ストラング:線形代数イントロダクション原書第4版の「行列の構成」についてその意味と意図が今一歩よくわかりませんでした。今回2024年2月8日の修正は2回目の大々的な修正です。4版ではすでにこの節を理解することは不可能と思い、6版の関連部分を全面翻訳することにしました。6版の7.2節を翻訳し、その後に従来通り応用へのコメントをします。「行列の構成」を理解するカギはAを構成する方法にあるのではなく、基底と成分について理解することでした。
間違い、理解不足等があると思いますので、コメントいただけると助かります。
以下翻訳です。翻訳はChatGPTにより行い、若干の修正を加えました。原文はAPPENDIXです。
8.2 The Matrix of a Linear Transformationの訳
"""翻訳
次の項**"基底の変換: 行列B"** では、すべての線形変換 $T$ に対して行列 $A$ を割り当てます。通常の列ベクトルにおいて、入力 $\boldsymbol{v}$ は $V=R^n$ にあり、出力 $T(\boldsymbol{v})$ は $W=R^m$ にあります。この変換に対する行列は $m$ 行 $n$ 列になります。$V$ と $W$ での基底の選択が $A$ を決定します。
$R^n$ と $R^m$ の標準基底ベクトルは、単位行列 $I$ の列です。その選択は標準行列につながります。すると通常の方法で $T(\boldsymbol{v})=A\boldsymbol{v}$ となります。しかし、これらの空間には異なる基底も存在するので、同じ変換 $T$でも別の行列によって表現されます。線形代数の主要なテーマは、$T$ に対して最良の行列(対角行列)を与える基底を選択することです。
すべてのベクトル空間 $V$ と $W$ には基底があります。それらの基底の各選択は、$T$ に対する行列につながります。入力基底が出力基底と異なる場合、$T(\boldsymbol{v})$ のための行列は単位行列 $I$ にはなりません。それは「基底の変換行列」になります。ここに重要な考え方があります:
入力基底ベクトル $\boldsymbol{v}_1$ から $\boldsymbol{v}_n$ に対する出力 $T(\boldsymbol{v})$ を知っていると仮定します。行列の列 1 から $n$ には、それらの出力 $T(\boldsymbol{v}_1),\cdots,T(\boldsymbol{v}_n)$ が含まれます。$A \times c=$ 行列 $\times$ ベクトル = それらの $n$ 列の組み合わせ。
$Ac$ は正しい組み合わせ $c_1T(v_1)+\cdots+c_nT(v_n)=T(v)$ を与えます。
理由:すべての $\boldsymbol{v}$ は基底ベクトル $\boldsymbol{v}_j$ の一意の組み合わせ $c_1\boldsymbol{v}_1+\cdots+c_n \boldsymbol{v}_n$ です。
$T$ が線形変換である(ここが線形性のポイント)ので、$T(\boldsymbol{v})$ は出力 $T(\boldsymbol{v}_j)$ の同じ組み合わせ $c_1T(\boldsymbol{v}_1)+\cdots+c_nT(\boldsymbol{}v_n)$ でなければなりません。
最初の例として、$R^2$ と $R^3$ の標準基底ベクトルに対する行列 $A$ を考えます。
$A$ の二つの列は、$\boldsymbol{v}_1=(1,0)$ と $\boldsymbol{v}_2=(0,1)$ から出力を得ます。
例1:
$T$ が $\boldsymbol{v}_1=(1,0)$ を $T(\boldsymbol{v}_1)=(2,3,4)$ に変換し、第二の基底ベクトル $\boldsymbol{v}_2=(0,1)$ が $\boldsymbol{v}_2=(5,5,5)$ になると仮定します。$T$ が $R^2$ から $R^3$ への線形変換であれば、その「標準行列」は 3 行 2 列になります。これらの出力 $T(\boldsymbol{v}_1)$ と $T(\boldsymbol{v}_2)$ は $A$ の列に入ります:
A = \begin{bmatrix} 2 & 5 \\ 3 & 5 \\ 4 & 5 \end{bmatrix} c_1=1 \text{および } c_2 =1 \text{は } T(v_1+v_2)=\begin{bmatrix}
2 & 5 \\ 3 & 5 \\ 4 & 5 \end{bmatrix}\begin{bmatrix}1 \\ 1 \end{bmatrix}=\begin{bmatrix}7 \\ 8 \\ 9 \end{bmatrix}
を与えます。
基底の変換:行列 B
例2:
入力空間 $V=R^2$ が出力空間 $W=R^2$ と同じであると仮定します。$T(\boldsymbol{v})=\boldsymbol{v}$ が恒等変換である場合、その行列は $I$(単位行列)になると期待するかもしれません。しかし、それは入力基底が出力基底と同じ場合にのみ起こります。異なる基底を選択して、行列がどのように構築されるかを見てみましょう。
この特別なケース $T(\boldsymbol{v})=\boldsymbol{v}$ について、行列を $A$ ではなく $B$ と呼ぶことにします。これは単に $\boldsymbol{v}$ から $\boldsymbol{w}$ への基底の変換です。それぞれが $\boldsymbol{w}_1$ と $\boldsymbol{w}_2$ の組み合わせです。
\begin{matrix} 入力\\基底 \end{matrix} \begin{bmatrix} \boldsymbol{v}_1 & \boldsymbol{v}_2 \end{bmatrix}=\begin{bmatrix} 3&6\\3&8 \end{bmatrix}\begin{matrix} 出力\\基底 \end{matrix} \begin{bmatrix} \boldsymbol{w}_1 & \boldsymbol{w}_2 \end{bmatrix}=\begin{bmatrix} 3&0\\1&2 \end{bmatrix}\begin{matrix} 基底の\\変換 \end{matrix} \begin{bmatrix} \boldsymbol{v}_1 =\mathbf{1} \boldsymbol{w}_1+\mathbf{1}\boldsymbol{w}_2\\\boldsymbol{v}_2=\mathbf{2}\boldsymbol{w}_1+\mathbf{3}\boldsymbol{w}_2 \end{bmatrix}
注意してください!入力基底 $\boldsymbol{v}_1, \boldsymbol{v}_2$ を出力基底 $\boldsymbol{w}_1, \boldsymbol{w}_2$ の観点から記述しました。これは我々の重要なルールのためです。恒等変換 $T$ を各入力基底ベクトルに適用します:$T(\boldsymbol{v}_1)=\boldsymbol{v}_1$ および $T(\boldsymbol{v}_2)=\boldsymbol{v}_2$。次に、これらの出力 $\boldsymbol{v}_1$ と $\boldsymbol{v}_2$ を出力基底 $\boldsymbol{w}_1$ と $\boldsymbol{w}_2$ で記述します。これらの太字の数字 1, 1 と 2, 3 は行列 $B$ (基底の変換行列)の列 1 と列 2 となります:$WB=V$ なので $B=W^{-1}V$ です。
基底の変換に対する行列 $B$
\begin{matrix} \text{基底の変換に対する} \\ \text{行列 } B \end{matrix} \begin{bmatrix} \\ \boldsymbol{w}_1 \boldsymbol{w}_2\\ \;\end{bmatrix}=\begin{bmatrix} \\B\\ \; \end{bmatrix}\begin{bmatrix} \boldsymbol{v}_1 \boldsymbol{v}_2 \end{bmatrix}\text{ は } \begin{bmatrix} 3&0\\1&2 \end{bmatrix}\begin{bmatrix} \mathbf{1}&\mathbf{2}\\ \mathbf{1}&\mathbf{3} \end{bmatrix}= \begin{bmatrix} 3&6\\3&8 \end{bmatrix} \;\;\;(1)
入力基底が行列 $V$ の列にあり、出力基底が $W$ の列にあるとき、$T=I$ の基底の変換行列は $B=W^{-1}V$ です。
私が見た鍵は、そのルール $B=W^{-1}V$ を理解する明確な方法です。同じベクトル $\boldsymbol{u}$ を入力基底の $\boldsymbol{v}$ と出力基底の $\boldsymbol{w}$ で書くとします。これを三通りの方法で行います:
\begin{matrix} \boldsymbol{u}=c_1\boldsymbol{v}_1+\cdots+c_n\boldsymbol{v}_n\\ \boldsymbol{u}=d_1\boldsymbol{w}_1+\cdots+d_n\boldsymbol{w}_n \end{matrix}\text{ は } \begin{bmatrix} \\ \boldsymbol{v}_1 \cdots \boldsymbol{v}_n\\ \;\end{bmatrix}\begin{bmatrix} c_1\\ \vdots \\ c_n \end{bmatrix}=\begin{bmatrix} \boldsymbol{w}_1 \cdots \boldsymbol{w}_n \end{bmatrix}\begin{bmatrix} d_1\\ \vdots \\d_n \end{bmatrix} \text{ そして }V\boldsymbol{c}=W\boldsymbol{d}
新しい基底 $\boldsymbol{w}$ の係数 $\boldsymbol{d}$ は $\boldsymbol{d}=W^{-1}V\boldsymbol{c}$ です。そして $B$ は
W^{-1}V \;\;\;(2)
この式 $B=W^{-1}V$ は世界の最大の謎の一つを生み出します:標準基底 $V=I$ が異なる基底 $W$ に変換されると、基底の変換行列は $W$ ではなく $B=W^{-1}$ になります。大きな基底ベクトルはより小さい係数を持ちます!
