg",
"description": "Meal",
"score": 0.81980187,
"topicality": 0.81980187
},
{
"mid": "/m/04q6ng",
"description": "Comfort food",
"score": 0.8160322,
"topicality": 0.8160322
}
]
}
Cloud Vision APIで食べ物の写真を判定した結果
牡蠣、寿司、ハンバーガーは食べ物であることだけでなく、種類まで特定できています。
エビフライは揚げ物であることは判定できていますが、「エビフライ」であることは判断できていないようです。
本記事にあげていない写真でも試しましたが、基本的には食べ物の種類まで特定できそうでした。
揚げ物というジャンルはわかりやすいものの、エビの要素が画像上わかりにくいエビフライと同条件の写真は種類の特定が難しいようでした。
最後に
今回はラベル検出を試しましたが、Cloud Vision APIでは画像の色合いの検出もできるようです。
食べ物の写真の色合いの傾向がつかめるとどのような色合いがおいしそうかがわかるかもしれないですね。
画像認識API自体はGoogle以外でも提供されているため、今後そちらも試す必要があると考えています。
-
深夜の時間帯などにおいしそうな食事の写真をアップすることで見るものを空腹にさせる行為 ↩