はじめに
この記事では私が作成した文書要約のWebアプリの使用方法とコードを解説しています。
コードはGitHubにリポジトリを作成しておりますので、使用されたい方はCloneしてお使いください。
https://github.com/YusukeOhnishi/document_summarize
このWebアプリは文書(英語論文)を読む際に、
- さっとまとめてくれるWebアプリがあれば便利そう
- 自身で必要な機能を追加していけたら楽しそう
というモチベーションから作成しました。実際に使用してみて、不便だなと感じる点やこういう機能が欲しいなと思えば、コメント及び連絡いただけると助かります。できる限り機能追加して対応していきます。
Webアプリの使用方法
Webアプリの起動
作成したアプリケーションはローカル環境及びDockerコンテナ上で起動することができます。
以下のコマンドを実行してクローンを行ってください。
git clone https://github.com/YusukeOhnishi/document_summarize.git
cd ./document_summarize
このアプリを使用するためにはOpenAI API Keyが必要です。
そのため、以下のOpenAIの公式ページから、自身のOpenAI API Keyを取得してください。
https://platform.openai.com/api-keys

Docker
Dockerにて起動する場合、まずはDockerfile内にOpenAI API Keyを書き込みます。
Dockerfileの以下の箇所にOpenAI API Keyを書き込みファイルを保存してください。
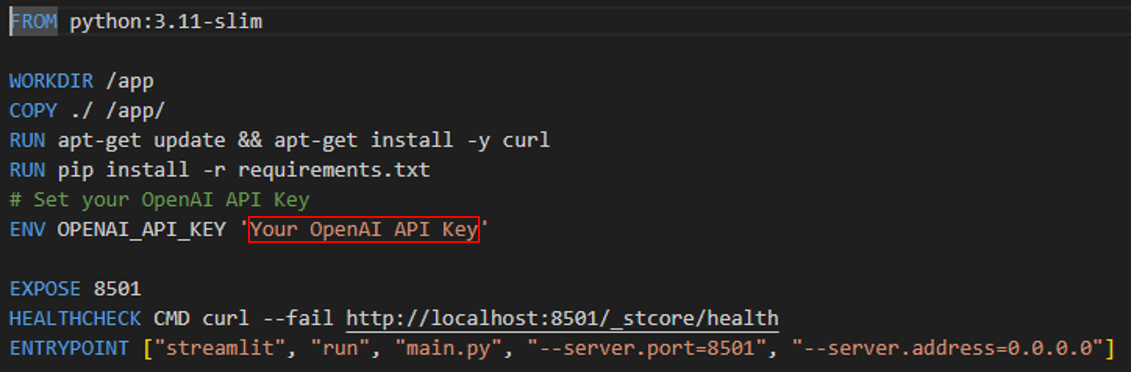
Dockerfileの修正後にDockerコンテナをbuild・runするために以下のコマンドを実行してください。
docker build -t document_summarize .
docker run -p 8501:8501 document_summarize
コンテナの起動が完了した後、 ブラウザでhttp:localhost:8501
にアクセスすることでWebアプリの画面に遷移することができます。
Local
ローカル環境にてアプリを起動する場合、必要であれば以下のコマンドを実行して仮想環境を構築して下さい。
python venv venv
source ./source venv/bin/activate
以下のコマンドを実行して、環境変数(OpenAI API Key)と必要なライブラリのインストールを行いstreamlitを起動してください。
export OPENAI_API_KEY='Your OpenAI API Key'
pip install -r requirement.txt
streamlit run main.py
起動後にコマンドライン上に出力されているURLにアクセスすることで、ローカル上にて起動しているアプリの画面に遷移することができます。
アプリの使い方
概要
このアプリでは出力言語と用いるgptモデルを指定して、指定したファイルの要約結果を出力できます。
出力を行う前にインプットの文章にかかるOpenAI APIの費用とトークン数を確認することができます。また、要約結果出力時に、アウトプットの文章にかかるOpenAI APIの費用とトークン数を確認することができます。
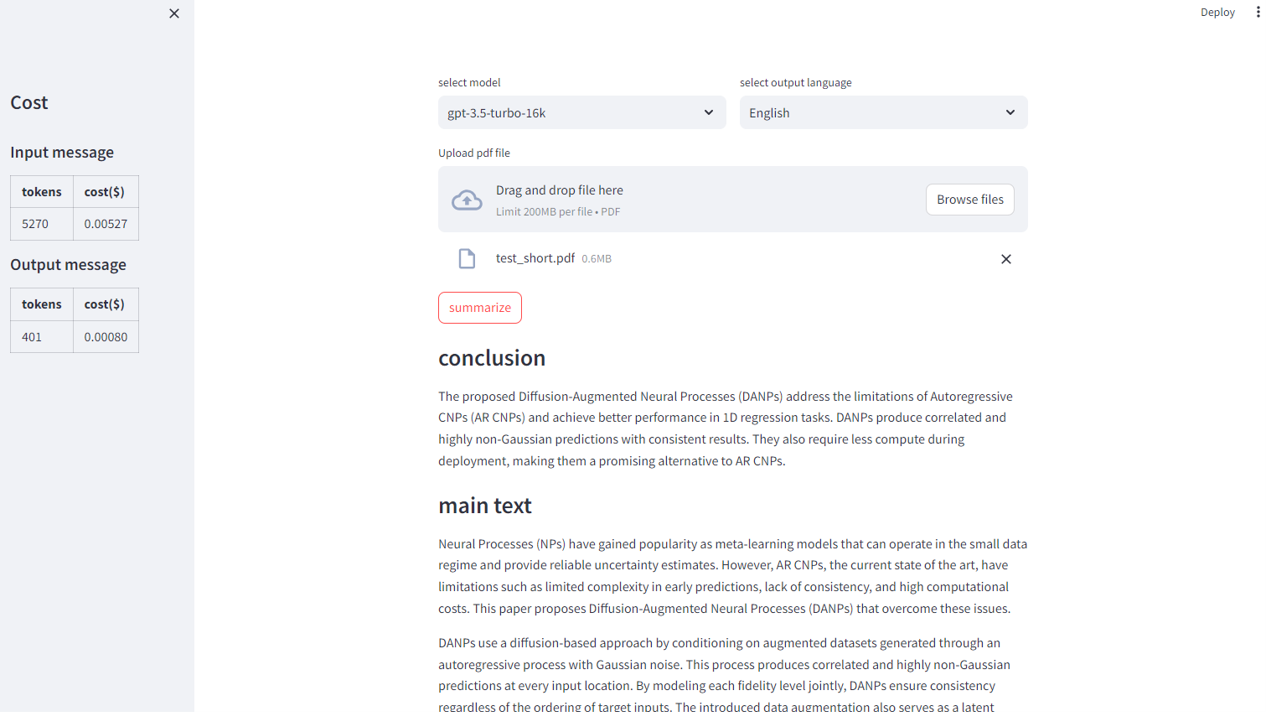
gptモデルの設定
gpt modelを設定します(デフォルトはgpt-3.5-turbo-16k)。
現在以下のモデルが使用できるようにしています。
- gpt-3.5-turbo-16k
- gpt-3.5-turbo
- gpt-4
- gpt-4-32k
- gpt-4-1106-preview

出力言語の設定
最終結果の出力言語を指定します。
現在以下の言語を指定することができます。
- English
- Japanese

ファイルアップロード
ファイルをアップロードします。ファイルはドラッグ&ドロップにてアップロードいただくか、エクスプローラーから直接指定することができます。
現在対応しているファイル形式は以下の通りです。

ファイルのアップロードが完了すると、サイドバーに入力文章にかかるトークン数と費用が表示されます。

要約の実行
summaryボタンを押すことで要約処理が実行されます。

要約処理が完了すると、要約結果が出力されます。また併せてサイドバーに出力文章にかかるトークン数と費用が表示されます。

コードの解説
メインのコードは以下のような流れで処理を行っています。
- モデル情報・言語情報・ファイル情報の取得
- gptモデル定義
- ファイル情報から文章の取得とトークン数とAPI利用料を取得
- gptモデルの最大トークン数に応じて文章を分割
- 文書の要約処理
- 要約結果のトークン数とAPI利用料を取得
import streamlit as st
import os
from src.load import load_pdf
from src.create_prompt import (
create_summary_prompt, create_part_summary_prompt)