\text{標準基底における
}
\begin{bmatrix} x\\y \end{bmatrix}
\text{は、} \boldsymbol{w}_1, \boldsymbol{w}_2 \text{ 基底において }\begin{bmatrix} \boldsymbol{w}_1 &\boldsymbol{w}_2 \end{bmatrix}^{-1}\begin{bmatrix} x\\y \end{bmatrix}\text{ の係数を持ちます。}
行列の構成ためのT
今度は任意の線形変換に対する行列を構築します。線形変換 $ T $ が $ n $ 次元の空間 $ \mathbf{V} $ を $ m $ 次元の空間 $ \mathbf{W} $ に変換すると仮定します。$ \mathbf{V} $ の基底として $ \mathbf{v}_1, \ldots, \mathbf{v}_n $ を、$ \mathbf{W} $ の基底として $ \mathbf{w}_1, \ldots, \mathbf{w}_m $ を選びます。行列 $ A $ のサイズは $ m \times n $ となります。$ A $ の最初の列を見つけるためには、最初の基底ベクトル $ \mathbf{v}_1 $ に $ T $ を適用します。出力 $ T(\mathbf{v}_1) $ は $ \mathbf{W} $ 内にあります。$T(\mathbf{v}_1)$ は $\mathbf{W}$ の出力基底に対する
$ a_{11}\mathbf{w_1} + \ldots + a_{m1}\mathbf{w_m} $ の組み合わせです。
これらの数値 $ a_{11}, \ldots, a_{m1} $ は $ A $ の最初の列に入ります。$ \mathbf{v}_1 $ を $ T(\mathbf{v}_1) $ に変換することは、$ (1,0, \ldots ,0) $ に $ A $ を掛けることと一致します。これが行列の最初の列を生成します。$ T $ が導関数で最初の基底ベクトルが 1 の場合、その導関数は $ T(\mathbf{v_1})= 0 $ です。したがって、導関数行列の $ A $ の最初の列はすべてゼロです。
例3
入力基底 $ \mathbf{v} $ は $ 1,x,x^2,x^3 $ です。出力基底 $ \mathbf{w} $ は $ 1,x,x^2 $ です。そして $ T $ は導関数を取ります:$ T(\mathbf{v}) = \frac{d\mathbf{v}}{dx} $ であり、$ A $ は「導関数行列」です。
もし $ \mathbf{v} = c_1 + c_2x + c_3x^2 + c_4x^3 $ ならば、$ \frac{d\mathbf{v}}{dx} = c_2 + 2c_3x + 3c_4x^2 $ です。
Ac =
\begin{bmatrix}
0&1&0&0 \\
0&0&2&0 \\
0&0&0&3
\end{bmatrix}
\begin{bmatrix}
c_1 \\
c_2 \\
c_3 \\
c_4
\end{bmatrix}
=
\begin{bmatrix}
c_2 \\
2c_3 \\
3c_4
\end{bmatrix}
キーとなるルール:$ A $ の $ j $ 番目の列は $ j $ 番目の基底ベクトル $ \mathbf{v}_j $ に $ T $ を適用することで見つかります。
$ T(\mathbf{v}_j) $ は基底ベクトルの組み合わせであり、
a_{1j}\mathbf{w}_1 + \cdots + a_{mj} \mathbf{w}_m
です。
これらの数値 $ a_{ij} $ は $ A $ に入ります。行列は基底ベクトルを正確に構築します。そして、線形性により他のすべてのベクトルも正確に反映されます。すべての $ \mathbf{v} $ は $ c_1 \mathbf{v}_1 + \ldots + c_n \mathbf{v}_n $ の組み合わせであり、$ T(\mathbf{v}) $ も $ \mathbf{w} $ の組み合わせです。$ A $ に $ \mathbf{v} $ の組み合わせである係数ベクトル $ c = (c_1, \ldots , c_n) $ を掛けると、$ Ac $ は $ T(\mathbf{v}) $ の係数を生成します。これは行列の積(列の結合)が $ T $ のように線形であるためです。
$ \mathbf{V} $ から $ \mathbf{W} $ へのすべての線形変換は、基底を使用して行列に変換されます。
例 4
積分 $T^{+}(v)$ において、最初の基底関数は再び 1 です。その積分は2番目の基底関数 $x$ です。したがって、「積分行列」 $A^+$ の最初の列は $(0, 1, 0, 0)$ です。
\begin{matrix} \text{積分 }d_1+d_2x+d_3x^2\\
\text{は } d_1x+\frac{1}{2}d_2x^2 + \frac{1}{3}d_3x^3 \text{です} \end{matrix}
A^+d=\begin{bmatrix}0&0&0\\1&0&0\\0&\frac{1}{2} &0\\0&0&\frac{1}{3} \end{bmatrix}\begin{bmatrix}d_1\\d_2\\d_3 \end{bmatrix}=\begin{bmatrix}0\\d_1\\\frac{1}{2}d_2\\ \frac{1}{3}d_3 \end{bmatrix}
関数を積分してから微分すると、元に戻ります。つまり $AA^+=I$ です。しかし、微分してから積分すると、定数項が失われます。したがって $A^+A$ は $I$ ではありません。
1の導関数の積分はゼロです:
$$T^+T(1)=\text {ゼロ関数の積分 = 0}$$
これは $A^+A$ に一致します。その最初の列はすべてゼロです。導関数 $T$ には核(定数関数)があります。その行列には零空間があります。主なアイデアは再び: $Av$ は $T(v)$ のコピーです。
導関数と積分の例では3つのポイントを示しました。第一に、線形変換 $T$ は、微積分、微分方程式、線形代数の至る所に存在します。第二に、$R^n$ 以外の空間も重要です。私たちは $V$ と $W$ で関数を扱いました。第三に、微分してから積分すると、それらの行列 $A^+A$ を乗算することができます。
行列積 AB と変換 TS の一致
私たちは重要なことにたどり着きました — 行列を掛け合わせるルールの本当の理由です。ついにその理由がわかります!2つの線形変換 $T$ と $S$ は、2つの行列 $A$ と $B$ によって表されます。これから、$TS$ と積 $AB$ を比較します:
変換 $T$ を $S$ の出力に適用するとき、次のルールによって $TS$ を得ます:$(TS)(\boldsymbol{u})$ は $T(S(\boldsymbol{u}))$ と定義されます。出力 $S(\boldsymbol{u})$ は $T$ への入力となります。
行列 $A$ を $B$ の出力に適用するとき、このルールによって $AB$ を掛け合わせます:$(AB)(\boldsymbol{x})$ は $A(B\boldsymbol{x})$ と定義されます。出力 $B\boldsymbol{x}$ は $A$ への入力となります。行列の積は、$TS$ を表す正しい行列 $AB$ を与えます。
変換 $S$ は空間 $U$ から $V$ へのもので、その行列 $B$ は $U$ の基底 $\boldsymbol{u}_1,\cdots,\boldsymbol{u}_p$ と $V$ の基底 $\boldsymbol{v}_1,\cdots, \boldsymbol{v}_n$ を使用します。その行列は $n$ 行 $p$ 列です。変換 $T$ は以前と同様に $V$ から $W$ へのものです。その行列 $A$ は、出力空間である $S$ と入力空間である $T$ のために同じ基底 $\boldsymbol{v}_1,\cdots, \boldsymbol{v}_n$ を使用しなければなりません。そして行列 $AB$ は $TS$ に一致します。
積の変換 $TS$ は、任意のベクトル $\boldsymbol{u}$ が $U$ から始まり、$V$ の $S(\boldsymbol{u})$ へ行き、そして $W$ の $T(S(\boldsymbol{u}))$ へ行きます。行列 $AB$ は、任意の $\boldsymbol{x}$ が $R^P$ から始まり、$R^n$ の $B\boldsymbol{x}$ へ行き、そして $R^m$ の $AB\boldsymbol{x}$ へ行きます。行列 $AB$ は $TS$ を正確に表しています:
$$TS:U→V→W ; AB:(m \text{ 行 } n)(n \text{ 行 } p)=(m \text{ 行 } p)$$
