Plotly Dashとは
Plotly Dash(正式名称はDashらしいですが、他にもDashという名前のアプリが色々あるのでこの表記とします)はPython製のWebアプリケーションのフレームワークです。
FlaskやBottleなど、Python製のWebフレームワークは色々ありますが、Plotly DashはPlotlyを使用した可視化されたデータを組み込むことができます。
インストール
pipでインストールします。condaでインストールできるかどうかは確認していません。
pip install dash==0.17.7 # The core dash backend
pip install dash-renderer==0.7.4 # The dash front-end
pip install dash-html-components==0.7.0 # HTML components
pip install dash-core-components==0.11.0 # Supercharged components
pip install plotly==2.0.12 # Plotly graphing library used in examples
とりあえずかいてみる
import dash
import dash_core_components as dcc
import dash_html_components as html
import numpy as np
x = np.linspace(-np.pi, np.pi, 10)
y = np.sin(x)
app = dash.Dash()
app.layout = html.Div(children=[
html.H1(children='H1の文章'),
html.Div(children='''
divの文章
'''),
dcc.Graph(
id='example-graph',
figure={
'data': [
{'x': x, 'y': np.sin(x), 'type': 'line', 'name': 'line'},
{'x': x, 'y': np.cos(x), 'type': 'bar', 'name': 'bar'},
],
'layout': {
'title': 'グラフのタイトル'
}
}
)
])
if __name__ == '__main__':
app.run_server(debug=True)
上記のようにHTMLを書く必要はなく、Pythonのコードだけで完結できます。
グラフの描画はPlotlyが担っています。
Markdown
Markdown記法に対応しています。
import dash
import dash_html_components as html
import dash_core_components as dcc
markdown_doc = dcc.Markdown('''
# 見出し1
## 見出し2
### 見出し3
---
* 箇条書き
* 箇条書き
---
[りんく](http://commonmark.org/help)
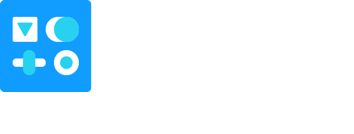
> 引用
''')
app = dash.Dash()
app.layout = html.Div(markdown_doc)
if __name__ == '__main__':
app.run_server(debug=True)
筆者の環境ではtableが表現できませんでした。もしうまくいった方がいらっしゃいましたら教えてください。
Live Updates
動的なグラフにも対応しています。
下記の例では psutil
を使用してマシンのリソース状況をリアルタイムにモニタします。
import dash
from dash.dependencies import Input, Output, Event
import dash_core_components as dcc
import dash_html_components as html
import datetime
import plotly
import numpy as np
import pandas as pd
import psutil
colors = {
'background': '#111111',
'text': '#7FDBFF'
}
app = dash.Dash(__name__)
app.layout = html.Div(children=[
html.Div([
html.H4('オレオレシステムモニタ'),
html.Div(id='live-update-text'),
dcc.Graph(id='live-update-graph'),
html.H4('プロセスリスト'),
html.Div(id='live-update-proc'),
dcc.Interval(
id='interval-component',
interval=1 * 1000 # in milliseconds
)
])
],
style={'backgroundColor': colors['background'], 'color': colors['text']}
)
class Context:
def __init__(self):
self.t = []
self.cpu = []
self.per_cpu = [[] for x in range(psutil.cpu_count())]
self.mem = []
@classmethod
def append_data(cls, d1, d2):
n = len(d1)
if n > 100:
del d1[0:n - 99]
d1.append(d2)
context = Context()
# The `dcc.Interval` component emits an event called "interval"
# every `interval` number of milliseconds.
# Subscribe to this event with the `events` argument of `app.callback`
@app.callback(Output('live-update-text', 'children'),
events=[Event('interval-component', 'interval')])
def update_metrics():
now = datetime.datetime.now()
hour, minute, second = now.hour, now.minute, now.second
style = {'padding': '5px', 'fontSize': '16px'}
return [
html.Span('CPU: {}%'.format(context.cpu[-1]), style=style),
html.Span('Memory: {}%'.format(context.mem[-1]), style=style)
]
# Multiple components can update everytime interval gets fired.
@app.callback(Output('live-update-graph', 'figure'),
events=[Event('interval-component', 'interval')])
def update_graph_live():
# global context
context.append_data(context.t, datetime.datetime.now())
context.append_data(context.cpu, psutil.cpu_percent())
for data, pct in zip(context.per_cpu, psutil.cpu_percent(percpu=True)):
context.append_data(data, pct)
context.append_data(context.mem, psutil.virtual_memory().percent)
# Create the graph with subplots
fig = plotly.tools.make_subplots(rows=2, cols=1, vertical_spacing=0.2)
fig['layout']['margin'] = {
'l': 30, 'r': 10, 'b': 30, 't': 10
}
fig['layout']['plot_bgcolor'] = colors['background']
fig['layout']['paper_bgcolor'] = colors['background']
fig['layout']['font'] = {'color': colors['text']}
fig['layout']['legend'] = {'x': 0, 'y': 1, 'xanchor': 'left'}
fig['layout']['yaxis1'].update(range=[0, 100])
fig['layout']['yaxis2'].update(range=[0, 100])
fig.append_trace({
'x': context.t,
'y': context.cpu,
'name': 'cpu',
'mode': 'lines',
'type': 'scatter',
}, 1, 1)
for i, y in enumerate(context.per_cpu):
fig.append_trace({
'x': context.t,
'y': y,
'name': 'cpu {}'.format(i),
'mode': 'lines',
'type': 'scatter',
}, 1, 1)
fig.append_trace({
'x': context.t,
'y': context.mem,
'name': 'memory',
'mode': 'lines',
'type': 'scatter',
'fill': 'tonexty',
}, 2, 1)
return fig
def get_proc_df():
def get_proc(proc):
try:
pinfo = proc
except psutil.NoSuchProcess:
pass
return (pinfo.pid, pinfo.name(), pinfo.memory_percent(), pinfo.cpu_percent())
data = [get_proc(proc) for proc in psutil.process_iter()]
df = pd.DataFrame(data, columns=['pid', 'name', 'memory', 'cpu'])
df['memory'] = df['memory'].map(lambda x: '{:.2f}%'.format(x))
df['cpu'] = df['cpu'] / psutil.cpu_count()
df['cpu'] = df['cpu'].map(lambda x: '{:.2f}%'.format(x))
return df.sort_values('cpu', ascending=False)
@app.callback(Output('live-update-proc', 'children'),
events=[Event('interval-component', 'interval')])
def generate_table():
df = get_proc_df()
max_rows = 10
return html.Table(
# Header
[html.Tr([html.Th(col) for col in df.columns])] +
# Body
[html.Tr([
html.Td(df.iloc[i][col], style={'width': '8em'}) for col in df.columns
]) for i in range(min(len(df), max_rows))]
)
if __name__ == '__main__':
app.run_server(debug=True)
対話的な操作
UIを操作しながら対話的に出力内容をコントロールすることができます。
やり方はそのうち書きたいと思います。