from src.chat_model import chat_model
from src.utils import (clean_text, split_text)
from data.model_info import get_model_info
from data.selection import (get_model_list, get_language_list)
api_key = os.getenv('OPENAI_API_KEY')
st.sidebar.write('# Cost')
### 1.モデル情報・言語情報・ファイル情報の取得 ###
col1, col2 = st.columns((1, 1))
with col1:
model = st.selectbox(
'select model',
get_model_list())
with col2:
language = st.selectbox(
'select output language',
get_language_list())
pdf_file = st.file_uploader("Upload pdf file", type='pdf')
### 2.gptモデル定義 ###
try:
model_info = get_model_info(model)
max_word = int(model_info["max_token"]/3)
chat_model = chat_model(api_key, model_info["model_name"])
### 3.ファイル情報から文章の取得とトークン数とAPI利用料を取得 ###
text = load_pdf(pdf_file)
text = clean_text(text)
input_message_token_num = chat_model.get_message_token_num(text)
input_message_price = input_message_token_num * \
model_info["input_price"]/1000
input_message_price = '{:.5f}'.format(input_message_price)
except:
st.stop()
### 4.gptモデルの最大トークン数に応じて文章を分割 ###
text_list = split_text(text, max_word)
st.sidebar.write(f'''
## Input message
|tokens|cost($)|
|---|---|
|{input_message_token_num}|{input_message_price}|
''')
### 5.文書の要約処理 ###
if st.button('summarize'):
message = ""
if len(text_list) <= 1:
message = text_list[0]
else:
for text in text_list:
result_tmp = chat_model.get_chat_message(
create_part_summary_prompt(text))
message += result_tmp.choices[0].message.content
result = chat_model.get_chat_message(
create_summary_prompt(message, language))
result = result.choices[0].message.content
st.write(result)
### 6.要約結果のトークン数とAPI利用料を取得 ###
output_message_token_num = chat_model.get_message_token_num(result)
output_message_price = output_message_token_num * \
model_info["output_price"]/1000
output_message_price = '{:.5f}'.format(output_message_price)
st.sidebar.write(f'''
## Output message
|tokens|cost($)|
|---|---|
|{output_message_token_num}|{output_message_price}|
''')
1. モデル情報・言語情報・ファイル情報の取得
### 1.モデル情報・言語情報・ファイル情報の取得 ###
col1, col2 = st.columns((1, 1))
with col1:
model = st.selectbox(
'select model',
get_model_list())
with col2:
language = st.selectbox(
'select output language',
get_language_list())
pdf_file = st.file_uploader("Upload pdf file", type='pdf')