変換の積 $TS$ は行列の積 $AB$ と一致します。重要なケースは、空間 $U,V,W$ が同じで、その基底が同じである場合です → 正方行列。
例5
$S$ は平面を $\theta$ だけ回転させ、$T$ も $\theta$ だけ回転させます。すると $TS$ は $2\theta$ だけ回転します。この変換 $T^2$ は、$2\theta$ を通じて回転行列 $A^2$ に対応します:
T=S \;\; A=B \;\;\; T^2 = 2\theta \text{ だけの回転 } \;\;\; A^2=\begin{bmatrix}\cos(2\theta) & -\sin(2\theta) \\ \sin(2\theta) & \cos(2\theta) \end{bmatrix} \;\;\; (4)
A^2=\begin{bmatrix}\cos(\theta) & -\sin(\theta) \\ \sin(\theta) & \cos(\theta) \end{bmatrix}\begin{bmatrix}\cos(\theta) & -\sin(\theta) \\ \sin(\theta) & \cos(\theta) \end{bmatrix}
=\begin{bmatrix}\cos^2(\theta)- \sin^2(\theta) & - 2\sin(\theta)\cos(\theta) \\ 2\sin(\theta)\cos(\theta)& \cos^2(\theta)-\sin^2(\theta) \end{bmatrix} \;\;\; (5)
(4) と (5) を比較すると、$\cos 2\theta = \cos^2 \theta -\sin^2 \theta$ と $\sin 2\theta=2\sin \theta \cos \theta$ が得られます。
例6
$S$ は角度 $\theta$ で回転し、$T$ は角度 $-\theta$ で回転します。すると $TS=I$ になり、これは $AB=I$ につながります。この場合、$T(S(\boldsymbol{u}))$ は $\boldsymbol{u}$ です。前方と後方に回転します。行列が一致するためには、$AB\boldsymbol{x}$ は $\boldsymbol{x}$ でなければなりません。角度 $\theta$ と $-\theta$ の行列は互いに逆行列です。
最適な基底の選択
本節の最終ステップに到達します。行列を対角化する基底を選択します。標準基底($I$ の列)を使用すると、変換 $T$ はある行列 $A$ を生成しますが、おそらく対角ではありません。異なる基底を選択すると、同じ $T$ は異なる行列によって表されます。2つの偉大な選択肢は、固有ベクトルと特異ベクトルです:
ー固有ベクトルー
$T$ が $R^n$ から $R^n$ への変換である場合、その行列 $A$ は正方形です。$n$ 個の独立した固有ベクトルがある場合、それらを入力と出力の基底として選択します。この良い基底では、$T$ のための行列は対角固有値行列 $\Lambda$ です。
例7
射影行列 $T$ は、$R^2$ のすべての $\boldsymbol{v}=(x,y)$ を線 $y=-x$ 上に射影します。標準基底を使用して、$\boldsymbol{v}_1=(1,0)$ は $T(\boldsymbol{v}_1)=\left(\frac{1}{2},-\frac{1}{2}\right)$ に射影されます。$ \boldsymbol{v}_2=(0,1)$ に対する射影は $T(\boldsymbol{v}_2)=\left(-\frac{1}{2},\frac{1}{2}\right)$ です。これらは $A$ の列です:
\begin{matrix}\text{射影行列}\\\text{標準基底}\\ \text{対角ではない} \end{matrix} A=\begin{bmatrix} \frac{1}{2}&-\frac{1}{2}\\-\frac{1}{2}&\frac{1}{2}\end{bmatrix} \text{ は } A^T=A \text{ および } A^2=A \text{ を満たします。}
つぎは固有ベクトルの重要な利用法です。それらを基底ベクトルにします!対角化するためです!
ー基底ベクトル=固有ベクトルー
基底ベクトルが固有ベクトルの場合、行列は対角行列になります。
\begin{matrix}
\boldsymbol{v}_1&=\boldsymbol{w}_1&=(1,ー1)&\text{ はそれ自身に射影されます: } &T(\boldsymbol{v}_1)=\boldsymbol{v}_1\text{ かつ }\lambda_1=1\\
\boldsymbol{v}_2&=\boldsymbol{w}_2&=(1,1)&\text{ はゼロに射影されます: }&T(\boldsymbol{v}_2)=0\text{ かつ }\lambda_2=0 \end{matrix}
\begin{matrix} \text{固有ベクトル基底}\\ \text{対角行列} \end{matrix} \text{ 新しい行列は } \begin{bmatrix}1&0\\0&1 \end{bmatrix}\begin{bmatrix}\lambda_1&0\\0&\lambda_2 \end{bmatrix}=\Lambda \;\;\; (6)
固有ベクトルは完璧な基底ベクトルです。それらは固有値行列 $\Lambda$ を生成します。
入力基底 = 出力基底の他の選択はどうでしょうか?それらの基底ベクトルを $B$ の列に入れます。上で見たように、基底の変換行列(標準基底と新しい基底の間)は $B_{\text{in}}=B$ と $B_{\text{out}}=B^{-1}$ です。$T$ の新しい行列は $A$ に似ています:
$A_{\text{new}}=B^{-1}AB$ 新しい基底の $\boldsymbol{b}$ での $A$ は標準基底の $A$ に似ています:
A_{\boldsymbol{b}'s\text{ to }\boldsymbol{b}'s} = B_{\text{standard to }\boldsymbol{b}'s}^{-1} \;\; A_{\text{standard}} \;\; B_{\boldsymbol{b}'s\text{ to standard}}\;\;(7)
変換 $ITI$ と行列 $B^{-1}AB$ に対する乗算ルールを使用しました。
最後に、異なる空間 $V$ と $W$、および異なる基底 $\boldsymbol{v}$ と $\boldsymbol{w}$ を可能にします。$T$ が分かっていて基底を選択すると、行列 $A$ を得ます。$A$ はおそらく対称でも正方形でもありません。しかし、対角行列を生成する $\boldsymbol{v}$ と $\boldsymbol{w}$ を常に選択することができます。これは分解 $A=U\Sigma V^T$ の中で特異値行列 $\Sigma=\text{diag} (\sigma_1,\cdots,\sigma_r)$ になります。
特異ベクトル
\text{特異値分解 (SVD) は} U^{-1}AV=\Sigma \text{ を示します。}
\text{右特異ベクトル}\boldsymbol{v}_1,\cdots,\boldsymbol{v}_n \text{ は入力基底になります。}
\text{左特異ベクトル}\boldsymbol{u}_1,\cdots,\boldsymbol{u}_m\text{ は出力基底になります。}
\text{行列乗算のルールに従い、これらの新しい基底での同じ変換のための行列は}
B_{\text{out}}^{-1}AB_{\text{in}}=U^{-1}AV=\Sigma\text{ です。対角です!}
$\Sigma$ が $A$ に「似ている」とは言えません。私たちは今、入力と出力の2つの基底で作業しています。しかし、それらは直交基底であり、ベクトルの長さを保持します。David Voganの良い提案に従って、私たちは"""$\Sigma$ は $A$ に「相似」"""だといいます。
\text{定義 }\;\;\; C=Q_1^{-1}AQ_2 \text{ は } A \text{ に相似である、もし } Q_1 \text{ と } Q_2 \text{ が直交している場合。}
例8
変換 $T=\frac{d}{dx}$ に対する行列 $A$ を構築するために、入力基底として $1, x, x^2, x^3$、出力基底として $1, x, x^2$ を選びました。行列 $A$ はシンプルでしたが、残念ながら対角行列ではありません。しかし、各基底を逆の順序で並べ変えます。
今、入力基底は $x^3, x^2, x, 1$ であり、出力基底は $x^2, x, 1$ です。基底の変更行列 $B_{\text{in}}$ と $B_{\text{out}}$ は置換です。新しい基底での $T(u)=du/dx$ のための行列は、$\sigma = 3, 2, 1$ を持つ対角特異値行列 $B_{\text{out}}^{-1}AB_{\text{in}}=\Sigma$ です:
B_{\text{out}}^{-1}AB_{\text{in}} = \begin{bmatrix} \;&\;&1\\ \;& 1&\; \\ 1 & \; & \; \end{bmatrix}\begin{bmatrix} 0 &1&1&0\\ 0&0&2&0 \\ 0 & 0 & 0&3 \end{bmatrix}\begin{bmatrix} \;&\;&\;&1 \\ \;&\;&1& \; \\ \;&1 & \; & \;\\1&\;&\;&\; \end{bmatrix}=\begin{bmatrix} 3&0&0&0\\ 0&2&0&0 \\ 0 & 0 & 1&0 \end{bmatrix}
"""
Pythonによる理解
例4のコード
import numpy as np
# 入力基底ベクトルと出力基底ベクトルを定義
input_basis = [[1,0,0,0],[0,1,0,0],[0,0,1,0],[0,0,0,1]]
output_basis = [[1,0,0],[0,1,0],[0,0,1]]
# 導関数を取る関数Tを定義
def T(v):
if v == input_basis[0]:
return 0 + 0 + 0 + 0 # d(1)/dx = 0
elif v == input_basis[1]:
return 1*output_basis[0]+0*output_basis[1]+0*output_basis[2] # d(x)/dx = 1
elif v == input_basis[2]:
return 0*output_basis[0]+2*output_basis[1]+0*output_basis[2] # d(x^2)/dx = 2x
elif v == input_basis[3]:
return 0*output_basis[0]+0*output_basis[1]+3*output_basis[2] # d(x^3)/dx = 3x^2
# Tの出力を利用して行列Aを構築する関数
def construct_A(input_basis, output_basis):
A = np.zeros((len(output_basis), len(input_basis))) # 出力基底x入力基底のサイズで0行列を初期化
# 各入力基底ベクトルに対する導関数の計算
for i, v in enumerate(input_basis):
derivative = T(v)
# 導関数の結果に基づいて、行列Aの列を更新
if derivative == 0:
continue # 導関数が0の場合は、既に初期化されている0を使用
elif derivative == output_basis[0]:
A[0, i] = 1 # xの導関数は1、最初の行の対応する列に1を設定
elif derivative == 2*output_basis[1]:
A[1, i] = 2 # x^2の導関数は2x、2番目の行の対応する列に2を設定
elif derivative == 3*output_basis[2]:
A[2, i] = 3 # x^3の導関数は3x^2、3番目の行の対応する列に3を設定
return A
# 行列Aを構築
A_constructed = construct_A(input_basis, output_basis)
A_constructed
# 成分による計算
A=A_constructed
A@[1,2,3,4]
行列の構成の概念と実用例
連立方程式
注文書から請求書を作成するプロセスは連立方程式に関連付けた行列の構成に例えることができます。この比喩では、注文書に記載された商品やサービス(入力ベクトル)に対して、それぞれの価格(行列 $A$ の係数)を掛け合わせ、最終的に請求総額(出力ベクトル)を求めるプロセスを想像できます。
連立方程式の行列表現
連立方程式のシステムは、一般に行列の形式で表されます。これは $Ax = b$ の形で、ここで $A$ は係数行列、$x$ は未知数のベクトル、$b$ は結果のベクトルです。この文脈では、$A$ は商品やサービスの価格を表し、$x$ は注文された各商品やサービスの数量、$b$ は各項目の合計金額に相当します。
注文書から請求書へ
-
注文書:顧客からの注文書は、特定の商品やサービスの要求リストと見なすことができます。これは未知数ベクトル $x$ に相当し、注文された各アイテムの数量を表します。
-
価格表(行列 $A$):商品やサービスの価格表は、連立方程式の係数行列 $A$ に類似しています。この行列は、各商品やサービスに適用される単価を含んでおり、注文書に基づいて請求書の総額を計算するために使用されます。
-
請求書($b$):価格表($A$)と注文書($x$)を元に計算された請求書の総額は、結果のベクトル $b$ に相当します。これは、注文された各商品やサービスの数量に対応する価格を掛け合わせ、すべてのアイテムの合計金額を求めるプロセスです。
このプロセスは、連立方程式を解く行為と本質的に同じです。行列 $A$(価格表)を未知数ベクトル $x$(注文書)に適用することで、結果のベクトル $b$(請求書の合計)を得ることができます。この比喩を通じて、の行列の構成がどのように現実世界の問題、特に連立方程式を解くために使用されるかを視覚的に理解することができます。
連立方程式と行列の構成
「行列の構成」とは、特定の数学的または物理的な問題を解決するために行列とベクトルの演算を用いるプロセスを指します。したがって、連立方程式の解法は、この定義に完全に適合します。
連立方程式 $Ax = b$ において、係数行列 $A$、未知ベクトル $x$、結果ベクトル $b$ は、すべて行列演算で処理されます。この問題を解くためには、以下のような行列操作が行われます:
-
逆行列の計算: $A$ の逆行列 $A^{-1}$ を計算し、それを $b$ に乗じることで $x$ を求める($x = A^{-1}b$)。
-
LU分解、コレスキー分解、QR分解など: $A$ をより扱いやすい形式に分解し、その分解を利用して効率的に $x$ を求める。
-
反復法: $A$ が大規模で疎な場合などに、ガウス・ザイデル法や共役勾配法などの反復法を用いて $x$ を近似的に求める。
これらのプロセスはすべて、行列とベクトルの演算に基づいています。また、これらの操作を行う際には、行列 $A$ の構成(例えば、その分解や逆行列の計算など)が中心的な役割を果たします。
行列の構成と問題解決
連立方程式の解法において、行列 $A$ の構成は、問題を解くための「道具」として機能します。このプロセスは、与えられた問題(連立方程式)を、行列演算によって解ける形($Ax = b$)に構成し直し、その後の操作(逆行列の計算、行列の分解など)を通じて解を求めるというプロセスです。
したがって、連立方程式の解法は、行列 $A$ を用いて入力 $x$ を求めるという観点から、行列の構成の枠組みに非常に適しています。このアプローチは、行列とベクトルの演算を用いて、数学的な問題を効率的に解くための強力な手段を提供します。
線形回帰
最小二乗法を用いた線形回帰は、の行列の構成の文脈で考えると、射影の概念を直接使用しているとみなすことができます。線形回帰問題では、与えられたデータセットに最もよく適合する線形モデルを見つけることが目的です。このプロセスは、データ点を線形モデルによって張られる空間へ射影する操作と見なすことができ、この射影が最小二乗誤差を最小化します。
線形回帰と射影
線形回帰モデルは、形式的には $Y = X\beta + \epsilon$ と表され、ここで $Y$ は応答変数のベクトル、$X$ は説明変数の行列(デザイン行列)、$\beta$ は未知の係数ベクトル、$\epsilon$ は誤差項です。最小二乗法の目的は、誤差 $\epsilon$ の二乗和を最小化する係数ベクトル $\beta$ を見つけることです。
この問題は、$X$ によって張られる列空間への $Y$ の射影を見つけることと同等です。この射影は、$X$ の列空間内で $Y$ に最も近い点として解釈されます。この最適な射影点は、$X$ の列によって張られる空間上の $Y$ の直交射影に他なりません。
行列の構成と最小二乗法
この文脈での行列の構成は、$X$ の列が基底を形成し、最適な係数ベクトル $\beta$ を見つけることによって、この基底に関して $Y$ の最適な表現を求める過程と解釈できます。したがって、最小二乗法による線形回帰は、特定の基底に関して最適な射影を見つけるという「行列の構成」の概念に直接関連しています。
最小二乗解は、行列 $X$ の擬似逆行列 $X^+$ を使用して $\beta = X^+Y$ と計算されます。この擬似逆行列は、$X$ の列によって張られる空間への射影を実行する行列として機能します。
主成分分析
