##はじめに
準備編ではKaggleのコンペティション『Avito Demand Prediction Challenge』に参加するまでに必要な準備をしました。今回はデータをダウンロードするところから機械学習モデルを訓練して最終的に提出するところまでを実践編として書いていきたいと思います。
- データをダウンロードする
- tmux と Jupyter Notebookを立ち上げる
- データを観察する
- ターゲット値を観察する
- 欠損値を処理する
- 特徴量エンジニアリング
- モデルを訓練する
- 結果を提出する
##環境
Intel(R) Core(TM) i7-7700K CPU @ 4.20GHz 16GB
Radeon RX Vega 56 Air Boost 8GB
Ubuntu 16.04 LTS
##データをダウンロードする
参加するコンペティションのData
タブからデータをダウンロードします。基本的にダウンロードする場所はお好きな場所で構いませんが、input
ディレクトリをつくってその中にデータを置いておくと後々便利です。
- 新しいディレクトリ
~/Kaggle/Avito/input
を作る -
input
ディレクトリ内にデータをダウンロードする
##tmux と Jupyter Notebookを立ち上げる
まず作業用としてAvito
という名前でtmuxのセッションをつくります。
まだtmuxをインストールしていない方は準備編をご覧ください。
tmux new -s Avito
tmuxのセッションをつくると最初は自動でアタッチされます。
ここで任意ですが、先ほどデータをダウンロードしたディレクトリの一つ上~/Kaggle/Avito/
にコードを管理するディレクトリnotebooks
を作成します。
cd Kaggle/Avito/
mkdir notebooks
cd notebooks
次にJupyter Notebookを立ち上げます。
jupyter notebook
自動的にブラウザが立ち上がりますので New
> Python3
とクリックして新しくファイルを作成します。
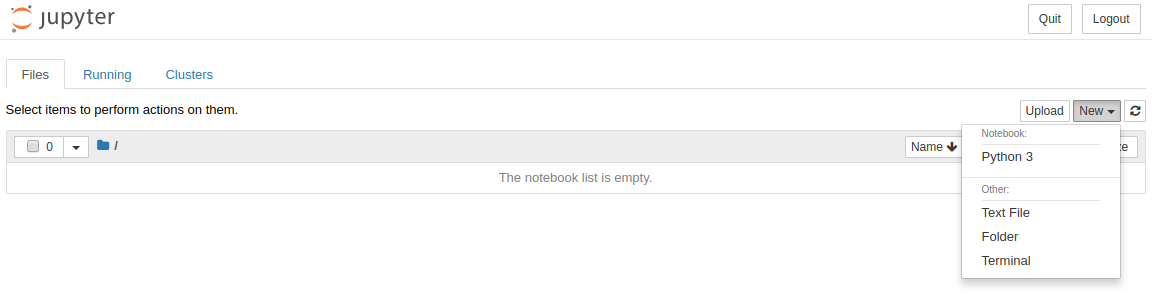
##データを観察する
まずコンペティションの概要から主催のAvitoとはロシアの大手広告サイトであり、主にオンライン広告を扱っているとわかります。そしてコンペティションとしては、表示されている広告がユーザーにとってどれほど需要があるかを予測するものとなっています。
Avito, Russia’s largest classified advertisements website, ... is challenging you to predict demand for an online advertisement based on its full description (title, description, images, etc.), its context (geographically where it was posted, similar ads already posted) and historical demand for similar ads in similar contexts. ...
