概要
- ローカルPCのUbutu VRAM環境(8GB)でStable Diffusionのfine tuning手法であるDreamBoothを動作させる方法を説明します.
- この記事を参考に、環境構築&動作確認を行った備忘禄です.
- DreamBoothによる学習は10〜20分程度、1024×768ピクセルの結果出力には1分程度でした.
- 以下は、栗駒こまるさんの3Dモデルから得られた画像をもとにwaifu-diffusionを学習させたときの出力サンプルです.
※3Dモデルのレンダリング画像のみで学習できるかどうかは、興味深いと思っているので別記事でまとめる予定です.
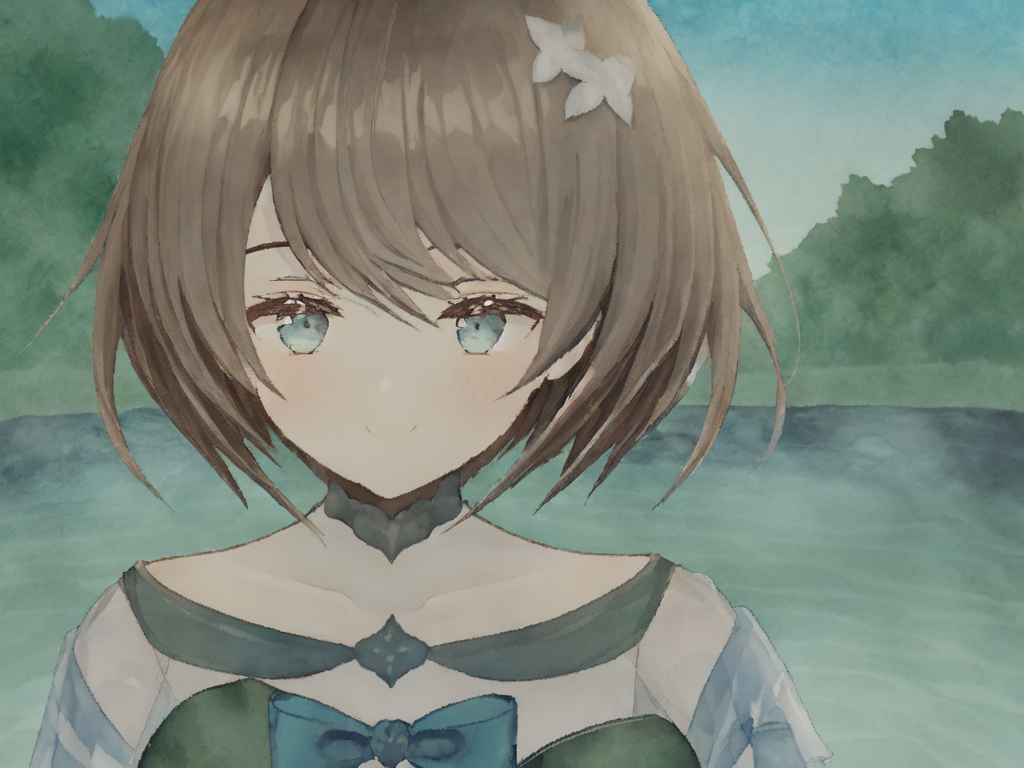

動作環境
-
OS : Ubuntu 22.04.1 LTS
-
ハード : OMEN by HP 40L Desktop GT21-0770jp ハイパフォーマンスモデル
- Gefoce RTX 3070 ( VRAM: 8GB )
- Core™ i7-12700K
- メモリ 32GB (8GB × 4) ※ 8GB×2を拡張済
-
Graphic Driver: 525.60.13
※ DreamBoothの学習においては、VRAM・RAM(メモリ)ともにギリギリの動作環境のようでした. ("学習実行"の節で述べますが、32GBではギリギリ足りておらず、スワップ領域を利用することになっています. )
※ また、Windowsはdeepspeedのinstallでコケます. 頑張ればできるかもしれませんが、いくつかissueを覗いたところ厳しそうでした. 私も以下と同じところでつまりました. wslでもエラーになるようです. 開発はmicrosoftなのになんで、、
https://github.com/microsoft/DeepSpeed/issues/2588
前提
https://huggingface.co/docs/diffusers/training/dreambooth
こちらの記事を確認すると、以下のように記述があります.
- VRAM 16GB: gradient checkpointingとbitsandbytesを活用して学習可能.
- VRAM 8GB: deepspeedを活用して、学習中のモデルパラメータやオプティマイザーの状態をCPUにオフロードすることで学習可能.
そのため以下の環境構築の節では、VRAM 8GBを前提に説明していますが、VRAM 16GBの場合も"deep speedのインストール"の直前まで行えば良いと思います.
環境構築
Python仮想環境作成
※ xformersのbuild済パッケージがPython3.7以上のため、Python3.7以降が推奨です.
python3 -m venv .venv
source .venv/bin/activate
python
Python 3.9.16 (main, Dec 27 2022, 06:19:10)
[GCC 11.3.0] on linux
Type "help", "copyright", "credits" or "license" for more information.
diffusersのインストール
pip install git+https://github.com/huggingface/diffusers
Pytorchのインストール https://pytorch.org/
pip install torch==1.13.0 torchvision torchaudio --extra-index-url https://download.pytorch.org/whl/cu117
※ xformersのbuild済パッケージがcuda11.6 or cuda11.7のため、どちらかが推奨です.
