概要
NanoPi-NEOもしくはNanoPi-NEO2に繋いだUSBカメラから、
OpenCV経由でカメラキャプチャして、リサイズして、グレースケール化して、
リニア空間での誤差拡散法で2値化して、NanoHat OLED (= SSD1306) に I2C使って表示する。以上、それだけ。
それだけなんだけど、リニア空間での誤差拡散法適用が、地味に論文1本書けるかもしれない(笑
ソースコード
全体はこちら。
https://github.com/blue777/NanoPi-NEO
### メイン部分
いたって普通の、なんの取り留めも、なんの変哲も無い、
普通のOpenCVコード。
#include "common/i2c_oled_ssd1306.h"
#include "common/img_halftone.h"
#include "common/perf_log.h"
#include <opencv2/opencv.hpp>
int main()
{
I2C_OLED_SSD1306 oled;
cv::VideoCapture cap(0);
if(!cap.isOpened())
{
printf("No capture devices.\n");
return -1;
}
cap.set( CV_CAP_PROP_FRAME_WIDTH, 640 );
cap.set( CV_CAP_PROP_FRAME_HEIGHT, 360 );
cap.set( CV_CAP_PROP_FPS, 30 );
oled.Init();
oled.DisplayOn();
while( 1 )
{
cv::Mat src,dst;
cap >> src;
cv::resize( src, src, cv::Size(128,64), 0, 0, cv::INTER_LANCZOS4 );
cv::cvtColor( src, dst, CV_BGR2GRAY);
{
// PerfLog iPerf("dither");
// ImageHalftoning::ErrDiff_LinearFloydSteinberg( dst.data, dst.step, dst.cols, dst.rows );
ImageHalftoning::ErrDiff_LinearStucki( dst.data, dst.step, dst.cols, dst.rows );
// ImageHalftoning::ErrDiff_FloydSteinberg( dst.data, dst.step, dst.cols, dst.rows );
// ImageHalftoning::ErrDiff_Burkes( dst.data, dst.step, dst.cols, dst.rows );
// ImageHalftoning::ErrDiff_Stucki( dst.data, dst.step, dst.cols, dst.rows );
// ImageHalftoning::ErrDiff_Atkinson( dst.data, dst.step, dst.cols, dst.rows );
// ImageHalftoning::PatternDither_2x2( dst.data, dst.step, dst.cols, dst.rows );
}
// cv::imwrite("src.png",src);
// cv::imwrite("dst.png",dst);
// PerfLog iPerf("disp");
oled.WriteImage(dst.data, dst.step);
}
return 0;
}
誤差拡散法による2値化
とりあえず、4種類実装してみた。うち2種類はリニア空間対応である。
ソースは「img_halftone.h」である。
コンパイルを簡単にするため、ヘッダ実装である。
それぞれの誤差拡散先の係数。
FloydSteinberg
- | - | * | 7/16 | - |
---|---|---|---|---|
- | 3/16 | 5/16 | 1/16 | - |
Burkes
- | - | * | 4/16 | 2/16 |
---|---|---|---|---|
1/16 | 2/16 | 4/16 | 2/16 | 1/16 |
Stucki
- | - | * | 8/42 | 4/42 |
---|---|---|---|---|
2/42 | 4/42 | 8/42 | 4/42 | 2/42 |
1/42 | 2/42 | 4/42 | 2/42 | 1/42 |
Atkinson
- | - | * | 1/8 | 1/8 |
---|---|---|---|---|
- | 1/8 | 1/8 | 1/8 | - |
- | - | 1/8 | - | - |
いずれのアルゴリズムも、基本的にガンマ値を考慮したものではないので、そのまま実装すると、画像が明るくでてしまう。
そのため、Gamma=2.2でリニア空間に戻してから誤差拡散法実施すると、ものすごく知覚的に一致し、なぜか綺麗に見えてくる。
左から順に、「グレースケール」「リニア空間で誤差拡散」「誤差拡散」です。
※右側画像が明るく見えるのが期待値で、画像がピクセル等倍表示になっていないと、期待値がらハズレます。拡大・縮小された状態でみると、話がめんどくさくなりますので、必ずピクセル等倍で見てください。
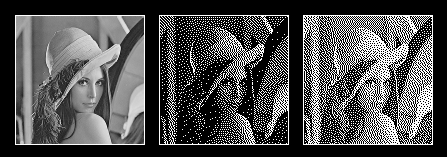
2値タイプのOLED表示を行うがこそ必要な技法である(笑
以下にコードの一部を載せる。(真ん中の画像生成に使用したもの)
void ErrDiff_LinearFloydSteinberg( unsigned char * image, int stride, int width, int height )