概要がわかったらさっそくダウンロードしたデータを観察してみます。
まず必要なライブラリをインポートします。入力できたら Shift + Enter
で実行しましょう。
import numpy as np
import pandas as pd
import matplotlib.pyplot as plt
import warnings
warnings.filterwarnings('ignore')
%matplotlib inline
次にダウンロードしたファイル train.csv
と test.csv
からデータを読み込みます。
%%time
というJupyter Notebookのマジックコマンドを使うと処理にかかった時間を計測してくれるので便利です。
%%time
train = pd.read_csv('../input/train.csv', parse_dates=['activation_date'])
test = pd.read_csv('../input/test.csv', parse_dates=['activation_date'])
print('train shape: {}'.format(train.shape))
print('test shape: {}\n'.format(test.shape))
データが正常に読み込めたら、データのサイズ(行, 列)と処理にかかった時間が表示されます。
train shape: (1503424, 18)
test shape: (508438, 17)
CPU times: user 14 s, sys: 620 ms, total: 14.7 s
Wall time: 13.6 s
ここでhead()
でデータの先頭5行を表示して中身を確認してみます。item_id
のような主キーやcategory_name
のような広告のカテゴリを表す特徴量があります。ちなみにtail()
で下から5行を表示することもできますので興味があればやってみてください。
train.head()
またinfo()
を使えばデータの型や特徴量の数がわかります。
train.info()
このようにデータをざっくりと確認したら、次はそれぞれの特徴量を詳しく見ていきます。ここで便利なのが準備編でインストールしたpandas-profiling
というライブラリです。これを使うとたったひとつのコマンドで各特徴量のヒストグラムや欠損値の有無がわかるので探索的データ解析(Exploratory Data Analysis: EDA)を効率的に行うことができます。
import pandas_profiling
pandas_profiling.ProfileReport(train)
##ターゲット値を観察する
今回予測するターゲット値はdeal_probability
です。表示された広告から実際に商品が購入される可能性を表しています。この値は0から1の間を取り、値が高ければユーザにとって需要のある広告(商品)と判断し、低ければその逆となります。
deal_probability - The target variable. This is the likelihood that an ad actually sold something. ... this column's value can be any float from zero to one.
それではターゲット値を観察するためにいくつかのグラフを描いていきます。
まずはヒストグラムです。ヒストグラムを描くにはseaborn
ライブラリのdistplot
メソッドを使います。
import seaborn as sns
sns.distplot(train['deal_probability'])
plt.xlabel('deal_probability')
plt.ylabel('frequency')
次は散布図です。散布図を描くにはscatter
メソッドを使います。
# scatter(x軸, y軸)
plt.scatter(range(train.shape[0]), np.sort(train['deal_probability'].values))
plt.xlabel('rows of train data set')
plt.ylabel('deal_probability')
また、KernelにはEDAを詳細に行なっているものがたくさんあるので、それらを参考にしながらデータを深く理解できるように努めます。実際に私が参考にさせていただいたものを下記に載せておきます。
- Lathwalさん 「Avito EDA, FE, Time Series, DT Visualization」
- sbanさん 「In-Depth Analysis & Visualisations - Avito」
##欠損値を処理する
欠損値とは値が欠落しているデータのことを指します。基本的に欠損値がある状態では機械学習がうまく機能しないので、その部分を取り除いてしまうか何らかの値で補完してあげる必要があります。「機械学習のための欠損値処理まとめ」という記事に原因とその対処法が大変わかりやすくまとめられていますので参考にしてください。
今回はカテゴリ特徴量の欠損値はLabelEncoder
で処理し、連続特徴量に関しては平均値mean
で補完します。
それではさっそく欠損値の有無から確認していきましょう。ここで欠損値の総数と割合を返す関数search_missing_data
を定義します。
def search_missing_data(dataframe):
total = dataframe.isnull().sum()
percent = round(dataframe.isnull().sum() / dataframe.isnull().count() * 100, 2)
types = dataframe.dtypes
missing = pd.concat([total, percent, types], axis=1, keys=['Total', 'Percent', 'dtype'])
return missing
次のようにtrain
を引数として関数を呼び出します。
search_missing_data(train)
この表から7つの特徴量に欠損値があることがわかります。前述のとおり、カテゴリ特徴量である param_1
, param_2
, param_3
, image_top_1
は数値型にエンコードして欠損値を埋めます。せっかくなので欠損値のない他のカテゴリ特徴量に関しても一緒にエンコードしてしまいます。
from sklearn.preprocessing import LabelEncoder