※ CUDA 11.xの互換性は、nvidia driverの 450.80.02以降なので気をつけましょう.
https://docs.nvidia.com/deploy/cuda-compatibility/index.html
Table 1. Example CUDA Toolkit 11.x Minimum Required Driver Versions (Refer to CUDA Release Notes)
CUDA Toolkit Linux x86_64 Minimum Required Driver Version Windows Minimum Required Driver Version
CUDA 12.x >=525.60.13 >=527.41
CUDA 11.x >= 450.80.02* >=452.39*
※ OS側にCUDAとCUDNNをインストールしていなくても、CUDA周りはpipで一緒にinstallしてくれます. 便利になっていますね.
kitsume@kitsume:~$ nvcc -v
コマンド 'nvcc' が見つかりません。次の方法でインストールできます:
sudo apt install nvidia-cuda-toolkit
xformersのインストール
build済のファイルをリンクの、"Artifacts"のところからダウンロードします.
※ 私はpython3.9 : torch1.13.0 : cuda11.7 なので以下をダウンロードしました.
xformers-ubuntu-22.04-py3.9-torch1.13.0+cu117.whl.zip
zipを解凍後whlファイルをinstall
pip install xformers-0.0.15.dev0+303e613.d20221128-cp39-cp39-linux_x86_64.whl
dreamboothに必要なパッケージのinstall
git clone https://github.com/huggingface/diffusers.git
diffusers/examples/dreambooth/requirements.txt のtorchvisionのコメントアウト ※コメントアウトしないと、上記で入れたpytorchが更新されてしまいます.
accelerate
#torchvision
transformers>=4.25.1
ftfy
tensorboard
modelcards
コメントアウト後、dreambooth exampleに必要なライブラリのインストール
pip install -U -r diffusers/examples/dreambooth/requirements.txt
deep speedのインストール
pip install deepspeed
deepspeedを使うことで、学習中のモデルパラメータやオプティマイザーの状態をCPUにオフロードすることができます.
accelerate configの設定
accelerate config
--------------------------------------------------------------------------------------------------------------------------------------------------------------------------------------------
In which compute environment are you running?
This machine
--------------------------------------------------------------------------------------------------------------------------------------------------------------------------------------------
Which type of machine are you using?
No distributed training
Do you want to run your training on CPU only (even if a GPU is available)? [yes/NO]:no
Do you wish to optimize your script with torch dynamo?[yes/NO]:no
Do you want to use DeepSpeed? [yes/NO]: yes
Do you want to specify a json file to a DeepSpeed config? [yes/NO]: no
--------------------------------------------------------------------------------------------------------------------------------------------------------------------------------------------
What should be your DeepSpeed's ZeRO optimization stage?
2
--------------------------------------------------------------------------------------------------------------------------------------------------------------------------------------------
Where to offload optimizer states?
cpu
--------------------------------------------------------------------------------------------------------------------------------------------------------------------------------------------
Where to offload parameters?
cpu
How many gradient accumulation steps you're passing in your script? [1]:
Do you want to use gradient clipping? [yes/NO]: yes
What is the gradient clipping value? [1.0]:
Do you want to enable `deepspeed.zero.Init` when using ZeRO Stage-3 for constructing massive models? [yes/NO]: yes
How many GPU(s) should be used for distributed training? [1]:1
--------------------------------------------------------------------------------------------------------------------------------------------------------------------------------------------
Do you wish to use FP16 or BF16 (mixed precision)?
fp16
accelerate configuration saved at /home/kitsume/.cache/huggingface/accelerate/default_config.yaml
swap領域の拡張
System RAMは25GB程度必要と記述がありますが、自分の32GB環境ではMemory outしてしまいました。
The drawback is that this requires more system RAM (about 25 GB).
https://huggingface.co/docs/diffusers/training/dreambooth
そこで、swap領域で耐えられるように拡張が必要です.
Ubuntuのデフォルトではswap領域が2GBのため、以下の記事を参考にして64GBに拡張しました。※ 16GB程度で十分です.
https://qiita.com/hidenorly/items/563e65e98492f0094d3c
動作確認
train_dreambooth.pyの準備
学習のためには、diffusers/examples/train_dreambooth.py スクリプトを使います.
また、以下の記述を参考にoptimizerをdeepspeed.ops.adam.DeepSpeedCPUADAMに変更します.
Changing the default Adam optimizer to DeepSpeed’s special version of Adam deepspeed.ops.adam.DeepSpeedCPUAdam gives a substantial speedup,
#optimizer_class = torch.optim.AdamW
optimizer_class = deepspeed.ops.adam.DeepSpeedCPUAdam
※import deepspeedが必要です.