この箇所ではstreamlitのselectboxの機能を用いて、モデルと言語の選択を行わせています。どのモデル・言語を表示させるかは、別ファイルにリストを作成し、これを取得することで選択肢の文字列を指定しています。
def get_model_list():
return ('gpt-3.5-turbo-16k', 'gpt-3.5-turbo', 'gpt-4-1106-preview', 'gpt-4-32k', 'gpt-4')
def get_language_list():
return ('English', 'Japanese')
またその後、streamlitのfile_uploade機能を用いて、ファイルのアップローダーを表示させています。このアップローダーにはtypeでpdfを選択し、pdfファイルのみをアップロードできるように指定しています。
2. gptモデル定義
### 2.gptモデル定義 ###
try:
model_info = get_model_info(model)
max_word = int(model_info["max_token"]/3)
chat_model = chat_model(api_key, model_info["model_name"])
この箇所では、「1. モデル情報・言語情報・ファイル情報の取得」にて入力されたモデルの情報からモデルの詳細情報を取得しています。こちらも別ファイルに記載した情報を入力されたモデル名をキーとして呼び出すということをしています。取得する情報としては
- モデル名(API呼び出しに使用するモデルの名前)
- MAXのトークン数
- インプットの文章に1000トークンあたりにかかるAPIの費用
- アウトプットの文章に1000トークンあたりにかかるAPIの費用
- です。
def get_model_info(model_name):
model_list = {
'gpt-3.5-turbo-16k': {
'model_name': 'gpt-3.5-turbo-16k',
'max_token': 16385,
'input_price': 0.001,
'output_price': 0.002,
},
'gpt-3.5-turbo': {
'model_name': 'gpt-3.5-turbo',
'max_token': 4096,
'input_price': 0.001,
'output_price': 0.002,
},
'gpt-4': {
'model_name': 'gpt-4',
'max_token': 8192,
'input_price': 0.03,
'output_price': 0.06,
},
'gpt-4-32k': {
'model_name': 'gpt-4-32k',
'max_token': 32768,
'input_price': 0.03,
'output_price': 0.06,
},
'gpt-4-1106-preview': {
'model_name': 'gpt-4-1106-preview',
'max_token': 128000,
'input_price': 0.01,
'output_price': 0.03,
},
}
return model_list[model_name]
次にMaxの単語数を取得しています。これは後で説明しますが長文の場合、APIに文章を送信した際にトークン数上限に引っ掛かりエラーとなる場合があります。そのためある程度の単語数で文章を分断させる必要があり、この1分断の1区切りあたりの単語数を指定しています。(今回はMax Token数の1/3としている)
最後にAPI Keyとモデル名を指定してgptモデルを扱うためのインスタンスを生成しています。今後、モデルの情報が必要になる処理については、このインスタンスに対してメソッドを実行するという流れを取ります。
from openai import OpenAI
import tiktoken
class chat_model():
def __init__(self, openai_api_key, model_name):
self.client = OpenAI(api_key=openai_api_key)
self.model_name = model_name
def get_chat_message(self, message):
response = self.client.chat.completions.create(
model=self.model_name,
messages=[
{"role": "user", "content": message},
],
)
return response
def get_message_token_num(self, message):
encoding = tiktoken.encoding_for_model(self.model_name)
tokens = encoding.encode(message)
tokens_count = len(tokens)
return tokens_count
3. ファイル情報から文章の取得とトークン数とAPI利用料を取得
### 3.ファイル情報から文章の取得とトークン数とAPI利用料を取得 ###
text = load_pdf(pdf_file)