時系列データからノイズを除去するために主成分分析(PCA)の使用は、行列の構成の文脈で考えることができます。主成分分析は、データセット内の変動を最もよく説明する方向を見つけ出し、それらの方向(主成分)に基づいてデータを再構成する手法です。このプロセスでは、データの次元削減が行われ、ノイズが低減されることが期待されます。
主成分分析と行列の構成
主成分分析では、データセットを表す行列 $X$ から、共分散行列または相関行列が計算されます。この共分散行列の固有ベクトルと固有値を求めることにより、データの変動を最大にする方向(主成分)を特定します。この固有ベクトル(主成分)は、新しい基底を形成し、データをこの基底に関して再構成することで、ノイズを減らすことができます。
このプロセスは、データセット $X$ を、主成分によって張られる低次元空間に射影する操作と見なすことができます。この射影は、データセットの本質的な特徴を保持しつつ、ノイズや冗長な情報を取り除くことを目指します。
「行列の構成」の文脈でのPCA
行列の構成の文脈では、主成分分析を用いたノイズ除去は、特定の基底(主成分)に関してデータを最適に表現する過程とみなせます。ここでの「基底の選択」は、データの変動を最大限に捉える方向を特定することに相当し、これらの方向(主成分)にデータを射影することで、よりクリーンなデータ表現を得ることができます。
この意味で、PCAを用いた時系列データのノイズ除去は、データの表現を最適化するための基底の選択と行列の構成の概念に直接関連しています。データの再構成は、選択された基底(主成分)に関して行われ、元のデータセットよりもノイズが少ない、洗練された表現を提供します。
Python コード
2つの周期性をもつ特徴量と20の観測値からなるデータにノイズを載せます。このノイズを主成分分析で除去する過程を考えます。
特徴量:標準基底
次に主成分分析の共分散行列をつくるために、特徴量を中心化します。
標準基底->中心化基底
つぎに中心化基底の共分散行列を用いて、固有値、固有ベクトルの算出
主成分にデータを射影します。
中心化基底->固有ベクトル基底
projected_data = np.dot(data_centered, sorted_eigenvectors)
射影されたデータを標準基底に戻す(逆変換)
reconstructed_data = np.dot(projected_data, sorted_eigenvectors.T) + np.mean(data_noisy, axis=0)
特徴量の理論データであるdataとそれにノイズを載せたデータdata_noisyの平均二乗誤差とdataとreconstructed_dataの平均二乗誤差を比べると主成分分析を用いたノイズ除去の効果が見れます。
import numpy as np
import matplotlib.pyplot as plt
from sklearn.metrics import mean_squared_error
# データの設定
n_features = 2 # 特徴量の数
n_samples = 20 # 観測値の数
# 時間軸の生成
t = np.linspace(0, 1, n_samples)
# 理論データの生成
data = np.zeros((n_samples, n_features))
data_noisy = np.zeros((n_samples, n_features))
noise = np.random.normal(0, 2, data.shape) # ノイズを生成;noiseの大きさが重要
for i in range(n_features):
frequency = i + 1 # 周期(特徴量ごとに異なる)
data[:, i] = np.sin(2 * np.pi * frequency * t)
# dataを特徴量の線形結合とします。
weight=np.random.rand(n_features,n_features)
data=data.dot(weight)
# 個別のノイズを加える
data_noisy = data + noise # ノイズを加えたデータ
# データの可視化
print('Synthetic Time Series Data with Different Periodic Components and Noise')
for i in range(n_features):
plt.figure(figsize=(3, 2))
plt.plot(t, data[:, i], label=f'Data {i+1}')
plt.plot(t, data_noisy[:, i], label=f'Data+noise {i+1}')
plt.xlabel('Time')
plt.ylabel('Value')
plt.legend( loc='upper left', bbox_to_anchor=(1, 1))
plt.show()
print('mse ',mean_squared_error(data[:,i], data_noisy[:,i]))
# numpyのみを使用して主成分分析を実装し、データのノイズを除去します。
# 1. データを平均0に中心化
data_centered = data_noisy - np.mean(data_noisy, axis=0)
# 2. 共分散行列を計算
cov_matrix = np.cov(data_centered, rowvar=False)
# 3. 共分散行列の固有値と固有ベクトルを計算
eigenvalues, eigenvectors = np.linalg.eig(cov_matrix)
# 4. 固有ベクトルを固有値の降順でソートし、基底を変換
# 固有値の大きい順にインデックスを取得
sorted_indices = np.argsort(eigenvalues)[::-1]
sorted_eigenvectors = eigenvectors[:, sorted_indices]
# 5. 主成分にデータを射影
# ここではall-1の主成分を使用
projected_data = np.dot(data_centered, sorted_eigenvectors)
n_components =n_features-1 # 使用する主成分の数
# 6. 射影されたデータを元の空間に戻す(逆変換)
reconstructed_data = projected_data[:, :n_components].dot(sorted_eigenvectors[:, :n_components].T) + np.mean(data_noisy, axis=0)
# 理論データとノイズ除去後のデータをプロットして比較
print('Theoretical vs. Denoised Data After PCA')
for i in range(n_features):
plt.figure(figsize=(3, 2))
plt.plot(t, data[:, i], label=f'Theoretical Feature {i+1}', linestyle='--')
plt.plot(t, reconstructed_data[:, i], label=f'Denoised Feature {i+1}')
plt.xlabel('Time')
plt.ylabel('Value')
plt.legend( loc='upper left', bbox_to_anchor=(1, 1))
plt.show()
print('mse ',mean_squared_error(data[:,i], reconstructed_data[:,i]))
カルマンフィルター
カルマンフィルターは、線形動的システムの状態を推定するためのアルゴリズムであり、ノイズを含む観測データからシステムの状態を最適に推定します。このプロセスは、状態推定値の更新と修正を行うために一連の行列演算を使用します。
カルマンフィルターと行列の構成
カルマンフィルターの基本的な構成要素は次の通りです:
-
状態遷移行列 $A$: システムの時刻 $t$ から時刻 $t+1$ への状態遷移を記述します。この行列は、システムのダイナミクスをモデル化し、現在の状態から次の状態への進化を予測します。
-
観測行列 $H$: システムの内部状態と観測データとの関係を記述します。この行列は、状態ベクトルから観測ベクトルへのマッピングを提供します。
-
プロセスノイズと観測ノイズの共分散行列 $Q$ と $R$: システムの不確実性と観測データの不確実性をそれぞれモデル化します。
カルマンフィルターのプロセスは、基本的に次のステップで構成されます:
-
予測ステップ:状態遷移行列 $A$ を使用して、前の状態推定から次の状態推定への予測を行います。
-
更新ステップ:観測行列 $H$ を使用して、新たな観測データを取り入れ、予測された状態推定を修正します。
このプロセスは、システムの状態空間を表すために選択された基底(状態ベクトルの成分)に関して、観測データを最適に射影することで、システムの真の状態に最も近い推定値を計算します。この意味で、カルマンフィルターは行列の構成の概念を用いて、動的システムの状態推定問題を解決する方法と見なすことができます。
