この記事は、強化学習の代表的なアルゴリズムであるSarsaとQ学習、2つのアルゴリズムの違いについてのまとめです。
SarsaとQ学習の違いは一言で言うと、Q関数の更新にエージェントのポリシー(方策)を用いるかどうか、です。まず、ポリシーとQ関数の関係について確認しておきます。
ポリシーとは、ある状態$s$が与えられたときにとる行動を返す関数$\pi(s)$です。次に得る利得が最も高くなりそうなポリシーは、基本的に状態$s$と行動$a$によって定まるQ関数$Q(s,a)$を$a$について最大化するようなものでしょう (greedy法):
$$\pi (s) := \rm{arg} \max_a Q(s,a)
$$
しかし、このポリシーではQ関数の初期値によってはまだとったことのない行動をとらないまま局所解に陥ってしまうかもしれません。そこで、例えば確率$\varepsilon$ですべての行動から一様にランダムに行動を決める、というポリシーも考えられます (ε-greedy法)。これは、$[0,1)$の一様乱数$x$を使って、
\pi'(s) :=
\left\{
\begin{array}{ll}
{\rm arg}\max_a Q(s,a) & {\rm if} \ x > \varepsilon \\
{\rm select} \ a \ {\rm randomly} & {\rm else}
\end{array}
\right.
ということです。この他にも、様々な観点からみた良いポリシーが多くあります。ポイントは、Q関数にもとづいてどのようなポリシーをとるかはアルゴリズムの設計者が決めるということです。
これを踏まえたうえで、SarsaとQ学習の違いを具体的に見ていきます。Sarsaでは現在の状態$S$とそこでとる行動$A$、行動によって得られた利得$R$、次の状態$S'$が分かったときに、現在の状態と行動についてのQ関数$Q(S,A)$の更新を、次の状態$S'$と$S'$が与えられたときポリシーによって決めたエージェントがとる行動$A'$によって決まるQ関数$Q(S',A')$を使って行います。$(S,A,R,S',A')$の組を使ってQ関数を更新するので、Sarsaと呼ばれます。アルゴリズムの疑似コードは次のようになります( [1] から引用)
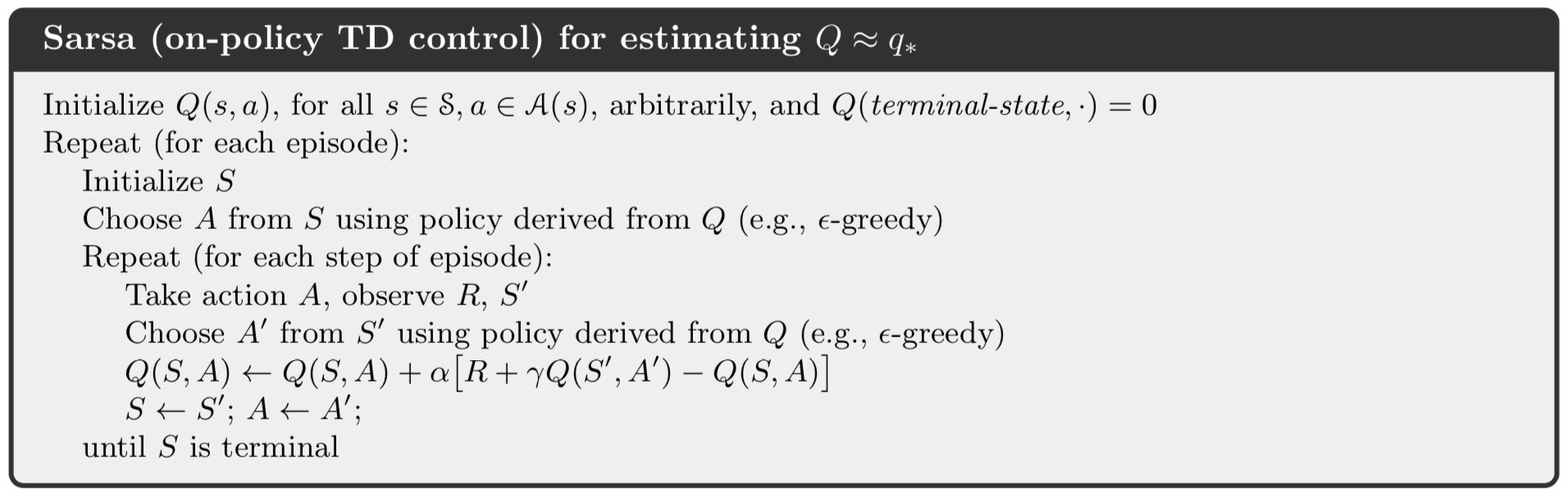
一方、Q学習では$(S,A,R,S')$が分かったときのQ関数の更新に、$S'$が与えられた時のQ関数の最大値を使います。アルゴリズムの疑似コードは次のようになります( [1] から引用)
疑似コードから、もしポリシーにgreedy法を採用した場合、SarsaとQ学習は一致することが分かります。
実装の例として、[2] の記事のCardPoleの学習をQ学習で行ったコードをSarsaに変更し、jupyter notebookで実行しやすいように改変したものを以下にのせておきます。