# categorical variables
cat_vars = ['region','city','parent_category_name','category_name',
'param_1','param_2','param_3','user_type','image_top_1']
for col in cat_vars:
le = LabelEncoder()
le.fit(list(train[col].values.astype('str')) + list(test[col].values.astype('str')))
train[col] = le.transform(list(train[col].values.astype('str')))
test[col] = le.transform(list(test[col].values.astype('str')))
適切にエンコードできているか確かめるために再度search_missing_data()
を呼び出します。
エンコードする前はオブジェクト型だったカテゴリ特徴量がint型にエンコードされ、欠損値も埋まっていることがわかります。
次に広告の説明文description
の欠損値を埋めていきます。説明文がないことを表したいので空文字列で埋めます。
train['description'].fillna('', inplace=True)
最後に広告(商品)の価格price
の欠損値を平均値で埋めます。nanmean
を使えば欠損値を含まない平均値を計算できます。image
に関しては後ほど処理するのでここではそのままにしておきます。
train['price'].fillna(np.nanmean(train['price'].values), inplace=True)
##特徴量エンジニアリング
特徴量エンジニアリング(Feature Engineering: FE)とは機械学習モデルのパフォーマンスを向上させるために特徴量を増やすことです。しかし新しい特徴量を作るには専門的な知識や経験を要します。そこでとても有効なのがKaggleのKernelを読むことです。今回は下記のKernelを参考に特徴量エンジニアリングをしていきます。
- SRKさん 「Simple Exploration + Baseline Notebook - Avito」
- sbanさん 「Ideas for Image Features and Image Quality」
まず title
と description
に含まれる単語の数を数えてそれぞれlength
という特徴量として追加します。
train['title_length'] = train['title'].apply(lambda x: len(x.split()))
test['title_length'] = test['title'].apply(lambda x: len(x.split()))
train['description_length'] = train['description'].apply(lambda x: len(x.split()))
test['description_length'] = test['description'].apply(lambda x: len(x.split()))
次にテキストデータの特徴量エンジニアリングで一般的に用いられている tf-idf (term frequency-inverse document frequency) という手法を使います。 tf-idf とは特定の文章中にのみ頻出する単語はその文章の内容をよく表すキーワードであるはずだという考えに基づき、単語の重要度をベクトルで表現します。実装にはTfidfVectorizer
を使います。まずはtitle
に対して行なっていきます。
%%time
from sklearn.feature_extraction.text import TfidfVectorizer
tfidf = TfidfVectorizer(ngram_range=(1,3))
full_tfidf = tfidf.fit_transform(list(train['title'].values) + list(test['title'].values))
train_tfidf = tfidf.transform(list(train['title'].values))
test_tfidf = tfidf.transform(list(test['title'].values))
このままでは次元が大きすぎるのでTruncatedSVD
を使って次元削減します。今回は3次元まで圧縮します。
from sklearn.decomposition import TruncatedSVD
n_components = 3
svd = TruncatedSVD(n_components=n_components, algorithm='arpack')
svd.fit(full_tfidf)
train_svd = pd.DataFrame(svd.transform(train_tfidf))
test_svd = pd.DataFrame(svd.transform(test_tfidf))
train_svd.columns = ['title_tfidf_svd_'+str(i+1) for i in range(n_components)]
test_svd.columns = ['title_tfidf_svd_'+str(i+1) for i in range(n_components)]
train = pd.concat([train, train_svd], axis=1)
test = pd.concat([test, test_svd], axis=1)
del full_tfidf, train_tfidf, test_tfidf, train_svd, test_svd
同様にしてdescription
に対しても行います。
%%time
tfidf = TfidfVectorizer(ngram_range=(1,2), min_df=5, max_features=20000)
full_tfidf = tfidf.fit_transform(list(train['description'].values) + list(test['description'].values))
train_tfidf = tfidf.transform(list(train['description'].values))
test_tfidf = tfidf.transform(list(test['description'].values))
n_components = 3
svd = TruncatedSVD(n_components=n_components, algorithm='arpack')
