問題の確認
インボリュート関数
$f(\alpha) = tan\ \alpha - \alpha$
の逆関数 $f^{-1}$を求めます。

詳しい内容は導入編で確認してください。
ある関数の逆関数を数値的に作るいろいろな方法 導入編 - Qiita
逆関数を表す方法
最初に思いついた方法は多項式による近似でした。
求めたい逆関数は単調増加関数だし、多項式でもそこそこの精度で近似できるんじゃないかと考えました。
つまり、逆関数を
$ y = a_0 + a_1x + a_2x^{2} + ...$
というように表すことで、近い値を得られるだろうという目論見です。
多項式の求め方
問題となるのは多項式の係数 $a_0, a_1, a_2, ...$を決定する方法です。多項式で得られる$y$の値と真の値との差が最小になるように係数を決める必要があります。
scikit-learnは機械学習用のライブラリですが、これを使えば多項式の係数を自動的に決めることができることがわかりました。
訓練データの生成
インボリュート関数を使って学習のための訓練データを生成します。
def involute(α):
return np.tan(α) - α
y = np.linspace(- np.pi / 4, np.pi / 4, 1000)
x = involute(y)
機械学習の慣例に合わせて入力($inv\alpha$)を$x$、出力($\alpha$)を$y$としています。$y$($\alpha$)の値を先に用意して、インボリュート関数を使って$x$($inv\alpha$)の値を求めて、それを訓練データとします。
scikit-learnを使った多項式のフィット
scikit-learnを使うと多項式のフィットを簡単に行うことができます。
まずは必要なライブラリをインポートします。
from sklearn.preprocessing import PolynomialFeatures
from sklearn.linear_model import LinearRegression
from sklearn.pipeline import make_pipeline
上で生成した訓練データは1次元配列ですが、scikit-learnではデータは列ベクトルが基本なので列ベクトルに変換します。
x_column = x.reshape(-1, 1)
y_column = y.reshape(-1, 1)
まずは10次多項式をフィットさせてみます。
model_poly = make_pipeline(PolynomialFeatures(degree=10), LinearRegression())
model_poly.fit(x_column, y_column)
model_poly.score(x_column, y_column)
0.95581676585298314
PolynomialFeatures(degree=10)
は10次多項式への変換、LinearRegression()
は線形回帰モデルの生成で、それらをmake_pipeline関数で組み合わせて多項式回帰モデルを作っています。
作成したモデルのfitメソッドに学習データを与えて、多項式のフィットを行います。
scoreメソッドはどの程度の推定精度があるかを数値で評価します。この値が1.0なら完璧に推定できているということになります。
多項式回帰モデルによる推定値のプロット
では、多項式回帰モデルで推定した値をプロットしてみましょう。
y_pred = model_poly.predict(x_column).flatten()
fig = figure(width=400, height=400)
fig.scatter(x, np.degrees(y), size=1, legend='真値')
fig.line(x, np.degrees(y_pred), line_color='orange', legend='推定値')
fig.xaxis.axis_label = 'invα'
fig.yaxis.axis_label = '圧力角α (deg)'
fig.legend.location = 'top_left'
show(fig)

predictメソッドに入力値を与えて推定値を求めています。
得られた推定値をプロットした結果が上のグラフです。
推定精度が悪すぎますね。
次数を20次に増やしてみるとどうでしょうか。
model_poly = make_pipeline(PolynomialFeatures(degree=20), LinearRegression())
model_poly.fit(x_column, y_column)
model_poly.score(x_column, y_column)
0.97492606041826035
y_pred = model_poly.predict(x_column).flatten()
fig = figure(width=400, height=400)
fig.scatter(x, np.degrees(y), size=1, legend='真値')
fig.line(x, np.degrees(y_pred), line_color='orange', legend='推定値')
fig.xaxis.axis_label = 'invα'
fig.yaxis.axis_label = '圧力角α (deg)'
fig.legend.location = 'top_left'
show(fig)
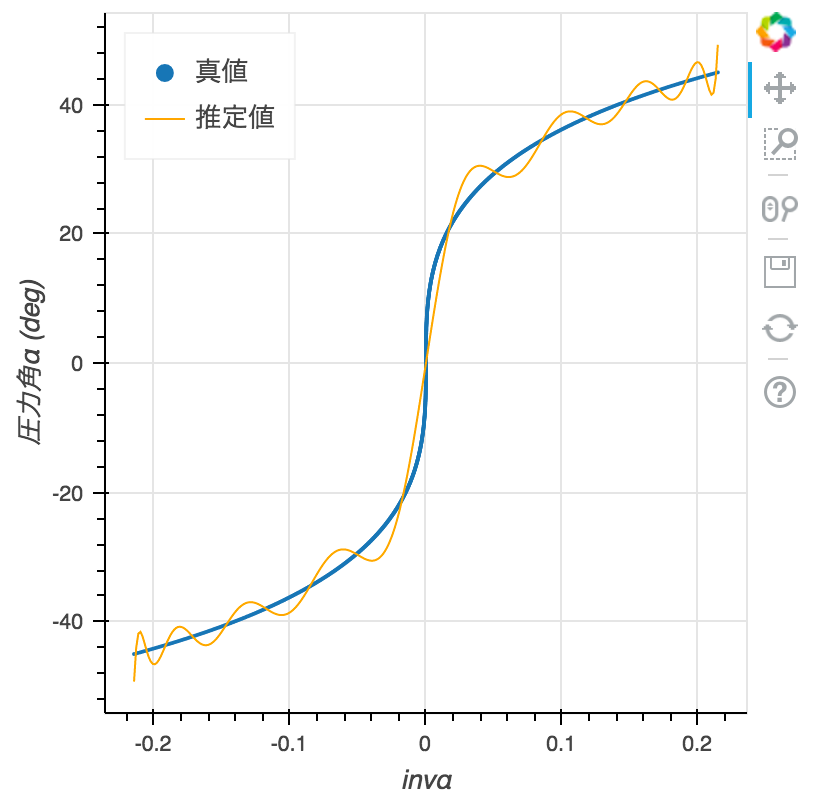
20次に増やしても変動の波が小刻みになるだけで、あまり改善はされていません。
このまま次数を増やしても良くなりそうにはありません。
多項式回帰ではうまく推定できない理由
原点付近の勾配が大きいことが多項式回帰でうまく推定できない原因だと思います。
インボリュート逆関数は原点で勾配が無限大になりますが、多項式を使っている限り絶対に無限大の勾配は表現できません。
ソースコード
説明に使ったNotebookはGistにアップロードしています。
インボリュート逆関数の推定_多項式回帰.ipynb