カルマンフィルターは、行列とその演算を用いて状態空間をナビゲートする方法の一例であり、線形代数の概念を応用して複雑なシステムの挙動を理解し制御する強力なフレームワークを提供します。
状態空間方程式と異常検知
状態空間モデルは、システムのダイナミクスを数学的に記述するために行列を使用し、システムの内部状態と観測データの関係をモデル化します。このアプローチは、システムの挙動を理解し、予測し、異常な動きを検出するために用いられます。
状態空間方程式と異常検知
状態空間方程式は一般に以下の2つの方程式で構成されます:
-
状態方程式:システムの時間による進化を記述し、内部状態のダイナミクスをモデル化します。
$ x_{t+1} = Ax_t + Bu_t + w_t $
ここで、$x_t$ は時刻 $t$ における状態ベクトル、$A$ は状態遷移行列、$B$ は制御入力行列、$u_t$ は制御入力、$w_t$ はプロセスノイズを表します。 -
観測方程式:システムの状態と観測データとの関係を定義します。
$ y_t = Hx_t + v_t $
ここで、$y_t$ は時刻 $t$ における観測ベクトル、$H$ は観測行列、$v_t$ は観測ノイズです。
異常検知において、状態空間モデルはシステムの正常な動作を記述するために使用され、観測データがモデルから大きく逸脱する場合に異常が検出されます。この逸脱は、観測データとモデルに基づく予測との間の残差を分析することで検出されます。
行列の構成の文脈での異常検知
行列の構成では、特定の基底に関して最適な表現を見つけることが重視されます。状態空間モデルにおいては、$A$、$B$、$H$ などの行列がシステムのダイナミクスと観測データの関係を定義する基底として機能します。異常検知においては、これらの基底に基づいて構築されたモデルを用いてデータを解析し、異常な動きを識別することが目的です。
したがって、状態空間方程式を用いた異常検知は、システムのダイナミクスと観測データの関係を最適に表現し、その表現を用いてデータから異常を検出するという、行列の構成の枠組みにうまく収まります。このアプローチは、行列とその演算を用いてシステムを理解し、異常な挙動を検出するための有効な手段を提供します。
時系列解析の季節性
時系列解析の周波数領域での季節性解析、特にフーリエ変換やウェーブレット変換を使用して季節性を調べる手法は、行列の構成の枠組みに適合します。これらの変換は、時系列データを周波数領域に変換し、データ内の周期的なパターンや季節性を明らかにすることを目的としています。
時系列解析と行列の構成
フーリエ変換やウェーブレット変換を行う際、基底関数(例えば、正弦波やウェーブレット)を用いて時系列データを表現します。このプロセスは、データを新しい「基底」に関して最適な表現を見つけるという意味で、行列の構成の一形態です。具体的には、時系列データを異なる周波数成分に分解し、それぞれの成分の強度や位相を分析することで、季節性やトレンドを把握します。
周波数領域での季節性解析
周波数領域での季節性解析では、時系列データのスペクトル(周波数成分の分布)を調べ、特定の周期性を持つ成分を識別します。例えば、フーリエ変換を用いると、データ内の定期的なパターンが特定の周波数に対応する成分として現れます。これにより、データの季節性を定量化し、理解することができます。
行列の構成の文脈での適合性
この分析プロセスは、特定の「基底」に対してデータを再構成し、その再構成を通じてデータの性質を理解するという、行列の構成の概念に直接関連しています。周波数領域の分析は、時系列データを構成する異なる「成分」や「要素」を明らかにし、それらがどのように全体の挙動に寄与しているかを示すものです。
ディープラーニング
ディープラーニングは、行列の構成の概念を広い意味で含んでいますが、その適用はより複雑で直接的ではない方法で行われます。ディープラーニングは、多層ニューラルネットワークを通じて複雑な非線形変換を実行することにより、データから高度なパターンを学習するプロセスです。このプロセスでは、重み行列と活性化関数を用いて入力データを変換し、目的の出力にマッピングします。
ディープラーニングと行列の構成
ディープラーニングモデルの各層は、基本的には入力ベクトルに対して行列変換(重み行列による線形変換)を適用し、その後に非線形活性化関数を適用するプロセスです。この観点から、ディープラーニングは複数の行列変換を組み合わせて使用し、それぞれの層で特定の「基底変換」を実行していると解釈できます。しかし、ディープラーニングでは、これらの変換が単純な基底の選択や再構成以上のものを含んでいます。
行列操作の重要性
ディープラーニングにおける行列操作は、ネットワークの前方伝播(入力から出力への計算)および後方伝播(勾配の計算と重みの更新)の両方において中心的な役割を果たします。これらの操作は、ネットワークがデータから複雑な関係を効率的に学習することを可能にします。
行列の構成の概念との関連
ディープラーニングにおける「行列の構成」という観点は、重み行列を通じてデータを新しい表現空間にマッピングするというプロセスに関連しています。これは、行列の構成の概念に類似しており、特定の基底(この場合は重み行列によって定義される)に関してデータを最適に表現することを目指しています。ただし、ディープラーニングの場合、この「基底」は学習過程を通じて動的に調整され、データの非線形性や複雑さを捉えるために高度な非線形変換を組み込んでいます。
Appendix
The next pages assign a matrix $A$ to every linear transformation $T$. For ordinary column vectors, the input $v$ is in $V=R^n$ and the output $T(v)$ is in $W=R^m$. The matrix for this transformation will be $m$ by $n$. Our choice of bases in $V$ and $W$ will decide $A$.
The standard basis vectors for $R^n$ and $R^m$ are the columns of $I$. That choice leads to a standard matrix. Then $T(v)=Av$ in the normal way. But these spaces also have other bases, so the same transformation $T$ is represented by other matrices. A main theme of linear algebra is to choose the bases that give the best matrix (a diagonal matrix) for $T$.
All vector spaces $V$ and $W$ have bases. Each choice of those bases leads to a matrix for $T$. When the input basis is different from the output basis, the matrix for $T(v)$ will no be the identity $I$ It will be the "change of basis matrix". Here is the key idea:
Suppose we know outputs $T(v)$ for the input basis vectors $v_1$ to $v_n$. Columns 1 to $n$ of the matrix will contain those outputs $T(v_1)$ to $T(v_n)$. $A$ times $c=$ matrix times vector = combination of those $n$ columns.
$Ac$ gives the correct combination $c_1T(v_1)+\cdots+c_nT(v_n)=T(v)$.
Reason Every $v$ is a unique combination $c_1v_1+\cdots+c_nv_b$ of the basis vectors $v_j$.
Since $T$ is a linear transformation (here is the moment for linearity), $T(v)$ must be
the same combination $c_1T(v_1)+\cdots+c_nT(v_n)$ of the outputs $T(v_j)$ in the columns.
Our first example gives the matrix $A$ for the standard basis vectors in $R^2$ and $R^3$.