text = clean_text(text)
input_message_token_num = chat_model.get_message_token_num(text)
input_message_price = input_message_token_num * \
model_info["input_price"]/1000
input_message_price = '{:.5f}'.format(input_message_price)
except:
st.stop()
この箇所ではまずpdfファイルの情報をstring型として読み取っています。今回はpypdfというモジュールを用いて内容を読み取っています。処理としてはページ毎に文章を読み取り、読み取った文章を文字列に連結させるというシンプルなものです。
from pypdf import PdfReader
def load_pdf(file_path):
reader = PdfReader(file_path)
texts = ""
for page in reader.pages:
texts += page.extract_text()
return texts
ここで読み取った文章は不要な文字列(改行コード等)が含まれた状態になっています。そのため、次にこれらの不要な文字列を除去しています。これで文章の取得は完了です。
import re
def clean_text(text):
text = text.replace('-\n', '')
text = re.sub(r'\s+', ' ', text)
return text
次に、取得した文章のトークン数を取得しています。これは「2. gptモデル定義」で取得したインスタンスに対して文章を渡し、get_message_token_numを呼び出すことで取得しています。取得方法はtiktokenというライブラリを用いて文章をトークン分割し、この長さを返却するという方法を取っています。この際、gptモデル毎にトークン分割の仕方が異なっているため、gptモデル名を指定しています。
最後にインプット文章にかかるAPI費用についてはトークン数とgptモデル毎の1000トークンあたりの価格の情報を持っているので、これらを元に計算させ、小数第5位までで四捨五入しています。
4. gptモデルの最大トークン数に応じて文章を分割
### 4.gptモデルの最大トークン数に応じて文章を分割 ###
text_list = split_text(text, max_word)
st.sidebar.write(f'''
## Input message
|tokens|cost($)|
|---|---|
|{input_message_token_num}|{input_message_price}|
''')
この箇所では「3. ファイル情報から文章の取得とトークン数とAPI利用料を取得」で取得した文章を「2. gptモデル定義」で求めた最大単語数(max_word)毎に分割し、分割した文字列の塊を要素としたリストを作成しています。
def split_text(text, wc_max):
words = text.split()
chunks = [' '.join(words[i:i + wc_max])
for i in range(0, len(words), wc_max)]
return chunks
5. 文書の要約処理
### 5.文書の要約処理 ###
if st.button('summarize'):
message = ""
if len(text_list) <= 1:
message = text_list[0]
else:
for text in text_list:
result_tmp = chat_model.get_chat_message(
create_part_summary_prompt(text))
message += result_tmp.choices[0].message.content
result = chat_model.get_chat_message(
create_summary_prompt(message, language))
result = result.choices[0].message.content
st.write(result)
この箇所では文章の要約を行わせていますが、「4. gptモデルの最大トークン数に応じて文章を分割」にて分割した文章の長さに1の場合とそれ以外の場合で処理を分けています。
分割文章が1つの場合
分割文章が1つの場合、つまり読み取った文章のトークン数がgptモデルのMaxトークン数以下の場合はそのままgptモデルにリストの要素をそのまま取得しています。この文章をそのままチャットに流すだけでは文章の要約は行われないため、プロンプトテンプレートを作成しておき、この中に文章を差し込んでgptモデルに文章を生成しています。プロンプトテンプレートの内容としては文章を制約条件の下で要約するように指定しています。またこの際に最初に取得した言語を挿入することで、最終的な出力結果の言語を何にするかを決定しています。
def create_summary_prompt(text, language):