svd.fit(full_tfidf)
train_svd = pd.DataFrame(svd.transform(train_tfidf))
test_svd = pd.DataFrame(svd.transform(test_tfidf))
train_svd.columns = ['description_tfidf_svd_'+str(i+1) for i in range(n_components)]
test_svd.columns = ['description_tfidf_svd_'+str(i+1) for i in range(n_components)]
train = pd.concat([train, train_svd], axis=1)
test = pd.concat([test, test_svd], axis=1)
del full_tfidf, train_tfidf, test_tfidf, train_svd, test_svd
最後にactivation_date
から曜日を表すweekday
という特徴量も追加してみます。
train['activation_weekday'] = train['activation_date'].dt.weekday
test['activation_weekday'] = test['activation_date'].dt.weekday
##モデルを訓練する
改めてデータを確認してから機械学習モデルに利用する特徴量を取捨選択していきます。
train.info()
item_id
は主キーとして提出用ファイルを作成する際に使うので変数test_id
に保存しておきます。
test_id = test['item_id'].values
item_id
や user_id
のようなユニークな値は特徴量としての情報を持たないため除外します。title
, description
, activation_date
は特徴量エンジニアリングを行い、それぞれ別の特徴量で表現したので除外します。また、欠損値を処理する工程で後回しにしたimage
は別に用意された画像ファイル群と合わせて処理する必要があったのですが、残念ながら時間との兼ね合いで処理しきれませんでした。そのため前述したsbanさんの「Ideas for Image Features and Image Quality」に解説を委ねたいと思います。ここでは除外します。
cols_to_drop = ['item_id', 'user_id', 'title', 'description', 'image', 'activation_date']
X_train = train.drop(cols_to_drop, axis=1)
X_test = test.drop(cols_to_drop, axis=1)
機械学習モデルを訓練するために訓練データとラベル(ターゲット値)を分割します。
y_train = train['deal_probability'].values
X_train = X_train.drop(['deal_probability'], axis=1)
print('X_train shape:', X_train.shape)
print('X_test shape:', X_test.shape)
ここまで来たらいよいよLightGBM
モデルを訓練します。パラメータの詳細やチューニングに関しては次の機会に記事にまとめられたらいいなと思っていますので、ここでは割愛させてください。コードを実行するとモデルの訓練がはじまります。
from sklearn.model_selection import train_test_split
import lightgbm as lgb
X_dev, X_val, y_dev, y_val = train_test_split(X_train, y_train, random_state=0)
# LightGBM dataset
lgb_train = lgb.Dataset(X_dev, y_dev)
lgb_valid = lgb.Dataset(X_val, y_val)
# LightGBM parameters
params = {
"objective": "regression",
"metric": "rmse",
"num_leaves": 30,
"learning_rate": 0.1,
"bagging_fraction": 0.7,
"feature_fraction": 0.7,
"bagging_frequency": 5,
"bagging_seed": 2018,
"verbosity": -1
}
# training
bst = lgb.train(params,
lgb_train,
num_boost_round=1000,
valid_sets=[lgb_valid],
early_stopping_rounds=100,
verbose_eval=20)
y_predict = bst.predict(X_test, num_iteration=bst.best_iteration)
##結果を提出する
機械学習モデルを訓練できたのでテストデータからdeal_probability
を予測します。予測結果が1より大きい場合は1に、0より小さい場合は0になるように調整して提出用のCSVファイルに出力します。
y_predict[y_predict > 1] = 1
y_predict[y_predict < 0] = 0
submit_df = pd.DataFrame({
'item_id': test_id,
'deal_probability': y_predict
})
submit_df.to_csv('my_submission.csv', index=False)
ファイルmy_submission.csv
は~/Kaggle/Avito/notebooks/
に作成されていますので、コンペティションのSubmissionから提出すれば完了です。お疲れ様でした!
##最後に
今回は与えられたデータからシンプルにLightGBMを使ってターゲット値を予測しました。欠損値を完全情報最尤推定法や多重代入法を用いて処理したり、LightGBM単体ではなくニューラルネットワークやその他の機械学習モデルと組み合わせたり、まだまだご紹介できなかった技術がたくさんあります。そして私自身も日々学んでいる最中で、これからも少しでも参考になる記事を書いていければと思っているので、もしよかったらフォローやいいねをしていただけると嬉しいです。最後になりましたが、今回の入賞チームの手法を載せて終わりたいと思います。お読みいただきありがとうございました。