{
int shift = 20;
int gamma[256];
std::vector<int> data(width*height);
for( int i = 0; i < 256; i++ )
{
gamma[i] = (int)(pow( i / 255.0, 2.2 ) *((1 << shift) - 1));
}
for( int y = 0; y < height; y++ )
{
unsigned char* src_line = image + stride * y;
int * dst_line = &data.data()[ width * y ];
int x = 0;
for( ; (x+4) <= width; x += 4 )
{
dst_line[x+0] = gamma[ src_line[x+0] ];
dst_line[x+1] = gamma[ src_line[x+1] ];
dst_line[x+2] = gamma[ src_line[x+2] ];
dst_line[x+3] = gamma[ src_line[x+3] ];
}
for( ; x < width; x++ )
{
dst_line[x+0] = gamma[ src_line[x+0] ];
}
}
for( int y = 0; y < height; y++ )
{
int* line = &data.data()[ width * y ];
unsigned char* dst_line = image + stride * y;
for( int x = 0; x < width; x++ )
{
int c = line[x];
int e = (1 << (shift-1)) <= c ? c - ((1 << shift) - 1) : c;
dst_line[x] = (1 << (shift-1)) <= c ? 255 : 0;
// - * 7/16
// 3/16 5/16 1/16
if( (x+1) < width ) line[x+1] += e * 7 / 16;
if( (y+1) < height )
{
int* lineN = line + width;
if( 0 <= (x-1) ) lineN[x-1] += e * 3 / 16;
lineN[x ] += e * 5 / 16;
if( (x+2) < width ) lineN[x+1] += e * 1 / 16;
}
}
}
}
NanoPi-NEO(2)でCloud9から実行する場合
OLED表示はI2Cを直叩きしてますので、特に外部依存なく使えるハズです。
そのため、OpenCV入れて、Cloud9上でコンパイル&実行だけで通る(ハズ)。
NanoPi-NEO2へのCloud9のインストール手順は、
Cloud9 を NanoPi NEO2 にインストールするで。
NanoPi-NEO(2)にOpenCV入れる
パッケージ入れればOK
apt-get install libcv-dev libopencv-dev
Cloud9のRunnerを編集する
そのままだと、OpenCVが使えないのでRunnerを修正しライブラリ参照を追加します
// This file overrides the built-in C++ (simple) runner
// For more information see http://docs.c9.io:8080/#!/api/run-method-run
{
"script": [
"set -e",
"if [ \"$debug\" == true ]; then ",
"/usr/bin/g++ -ggdb3 -std=c++11 $file -o $file.o `pkg-config --cflags opencv` `pkg-config --libs opencv`",
"chmod 755 \"$file.o\"",
"node $HOME/.c9/bin/c9gdbshim.js \"$file.o\" $args",
"else",
"/usr/bin/g++ -std=c++11 $file -o $file.o -O3 `pkg-config --cflags opencv` `pkg-config --libs opencv`",
"chmod 755 $file.o",
"$file.o $args",
"fi"
],
"info": "Running (C++ OpenCV) $file",
"debugger": "gdb",
"$debugDefaultState": false,
"env": {},
"selector": "^.*\\.(cpp|cc)$"
}
あとは実行すればOK
### OLEDの表示速度について
I2Cの転送速度 100kHz or 400kHz に依存します。
テキトーに計算すると、
OLEDのサイズが128x64、1Pixel=1bit表現なので、
$ ImageDataSize = 128 * 64 / 8 = 1288 [byte] $
I2Cで送る場合は制御コマンド1byteがつくので、StartCode/EndCode無視して、
bit単位に変換すると
$ (1288+1)*8 = 10312 [bit] $
になるので、I2C駆動速度が100kHzの場合は、
$ 1000000 [bits/sec]/ 10312 [bits/frame] = 9.6974 [frame/sec] $
となる。
400kHzの場合は、4倍になるので40fps近くになることになる。
そして、困ったことに、
NEO2 の 4.x,y系カーネルは、100kHz駆動
NEO の 3.x.y系カーネルは、400kHz駆動
その他未調査である。