return f"""
you are a scientist Please output a paper summary based on the following constraints and the input text.
%Constraints:
- Text is concise and easy to understand.
- Don't miss out on important keywords.
- Answer in two sections: conclusion and main text.
- The conclusion is about 150 characters.
- The main text is about 500 characters.
- The output format should follow the markdown format below.
- Please output the results in {language}.
%Output format
### conclusion
(Output the conclusion here)
### main text
(Output main text here)
##########################################
{text}
"""
こうして生成した文章をchat gptの会話のメッセージとして送信し、結果を取得しています。
class chat_model():
def __init__(self, openai_api_key, model_name):
self.client = OpenAI(api_key=openai_api_key)
self.model_name = model_name
def get_chat_message(self, message):
response = self.client.chat.completions.create(
model=self.model_name,
messages=[
{"role": "user", "content": message},
],
)
return response
分割文章が2つ以上の場合
分割文章が2つ以上の場合、つまり読み取った文章のトークン数がgptモデルのMaxトークン数以上の場合は文章の量を抑える必要があります。
今回作成したアプリでは分割した文章それぞれに対して、一度要約処理を行いこれら、要約された文章を再度結合させることで最終的にチャットとして投げる文章を作成しています。
分割した文章を要約させるプロンプトは別途用意しており、以下のテンプレートに分割した文章を挿入しています。こうして得た分割された文章を要約した結果を結合して、一つの短い文章を作成し、チャットへの送信を行います。以降の流れは「分割文章が1つの場合」と同様です。
def create_part_summary_prompt(text):
return f"""
you are a scientist Please output a paper summary based on the following constraints and the input text.
%%Constraints:
- Text is concise and easy to understand.
- Don't miss out on important keywords.
- Make it into one paragraph.
- Summarize within 400 words.
##########################################
{text}
"""
6. 要約結果のトークン数とAPI利用料を取得
この箇所ではアウトプットに関するトークン数とAPI費用を取得しています。
実施していることは「3. ファイル情報から文章の取得とトークン数とAPI利用料を取得」と同様のため説明は割愛します。
### 6.要約結果のトークン数とAPI利用料を取得 ###
output_message_token_num = chat_model.get_message_token_num(result)
output_message_price = output_message_token_num * \
model_info["output_price"]/1000
output_message_price = '{:.5f}'.format(output_message_price)
st.sidebar.write(f'''
## Output message
|tokens|cost($)|
|---|---|
|{output_message_token_num}|{output_message_price}|
''')
最後に
今回は文書要約アプリを作成しました。
今後もコードにアップデートを入れていく予定です。適宜こちらの記事も更新する予定ですが、記事の更新が遅れる可能性があるため、最新盤のコードを見たい場合はGitHubを確認してください。
https://github.com/YusukeOhnishi/document_summarize