はじめに
ウェブサービスのユーザがどのページからどのページに移っていっているのかとか、どの工程からどの工程に仕掛品が流れていったのかなどをPythonで可視化したい。
ここでは仮に以下のようなどのページからどのページに移っていったのかについて集計したデータがあったとする。
|
from |
to |
count |
0 |
A |
A |
111 |
1 |
A |
B |
1120 |
2 |
A |
C |
1230 |
3 |
B |
A |
214 |
4 |
B |
B |
1225 |
5 |
B |
C |
1216 |
6 |
C |
A |
327 |
7 |
C |
C |
2319 |
こんな感じでページ→ページの遷移数と遷移確率行列的なものを可視化したい。
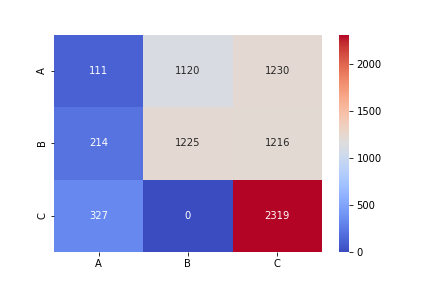

実装
以下でpandas, seaborn, matplotlibを使ってJupyter上で可視化する。
import pandas as pd
import matplotlib.pyplot as plt
import seaborn as sns
df = pd.DataFrame({'from': ['A', 'A', 'A', 'B', 'B', 'B', 'C', 'C'],
'to': ['A', 'B', 'C', 'A', 'B', 'C', 'A', 'C'],
'count': [111, 1120, 1230, 214, 1225, 1216, 327, 2319]})
df # C→Bのページ遷移は0件とする
|
from |
to |
count |
0 |
A |
A |
111 |
1 |
A |
B |
1120 |
2 |
A |
C |
1230 |
3 |
B |
A |
214 |
4 |
B |
B |
1225 |
5 |
B |
C |
1216 |
6 |
C |
A |
327 |
7 |
C |
C |
2319 |
pages = sorted(list(set(df['from'].values) | set(df['to'].values)))
pages
count_matrix = pd.DataFrame(index=pages, columns=pages, dtype=float)
count_matrix
|
A |
B |
C |
A |
NaN |
NaN |
NaN |
B |
NaN |
NaN |
NaN |
C |
NaN |
NaN |
NaN |
for i in zip(df['from'].values, df['to'].values, df['count'].values):
count_matrix.at[i[0], i[1]] = i[2]
count_matrix.fillna(0, inplace=True)
row_sum = count_matrix.sum(axis=1)
row_sum
A 2461.0
B 2655.0
C 2646.0
dtype: float64
probabilistic_matrix = count_matrix.div(row_sum, axis=0)
probabilistic_matrix
|
A |
B |
C |
A |
0.045104 |
0.455100 |
0.499797 |
B |
0.080603 |
0.461394 |
0.458004 |
C |
0.123583 |
0.000000 |
0.876417 |
sns.heatmap(count_matrix, annot=True, cmap='coolwarm', fmt='g', vmin=0)
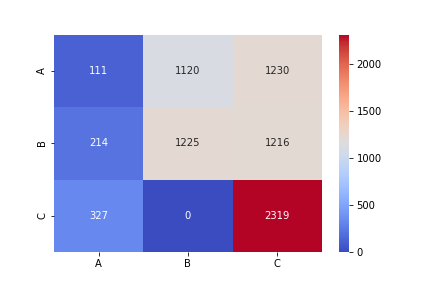
sns.heatmap(probabilistic_matrix, annot=True, cmap='coolwarm', fmt='.3f', vmin=0, vmax=1)
plt.ylabel('from')
plt.xlabel('to')
plt.show()
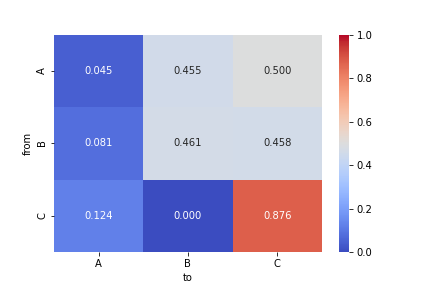