※このoptimizerの変更により劇的にメモリ効率 & 速度が改善されました.
train.shの準備
- 正規化画像を使用する場合もあるようですが、今回はインスタンス画像のみでの学習を行います.
export MODEL_NAME="CompVis/stable-diffusion-v1-4"
export INSTANCE_DIR="input_images/instance_images/dog"
export OUTPUT_DIR="model/dog"
accelerate launch train_dreambooth.py \
--pretrained_model_name_or_path=$MODEL_NAME \
--instance_data_dir=$INSTANCE_DIR \
--output_dir=$OUTPUT_DIR \
--instance_prompt="sks dog" \
--resolution=512 \
--train_batch_size=1 \
--sample_batch_size=1 \
--gradient_accumulation_steps=1 --gradient_checkpointing \
--learning_rate=5e-6 \
--lr_scheduler="constant" \
--lr_warmup_steps=0 \
--max_train_steps=300 \
--mixed_precision=fp16 \
--checkpointing_steps=100
finetuning用の画像の準備
こちらからダウンロードします.
https://drive.google.com/drive/folders/1BO_dyz-p65qhBRRMRA4TbZ8qW4rB99JZ
ディレクトリ構造は好みですが、以下のようにしています.
├── train_dreambooth.py
├── train.sh
├── inference.py
├── input_images
│ └── instance_images
│ └── dog
├── model
│ ├── dog
└── out_images
学習実行
./train.sh
- 以下のように、メモリがぎりぎり足りずにスワップ領域を借りています.
- また、スクショから2.5s/itになっていますが、スワップ領域に映る前は1.2s/it程度でした. つまり、メモリを増やしてスワップ領域にいかないようにすると、学習速度は倍になると思います.
- 学習中のGPUのVRAMは以下です. 8GB VRAMでギリギリであることがわかります.
kitsume@kitsume:~$ nvidia-smi
Fri Jan 6 17:27:51 2023
+-----------------------------------------------------------------------------+
| NVIDIA-SMI 525.60.13 Driver Version: 525.60.13 CUDA Version: 12.0 |
|-------------------------------+----------------------+----------------------+
| GPU Name Persistence-M| Bus-Id Disp.A | Volatile Uncorr. ECC |
| Fan Temp Perf Pwr:Usage/Cap| Memory-Usage | GPU-Util Compute M. |
| | | MIG M. |
|===============================+======================+======================|
| 0 NVIDIA GeForce ... On | 00000000:01:00.0 On | N/A |
| 33% 40C P2 121W / 220W | 7348MiB / 8192MiB | 94% Default |
| | | N/A |
+-------------------------------+----------------------+----------------------+
+-----------------------------------------------------------------------------+
| Processes: |
| GPU GI CI PID Type Process name GPU Memory |
| ID ID Usage |
|=============================================================================|
| 0 N/A N/A 1868 G /usr/lib/xorg/Xorg 237MiB |
| 0 N/A N/A 2003 G /usr/bin/gnome-shell 47MiB |
| 0 N/A N/A 8138 C+G ...234821957111671857,131072 158MiB |
| 0 N/A N/A 8786 G ...RendererForSitePerProcess 59MiB |
| 0 N/A N/A 16518 C ...ffusion/.venv/bin/python3 6812MiB |
+-----------------------------------------------------------------------------+
- 最終的に15分程度で学習は完了しました.
推論確認
- sks dogにサングラスをかけさせてみます.
prompt = "a photo of sks dog wearing sunglasses."
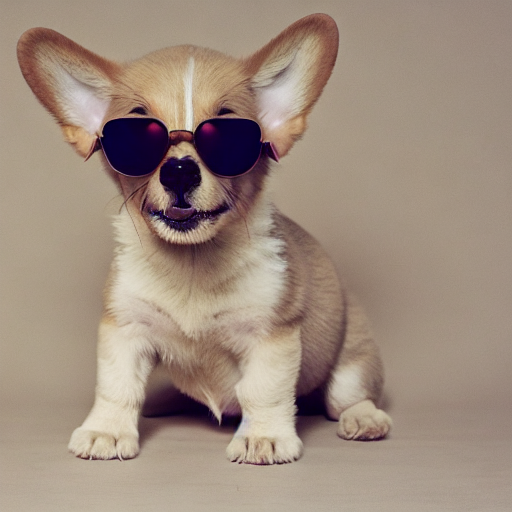
まとめと感想
- DreamBoothを8GB VRAM環境で動作させる方法を説明しました.
- 15分程度で学習が完了させることができ、動作環境を意識した技術およびライブラリの発展を感じました. 少数の画像から非常に汎化できるようでめちゃくちゃすごいですね、、正直恐ろしすぎます、、
- 冒頭でも述べましたが、3Dモデルから得た画像からどの程度学習できるか、過学習や汎化の様子を"実験的"で"定性的"に別記事でまとめる予定です.
- 本記事のスクリプトは、https://github.com/kitsume-hy/DreamBooth-waifu-diffusion-test/tree/dog にまとめています.
何かありましたら、雑にコメントください.
参考ページ
https://huggingface.co/docs/diffusers/training/dreambooth
https://github.com/facebookresearch/xformers/issues/543
https://huggingface.co/docs/diffusers/training/dreambooth