The two columns of $A$ are the outputs from $v_1=(1,0)$ and $v_2=(0,1)$
Example1 Suppose $T$ transforms $v_1=(1,0) to $T(v_1)=(2,3,4)$. Suppose the second basis vector $v_2=(5,5,5)$. If $T$ is linear from $R^2$ to $R^3$ then its "standard matrix" is 3by2. Those outputs $T(v_1)$ and $T(v_2)$ go into the columns of $A$:
A = \begin{bmatrix} 2 & 5 \\ 3 & 5 \\ 4 & 5 \end{bmatrix} c_1=1 \text{and } c_2 =1 \text{give } T(v_1+v_2)=\begin{bmatrix}
2 & 5 \\ 3 & 5 \\ 4 & 5 \end{bmatrix}\begin{bmatrix}1 \\ 1 \end{bmatrix}=\begin{bmatrix}7 \\ 8 \\ 9 \end{bmatrix}
Example2: Change of Basis: Matrix B
Suppose the input space $V=R$ is also the output space $W=R^2$
Suppose that $T(\boldsymbol{v})=\boldsymbol{v}$ is the identity transformation. You might expect its matrix to be $I$, but that only happens when the input basis is the same as the output basis.
I will choose different bases to see how the matnx is constructed.
For this special case $T(\boldsymbol{v})=\boldsymbol{v}$, I will call the matrix $B$ instead of $A$. We are just Changing basis from the $\boldsymbol{v}'s$ to the $\boldsymbol{w}'s$. Each is a combination of $\boldsymbol{w}_1$ and $\boldsymbol{w}_2$.
\begin{matrix} Input\\basis \end{matrix} \begin{bmatrix} \boldsymbol{v}_1 \boldsymbol{v}_2 \end{bmatrix}=\begin{bmatrix} 3&6\\3&8 \end{bmatrix}\begin{matrix} Output\\basis \end{matrix} \begin{bmatrix} \boldsymbol{w}_1 \boldsymbol{w}_2 \end{bmatrix}=\begin{bmatrix} 3&0\\1&2 \end{bmatrix}\begin{matrix} Change\\of basis \end{matrix} \begin{bmatrix} \boldsymbol{v}_1 =1 \boldsymbol{w}_1+1\boldsymbol{w}_2\\\boldsymbol{v}_2=2\boldsymbol{w}_1+3\boldsymbol{w}_3 \end{bmatrix}
Please notice! I wrote the input basis $\boldsymbol{v}_1,\boldsymbol{v}_2$ in terms of the output basis $\boldsymbol{w}_1,\boldsymbol{w}_2$. That is because of our key rule. We apply the identity transformation $T$ to each input basis vector: $T(\boldsymbol{v}_1)=\boldsymbol{v}_1$ and $T(\boldsymbol{v}_2)=\boldsymbol{v}_2$. Then we write those outputs $\boldsymbol{v}_1$ and $\boldsymbol{v}_2$ in the output basis $\boldsymbol{w}_1$ and $\boldsymbol{w}_2$. Those bold numbers 1,1 and 2,3 tell us column 1 and column 2 of the matrix B (the change of basis matrix): $WB=V$ so $B=W^{-1}V$.
\begin{matrix} \text{Matrix B for }\\ \text{change of basis }\end{matrix} \begin{bmatrix} \\ \boldsymbol{w}_1 \boldsymbol{w}_2\\ \;\end{bmatrix}=\begin{bmatrix} \\B\\ \; \end{bmatrix}\begin{bmatrix} \boldsymbol{v}_1 \boldsymbol{v}_2 \end{bmatrix}\text{ is } \begin{bmatrix} 3&0\\1&2 \end{bmatrix}\begin{bmatrix} \mathbf{1}&\mathbf{2}\\ \mathbf{1}&\mathbf{3} \end{bmatrix}= \begin{bmatrix} 3&6\\3&8 \end{bmatrix} \;\;\;(1)
When the input basis is in the columns of a matrix $V$,and the output basis is in the columns of $W$ the change of basis matrix for $T=I$ is $B=W^{-1}V$.
The key I see a clear way to understand that rule $B=W^{-1}V$. Suppose the same vector $\boldsymbol{u}$ is written in the input basis of $\boldsymbol{v}'s$ and the output basis of $\boldsymbol{w}'s$. I will do that three ways:
\begin{matrix} \boldsymbol{u}=c_1\boldsymbol{v}_1+\cdots+c_n\boldsymbol{v}_n\\ \boldsymbol{u}=d_1\boldsymbol{w}_1+\cdots+d_n\boldsymbol{w}_n \end{matrix}\text{ is } \begin{bmatrix} \\ \boldsymbol{v}_1 \cdots \boldsymbol{v}_n\\ \;\end{bmatrix}\begin{bmatrix} c_1\\ \vdots \\ c_n \end{bmatrix}=\begin{bmatrix} \boldsymbol{w}_1 \cdots \boldsymbol{w}_n \end{bmatrix}\begin{bmatrix} d_1\\ \vdots \\d_n \end{bmatrix} \text{ and }V\boldsymbol{c}=W\boldsymbol{d}
The coefficients $\boldsymbol{d}$ in the new basis of $\boldsymbol{w}$'s are $\boldsymbol{d}=W^{-1}V\boldsymbol{c}$. Then $B$ is $W^{-1}V ;;;(2)$
This formula $B=W^{-1}V$ produces one of the world's greatest mysteries: When the standard basis $V=I$
Is changed to a different basis $W$, the change of basis matrix is not $W$ but $B=W^{-1}$. Larger basis vectors have smaller coefficients!
\begin{bmatrix} x\\y \end{bmatrix}
in the standard basis has coefficients
\begin{bmatrix} \boldsymbol{w}_1 &\boldsymbol{w}_2 \end{bmatrix}^{-1}\begin{bmatrix} x\\y \end{bmatrix}
in the $\boldsymbol{w}_1,\boldsymbol{w}_2$ basis.
Matrix Products AB Match Transformations TS
We have come to some thing important —the real reason for the rule to multiply matrices. At last we discover why! Two linear transformations $T$ and $S$ are represented by two matrices $A$ and $B$. Now compare $TS$ with the multiplication $AB$:
When we apply the transformation $T$ to the output from $S$, we get $TS$ by this rule:$(TS)(\boldsymbol{u})$ is defined to be $T(S(\boldsymbol{u}))$. The output $S(\boldsymbol{u})$ becomes the input to $T$.
When we apply the matrix $A$ to the output from $B$, we multiply $AB$ by this rule: $(AB)(\boldsymbol{x})$ is defined to be $A(B\boldsymbol{x})$. The output $BC$ becomes the input to $A$. Matrix multiplication gives the correct matrix $AB$ to represent $TS$.
The transformation $S$ is from a space $U$ to $V$、Its matrix $B$ uses a basis $\boldsymbol{u}_1,\cdots,\boldsymbol{u}_p$ for $U$ and a basis $\boldsymbol{v}_1,cdots, \boldsymbol{v}_n$ for $V$. That matrix is $n$ by $p$. The transformation $T$ is from $V$ to $W$ as before. Its matrix $A$ must use the same basis $\boldsymbol{v}_1,cdots, \boldsymbol{v}_n$ — this is the output space for S and the input space for $T$. Then the matrix $AB$ matches TS.
Multiplication The linear transformation $TS$ starts with any vector $\boldsymbol{u}$ in $U$, goes to $S(\boldsymbol{u})$ in $V$ and then to $T(S(\boldsymbol{u}))$ in $W$. The matrix $AB$ starts with any $\boldsymbol{x}$ in $R^P$, goes to $B\boldsymbol{x}$ in $R^n$ and then to $AB\boldsymbol{x}$ in $R^m$. The matrix $AB$ correctly represents $TS$:
$$TS:U→V→W AB:($m$ by $n)($n$ by $p$)=($m$ by $p$).
Product of transformations $TS$ matches product of matrices $AB$. An important case is when the spaces $U,V,W$ are the same and their base sare the same $\rightarrow$ square matrices.