import matplotlib.pyplot as plt
# Imports specifically so we can render outputs in Jupyter.
from JSAnimation.IPython_display import display_animation
from matplotlib import animation
from IPython.display import display
import gym
import numpy as np
import random
import math
from time import sleep
def display_frames_as_gif(frames):
"""
Displays a list of frames as a gif, with controls
"""
patch = plt.imshow(frames[0])
plt.axis('off')
def animate(i):
patch.set_data(frames[i])
anim = animation.FuncAnimation(plt.gcf(), animate, frames = len(frames), interval=50)
display(display_animation(anim, default_mode='loop'))
## Initialize the "Cart-Pole" environment
env = gym.make('CartPole-v0')
## Defining the environment related constants
# Number of discrete states (bucket) per state dimension
NUM_BUCKETS = (1, 1, 6, 3)
# Number of discrete actions
NUM_ACTIONS = env.action_space.n
# Bounds for each discrete state
STATE_BOUNDS = list(zip(env.observation_space.low, env.observation_space.high))
STATE_BOUNDS[3] = [-math.radians(50), math.radians(50)]
# Index of the action
ACTION_INDEX = len(NUM_BUCKETS)
## Creating a Q-Table for each state-action pair
q_table = np.zeros(NUM_BUCKETS + (NUM_ACTIONS,))
## Learning related constants
MIN_EXPLORE_RATE = 0.01
MIN_LEARNING_RATE = 0.1
## Defining the simulation related constants
NUM_EPISODES = 1000
MAX_T = 250
STREAK_TO_END = 120
SOLVED_T = 199
def simulate():
## Instantiating the learning related parameters
learning_rate = get_learning_rate(0)
explore_rate = get_explore_rate(0)
discount_factor = 0.99 # since the world is unchanging
num_streaks = 0
for episode in range(NUM_EPISODES):
# Reset the environment
obv = env.reset()
# the initial state
state = state_to_bucket(obv)
action = select_action(state, explore_rate)
for t in range(MAX_T):
# Execute the action
obv, reward, done, _ = env.step(action)
# Observe the result
next_state = state_to_bucket(obv)
next_action = select_action(next_state, explore_rate)
# Update the Q based on the result
next_q = q_table[next_state+(next_action,)]
q_table[state + (action,)] += learning_rate*(reward + discount_factor*(next_q) - q_table[state + (action,)])
# Setting up for the next iteration
state = next_state
action = next_action
if done:
print("Episode %d finished after %f time steps" % (episode, t))
if (t >= SOLVED_T):
num_streaks += 1
else:
num_streaks = 0
break
# It's considered done when it's solved over 120 times consecutively
if num_streaks > STREAK_TO_END:
break
# Update parameters
explore_rate = get_explore_rate(episode)
learning_rate = get_learning_rate(episode)
def select_action(state, explore_rate):
# Select a random action
if random.random() < explore_rate:
action = env.action_space.sample()
# Select the action with the highest q
else:
action = np.argmax(q_table[state])
return action
def get_explore_rate(t):
return max(MIN_EXPLORE_RATE, min(1, 1.0 - math.log10((t+1)/25)))
def get_learning_rate(t):
return max(MIN_LEARNING_RATE, min(0.5, 1.0 - math.log10((t+1)/25)))
def state_to_bucket(state):
bucket_indice = []
for i in range(len(state)):
if state[i] <= STATE_BOUNDS[i][0]:
bucket_index = 0
elif state[i] >= STATE_BOUNDS[i][1]:
bucket_index = NUM_BUCKETS[i] - 1
else:
# Mapping the state bounds to the bucket array
bound_width = STATE_BOUNDS[i][1] - STATE_BOUNDS[i][0]
offset = (NUM_BUCKETS[i]-1)*STATE_BOUNDS[i][0]/bound_width
scaling = (NUM_BUCKETS[i]-1)/bound_width
bucket_index = int(round(scaling*state[i] - offset))
bucket_indice.append(bucket_index)
return tuple(bucket_indice)
simulate()
obs_current = env.reset()
state = state_to_bucket(obs_current)
frames = []
for i in range(1000):
frames.append(env.render(mode = 'rgb_array'))
action = select_action(state, MIN_EXPLORE_RATE)
obs_next, reward, done, info = env.step(action)
if done:
print(i)
break
state = state_to_bucket(obs_next)
env.render(close=True)
display_frames_as_gif(frames)