#はじめに
前回の記事では、word2vecやfastTextに「𝑥 = ‘松本人志’ + ‘浜田雅功’ 」を解かせてみました。
ベクトル化した単語を加減算してみたり、よく似たベクトルの単語(=類似語)を抽出してみたり、単語のベクトルを比較して類似度を計算してみたり、思いつくまま学習済データでシミュレーション。
表示されるリストをみて「なるほどぉ」とうねっているだけであるが、これはこれでおもしろい。
ただ、折角ベクトル化しているのだから、単語と単語の距離感とかがバッと目に飛び込んでくるような可視化ができるともっといいだろうということで、ネットサーチすると、類似語の傾向(分布)を2次元で表現しておられるサイトがいくつかありました。
そのひとつが以下のサイトです。
いくつかの単語の類似語をクラスターとして、どんな分布になっているか散布図にプロットされていました。とてもいいです。
こちらのコードをベースに
- t-SNEでも二次元化してみよう
- できれば すべての類似語もグラフに表示させよう
ということで、実行してみたのがこの記事です。
いかんせん、まだまだコード構成力が足りませんので、無駄の多い冗長的なコード記述だと思いますが、なんとかいけてはいます。(こうすりゃいいのにという点があれば ぜひご教示ください)
##実行条件など
- Google colabで実行
#類似分布確認したい単語の設定
tags = [u'[Python]', u'[機械学習]', u'[自然言語]', u'[AI]'] #word2vec
#tags = ['Python', '機械学習', '自然言語', 'AI'] #fastText
#モデル構築
import numpy as np
# 単語の類似語とベクトルを返す
def similars(words, model):
vectors = []
cluster = []
label = []
for i in range(len(tags)):
tag = tags[i]
similar = model.similar_by_word(tag, topn=10)
for x in similar:
cluster.append(i)
label.append(tag)
vectors.append(model[x[0]])
return vectors, cluster, label
vectors, cluster, label = similars(tags, model)
# similar‗words list作成 (dict type)
similar_words = []
for tag in tags:
try:
similar_word = model.most_similar(tag)
for i in range(10):
similar = {}
similar['tag'] = tag
similar['similar_tag'] = similar_word[i][0]
similar['score'] = similar_word[i][1]
similar_words.append(similar)
except:
pass
#similar_wordsデータフレーム化&表示
df_similar = pd.DataFrame.from_dict(similar_words)
df_similar
可視化モデル構築#可視化モジュール(t-SNEはコメントアウト:適用する場合は#設定をPCAと変更) from sklearn.decomposition import PCA #from sklearn.manifold import TSNE import matplotlib.pyplot as plt import japanize_matplotlib def draw_scatter_plot(vectors, words, cluster): colors = ["b", "g", "r", "c", "m", "y", "k"] # PCAで次元圧縮 pca = PCA(n_components=2) coords = pca.fit_transform(vectors) # t-SNEで次元圧縮 #tsne = TSNE(n_components=2, random_state=0) #np.set_printoptions(suppress=True) #coords = tsne.fit_transform(vectors) # matplotlibによる可視化 plt.rcParams["font.size"] = 12 fig, ax = plt.subplots(figsize=(10,10)) x = [v[0] for v in coords] y = [v[1] for v in coords] for i in range(len(tags)): xx = [] yy = [] for c, a, b in zip(cluster, x, y): if i == c: xx.append(a) yy.append(b) ax.scatter(xx, yy, c=colors[i % 7], label=tags[i]) ax.legend(loc='upper right') for j in range(len(df_similar['similar_tag'])): ax.plot(coords[j][0], coords[j][1], marker='') ax.annotate(df_similar['similar_tag'][j],(coords[j][0], coords[j][1])) plt.show()
#複数単語の類似度可視化
グラフ表示draw_scatter_plot(vectors, tags, cluster)
**fastTextでの表示**
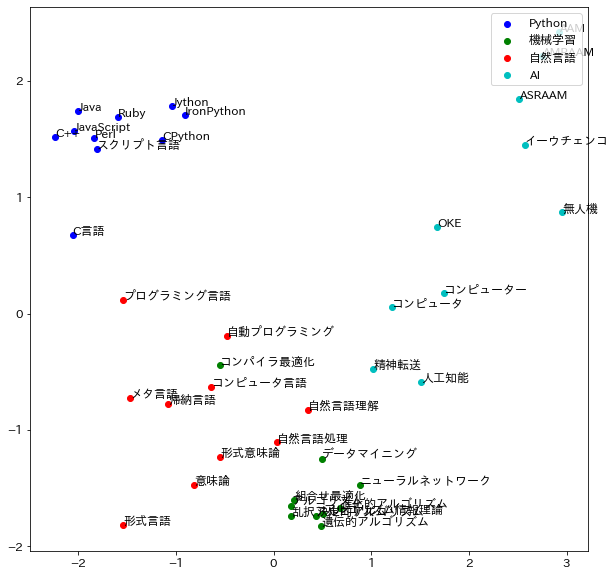#最後に
類似語を二次元グラフに表現でき、素直にうれしいです。
word2vecとfastTextは、異なる学習曲線済データを利用していますので、類似語にも分布にも違いが出ました。
いろんな見方で気づきが得られ、いいですね。この記事はここまでです。最後まで見ていただきありがとうございました。
#参考サイト
https://www.suzu6.net/posts/281-fasttext-in-colabolatory/http://cedro3.com/ai/word2vec-gensim/