Example 5 $S$ rotates the plane by $\theta$ and $T$ also rotates by$theta$. Then $TS$ rotates by $2\theta$. This transformation $T^2$ corresponds to the rotation matrix $A^2$ through $2\theta$:
T=S \;\; A=B \;\;\; T^2 = \text{ rotation by }2\theta \;\;\; A^2=\begin{bmatrix}\cos(2\theta) & -\sin(2\theta) \\ \sin(2\theta) & \cos(2\theta) \\ \end{bmatrix} \;\;\; (4)
A^2=\begin{bmatrix}\cos(\theta) & -\sin(\theta) \\ \sin(\theta) & \cos(\theta) \end{bmatrix}\begin{bmatrix}\cos(\theta) & -\sin(\theta) \\ \sin(\theta) & \cos(\theta) \end{bmatrix}=\begin{bmatrix}\cos^2(\theta)- \sin^2(\theta) & - 2\sin(\theta)\cos(\theta) \\ - 2\cos(\theta)\sin(\theta)& \cos^2(\theta)-\sin^2(\theta) & \end{bmatrix} \;\;\; (5)
Comparing (4) with (5) produces $\cos 2\theta = \cos^2 \theta -\sin^2 \theta$ and $\sin 2\theta=2\sin \theta \cos \theta$
Example 6
$S$ rotates by the angle $\theta$ and $T$ rotates byー$\theta$. Then $TS=I$ leads to $AB=I$. In this case $T(S(\boldsymbol{u}))$ is $\boldsymbol{u}$. We rotate forward and back. For the matrices to match, $Ab\boldsymbol{x}$ must be $\boldsymbol{x}$. The matrices with $\theta$ and $-\theta$ are inverses.
Choosing the Best Bases
Now comes the final step in this section of the book. Choose bases that diagonalize the
matrix. With the standard basis (the columns of $I$) our transformation $T$ produces some matrix $A$— probably not diagonal. That same $T $ is represented by different matrices when we choose different bases. The two great choices are eigenvectors and singular vectors:
Eigenvectors
If $T$ transforms $R^n$ to $R^n$, its matrix $A$ is square. If there are $n$ independent eigenvectors, choose those as the input and output basis. In this good basis, the matrix for $T$ is the diagonal eigenvalue matrix $A$.
Example 7
The projection matrix $T$ projects every $\boldsymbol{v}=(x,y)$ in $R^2$ onto the line $y=-x$. Using the standard basis, $\boldsymbol{v}_1=(0,1)$ projects to $T(\boldsymbol{v}_1)=\left(\frac{1}{2},-\frac{1}{2}\right)$. For $\boldsymbol{v}_2=(0,1)$ the projection is $T(\boldsymbol{v}_2)=(-\frac{1}{2},\frac{1}{2})$. Those are the columns of $A$:
\begin{matrix}\text{Projection matrix}\\\text{Standard bases}\\ \text{Not diagonal} \end{matrix} A=\begin{bmatrix} \frac{1}{2}&-\frac{1}{2}\\-\frac{1}{2}&\frac{1}{2}\end{bmatrix} \text{ has } A^T=A \text{ and } A^2=A.
Now comes the main point of eigenvectors. Make them the basis vectors! Diagonalize!
When the basis vectors are eigenvectors, the matrix becomes diagonal.
\begin{matrix}\boldsymbol{v}_1&=\boldsymbol{w}_1&=(1,ー1)&\text{ projects to itself:} &T(\boldsymbol{v}_1)=\boldsymbol{v}_1\text{ and }\lambda_1=1\\
\boldsymbol{v}_2&=\boldsymbol{w}_2&=(1,1)&\text{ projects to zero: }&T(\boldsymbol{v}_2)=0\text{ and }\lambda_2=0 \end{matrix}
\begin{matrix} \text{Eigenvector bases}\\ \text{Diagonal matrix} \end{matrix} \text{ The new matrix is } \begin{bmatrix}1&0\\0&1 \end{bmatrix}\begin{bmatrix}\lambda_1&0\\0&\lambda_2 \end{bmatrix}=\Lambda \;\;\; (6)
Eigenvectors are the perfect basis vectors. They produce the eigenvalue matrix $\Lambda$.
What about other choices of input basis =output basis? Put those basis vectors into the columns of $B$. We saw above that the change of basis matrlces (between standard basis and new basis)are $B_{in}=B$ and $B_{out}=B^{-1}$. The new matrix for $T$ is similar to $A$:
$A_{new}=B^{-1}AB$ In the new basis of b's is similar to A in the standard basis:$
A_{\text{b's to b's}} \;\; B_{\text{standard to b's}}^{-1} \;\; A_{standard} \;\; B_{b's\; to\;standard}\;\;(7)
I used the multiplication rule for the transformation $ITI$ and the matrices $B^{-1}AB$
Finally we allow different spaces $V$ and $W$, and different basis $\boldsymbol{v}$'s and $\boldsymbol{w}'s$. When we know $T$and we choose bases, we get a matrix $A$. Probably $A$ is not symmetric or even square. But we can always choose $\boldsymbol{v}$'s and $\boldsymbol{w}$'s that produce a diagonal matnx. This will be the singular value matrix $\Sigma=\text{diag} (\sigma_1,\cdots,\sigma_r$) in the decomposition $A=U\Sigma V^T$.
Singular vectors The SVD says that $U^{-1}AV=\Sigma$. The right singular vectors $\boldsymbol{v}_1,\cdots,\boldsymbol{v}_n$ will be the input basis. The left singular vectors $\boldsymbol{u}_1,\cdots.\boldsymbol{u}_m$
will be the output basis. By the rule for matrix multiplication, the matrix for the same transformation in these new bases is $B_{out}^{-1}AB_{in}=U^{-1}AV=\Sigma$. Diagonal!
I can't say that $\Sigma$ is "similar" to $A$. We are working now with two bases, input and output. But those are orthonormal bases and theypreserve the lengths of vectors. Following a good suggestion by David Vogan, I propose that we say: $\Sigma$ is "isometric” to $A$.
\text{Definition }\;\;\; C=Q_1^{-1}AQ_2 \text{ is isometric to } A \text{ if } Q_1 \text{ and } Q_2 \text{ are orthogonal}.
Example 8
To construct the matrix $A$ for the transformation $T=\frac{d}{dx}$, we chose the input basis $1,x,x^2,x^3$ and the output basis $1,x,x^2$. The matrix $A$ was simple but unfortunately it wasn't diagonal. But we can take each basis in the opposite order.
Now the input basis is $x^3,x^2,x,1$ and the output basis is $x^2,ェ,1$. The change of basis matrices $B_{in}$ and $B_{out}$ are permutations. The matrix for $T(u)=du/dx $ with the new bases is the diagonal singular value matrix $B_{out}^{-1}AB_{in}=\Sigma$ with $\sigma's=3,2,1$:
B_{out}^{-1}AB_{in} = \begin{bmatrix} \;&\;&1\\ \;& 1&\; \\ 1 & \; & \; \end{bmatrix}\begin{bmatrix} 0 &1&1&0\\ 0&0&2&0 \\ 0 & 0 & 0&3 \end{bmatrix}\begin{bmatrix} \;&\;&\;&1 \\ \;&\;&1& \; \\ \;&1 & \; & \;\\1&\;&\;&\; \end{bmatrix}=\begin{bmatrix} 3&0&0&0\\ 0&2&0&0 \\ 0 & 0 & 1&0 \end{bmatrix}
ーーーーーーーーーーーーーーーーーーーーーーーー
Python3ではじめるシステムトレード【第2版】環境構築と売買戦略
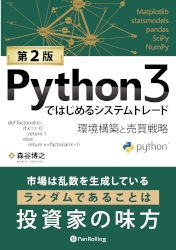
「画像をクリックしていただくとpanrollingのホームページから書籍を購入していただけます。
Python3ではじめるシステムトレード改訂版 アマゾンから購入いただけます。
24021223560240278460